Video Referring Expression Comprehension via Transformer with Content-conditioned Query
PROCEEDINGS OF THE 1ST INTERNATIONAL WORKSHOP ON DEEP MULTIMODAL LEARNING FOR INFORMATION RETRIEVAL, MMIR 2023(2023)
摘要
Video Referring Expression Comprehension (REC) aims to localize a target object in videos based on the queried natural language. Recent improvements in video REC have been made using Transformer-based methods with learnable queries. However, we contend that this naive query design is not ideal given the open-world nature of video REC brought by text supervision. With numerous potential semantic categories, relying on only a few slow-updated queries is insufficient to characterize them. Our solution to this problem is to create dynamic queries that are conditioned on both the input video and language to model the diverse objects referred to. Specifically, we place a fixed number of learnable bounding boxes throughout the frame and use corresponding region features to provide prior information. Also, we noticed that current query features overlook the importance of cross-modal alignment. To address this, we align specific phrases in the sentence with semantically relevant visual areas, annotating them in existing video datasets (VID-Sentence and VidSTG). By incorporating these two designs, our proposed model (called ConFormer) outperforms other models on widely benchmarked datasets. For example, in the testing split of VID-Sentence dataset, ConFormer achieves 8.75% absolute improvement on Accu.@0.6 compared to the previous state-of-the-art model.
更多查看译文
关键词
Referring Expression Comprehension,Transformer,Dynamic Query
AI 理解论文
溯源树
样例
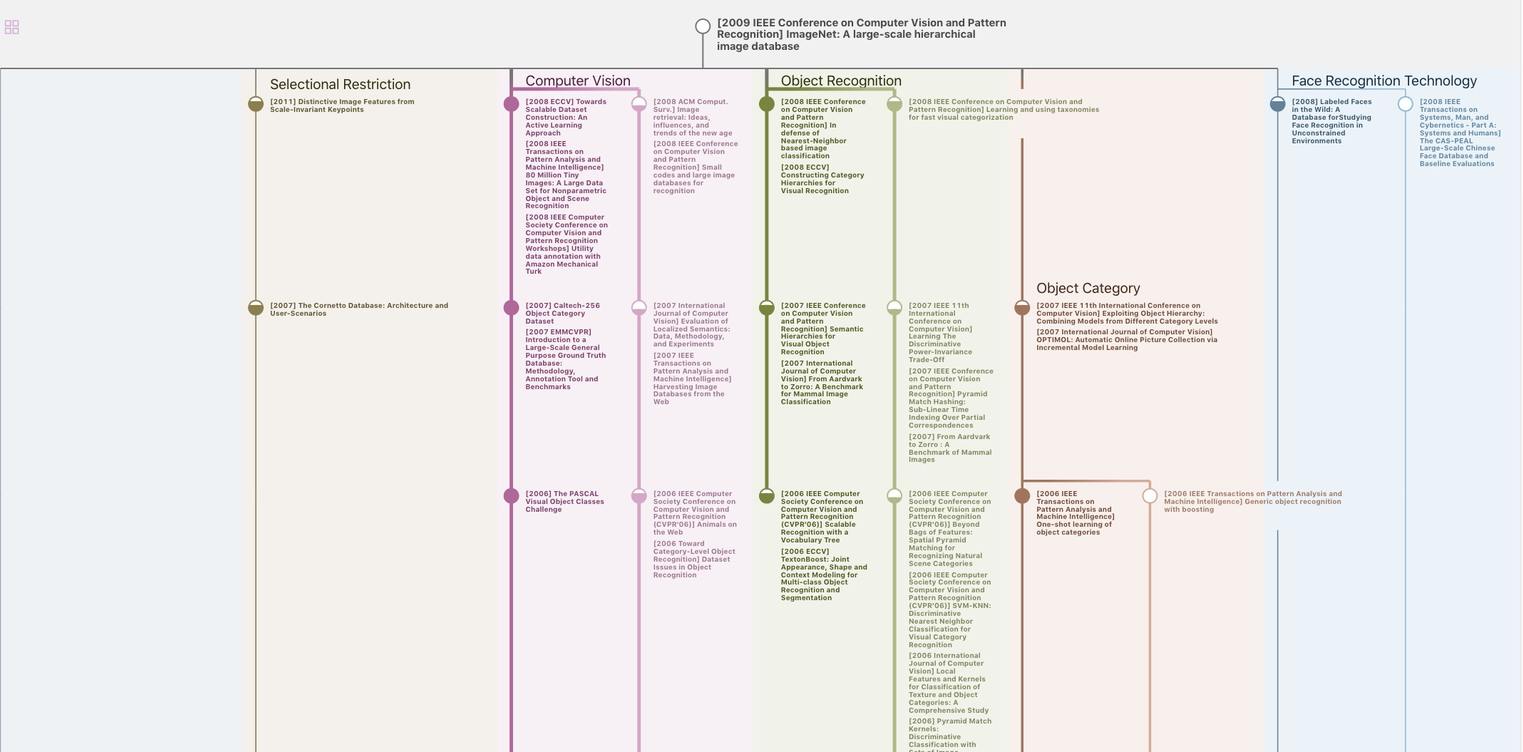
生成溯源树,研究论文发展脉络
Chat Paper
正在生成论文摘要