ZGUL: Zero-shot Generalization to Unseen Languages using Multi-source Ensembling of Language Adapters
CoRR(2023)
摘要
We tackle the problem of zero-shot cross-lingual transfer in NLP tasks via the use of language adapters (LAs). Most of the earlier works have explored training with adapter of a single source (often English), and testing either using the target LA or LA of another related language. Training target LA requires unlabeled data, which may not be readily available for low resource unseen languages: those that are neither seen by the underlying multilingual language model (e.g., mBERT), nor do we have any (labeled or unlabeled) data for them. We posit that for more effective cross-lingual transfer, instead of just one source LA, we need to leverage LAs of multiple (linguistically or geographically related) source languages, both at train and test-time - which we investigate via our novel neural architecture, ZGUL. Extensive experimentation across four language groups, covering 15 unseen target languages, demonstrates improvements of up to 3.2 average F1 points over standard fine-tuning and other strong baselines on POS tagging and NER tasks. We also extend ZGUL to settings where either (1) some unlabeled data or (2) few-shot training examples are available for the target language. We find that ZGUL continues to outperform baselines in these settings too.
更多查看译文
关键词
unseen languages,ensembling,generalization,zero-shot,multi-source
AI 理解论文
溯源树
样例
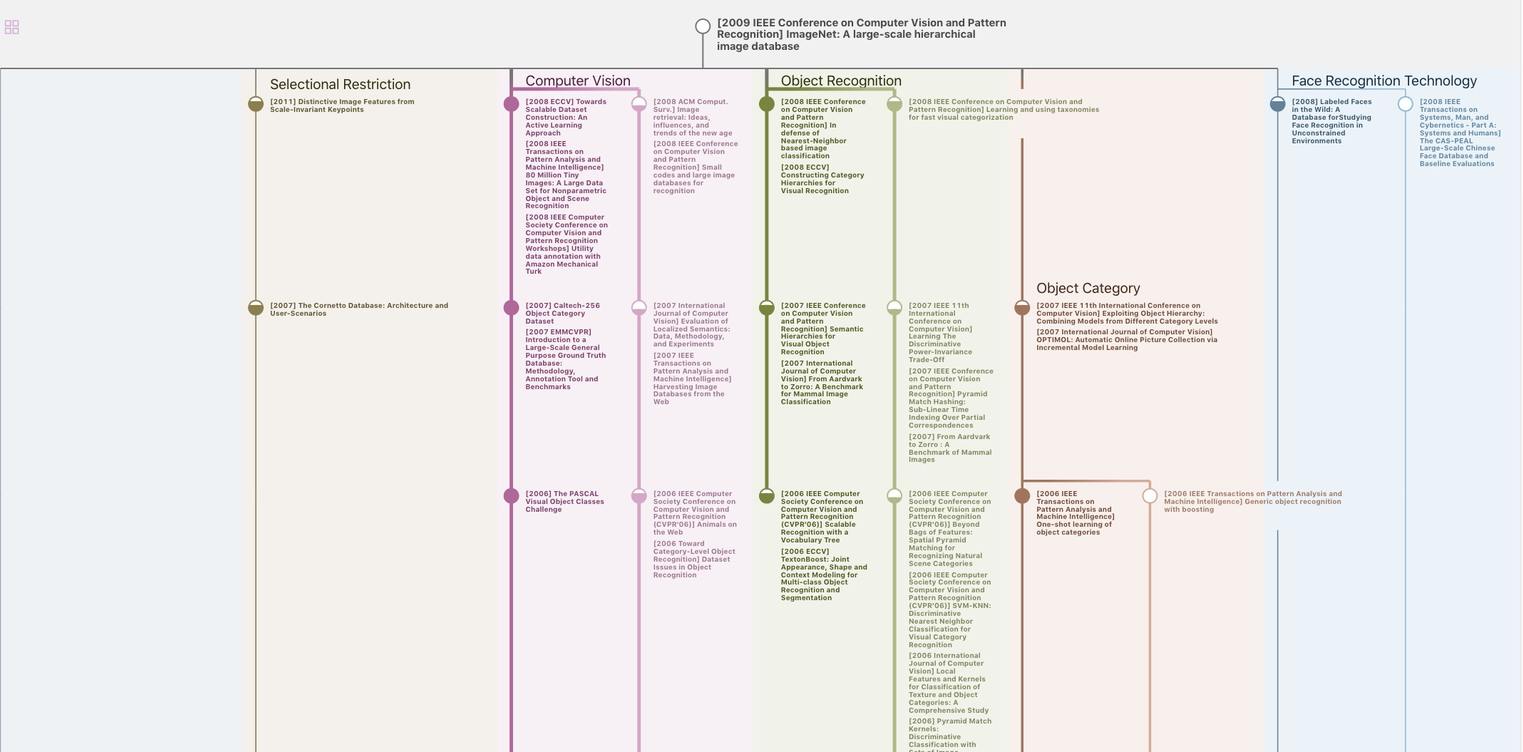
生成溯源树,研究论文发展脉络
Chat Paper
正在生成论文摘要