AdaMEC: Towards a Context-adaptive and Dynamically Combinable DNN Deployment Framework for Mobile Edge Computing
ACM TRANSACTIONS ON SENSOR NETWORKS(2024)
摘要
With the rapid development of deep learning, recent research on intelligent and interactive mobile applications (e.g., health monitoring, speech recognition) has attracted extensive attention. And these applications necessitate the mobile edge computing scheme, i.e., offloading partial computation from mobile devices to edge devices for inference acceleration and transmission load reduction. The current practices have relied on collaborative DNN partition and offloading to satisfy the predefined latency requirements, which is intractable to adapt to the dynamic deployment context at runtime. AdaMEC, a context-adaptive and dynamically combinable DNN deployment framework, is proposed to meet these requirements for mobile edge computing, which consists of three novel techniques. First, once-for-all DNN pre-partition divides DNN at the primitive operator level and stores partitioned modules into executable files, defined as pre-partitioned DNN atoms. Second, context-adaptive DNN atom combination and offloading introduces a graph-based decision algorithm to quickly search the suitable combination of atoms and adaptively make the offloading plan under dynamic deployment contexts. Third, runtime latency predictor provides timely latency feedback for DNN deployment considering both DNN configurations and dynamic contexts. Extensive experiments demonstrate that AdaMEC outperforms state-of-the-art baselines in terms of latency reduction by up to 62.14% and average memory saving by 55.21%.
更多查看译文
关键词
Context-adaptive,DNN combination and offloading,DNN partition,edge intelligence
AI 理解论文
溯源树
样例
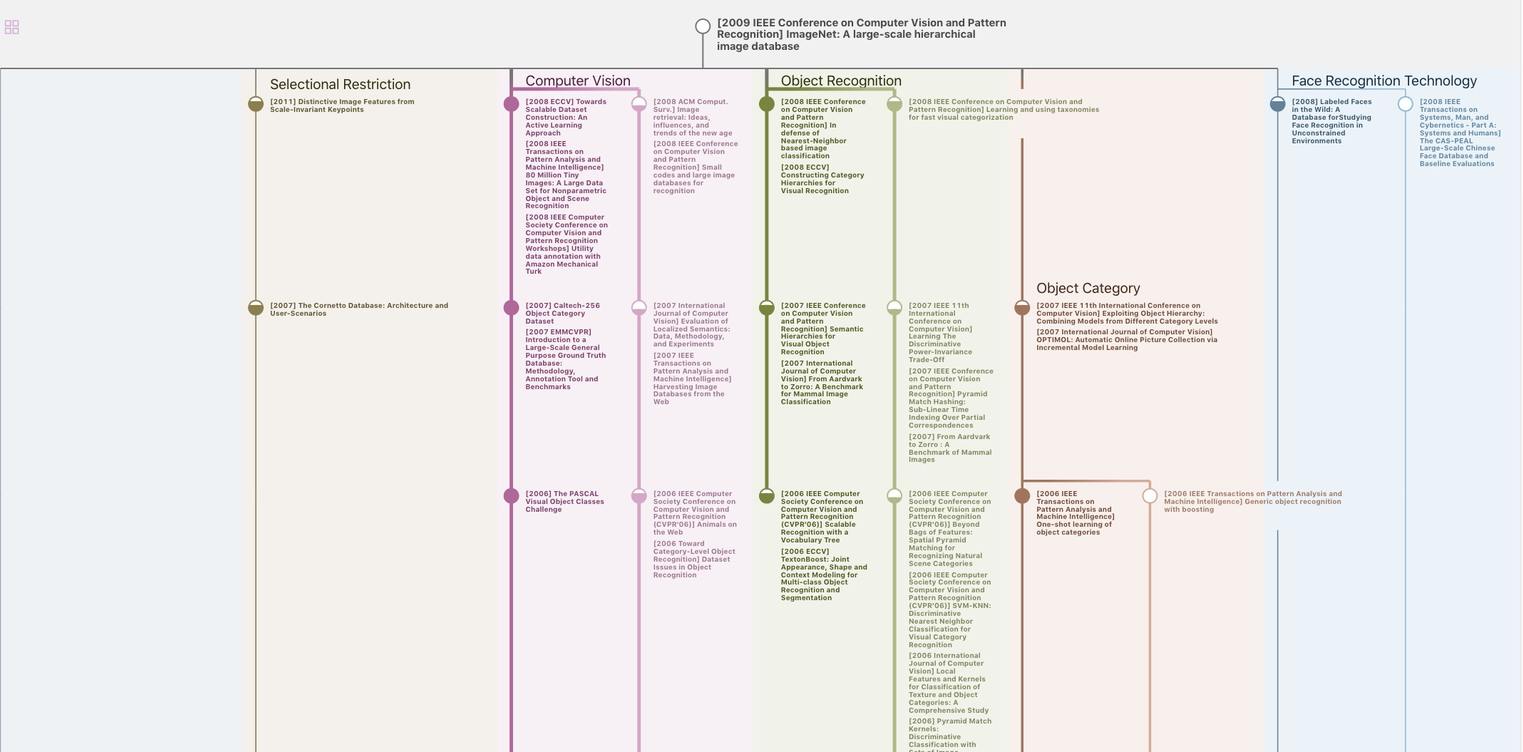
生成溯源树,研究论文发展脉络
Chat Paper
正在生成论文摘要