URL-BERT: Training Webpage Representations via Social Media Engagements
CoRR(2023)
摘要
Understanding and representing webpages is crucial to online social networks where users may share and engage with URLs. Common language model (LM) encoders such as BERT can be used to understand and represent the textual content of webpages. However, these representations may not model thematic information of web domains and URLs or accurately capture their appeal to social media users. In this work, we introduce a new pre-training objective that can be used to adapt LMs to understand URLs and webpages. Our proposed framework consists of two steps: (1) scalable graph embeddings to learn shallow representations of URLs based on user engagement on social media and (2) a contrastive objective that aligns LM representations with the aforementioned graph-based representation. We apply our framework to the multilingual version of BERT to obtain the model URL-BERT. We experimentally demonstrate that our continued pre-training approach improves webpage understanding on a variety of tasks and Twitter internal and external benchmarks.
更多查看译文
关键词
training webpage representations,social media,url-bert
AI 理解论文
溯源树
样例
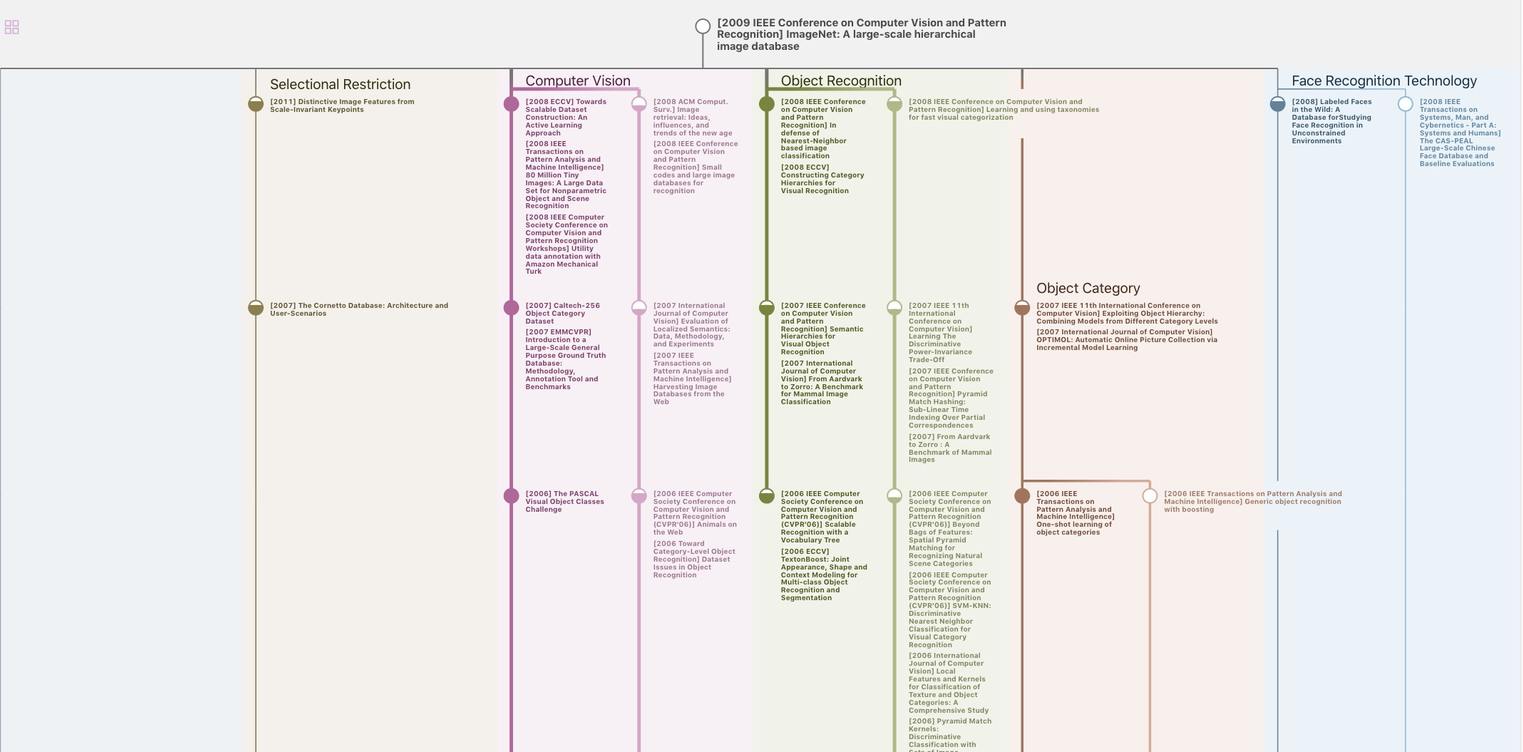
生成溯源树,研究论文发展脉络
Chat Paper
正在生成论文摘要