The Distributional Hypothesis Does Not Fully Explain the Benefits of Masked Language Model Pretraining.
CoRR(2023)
摘要
We analyze the masked language modeling pretraining objective function from the perspective of the distributional hypothesis. We investigate whether better sample efficiency and the better generalization capability of models pretrained with masked language modeling can be attributed to the semantic similarity encoded in the pretraining data's distributional property. Via a synthetic dataset, our analysis suggests that distributional property indeed leads to the better sample efficiency of pretrained masked language models, but does not fully explain the generalization capability. We also conduct analyses over two real-world datasets and demonstrate that the distributional property does not explain the generalization ability of pretrained natural language models either. Our results illustrate our limited understanding of model pretraining and provide future research directions.
更多查看译文
关键词
masked language model pretraining,distributional hypothesis
AI 理解论文
溯源树
样例
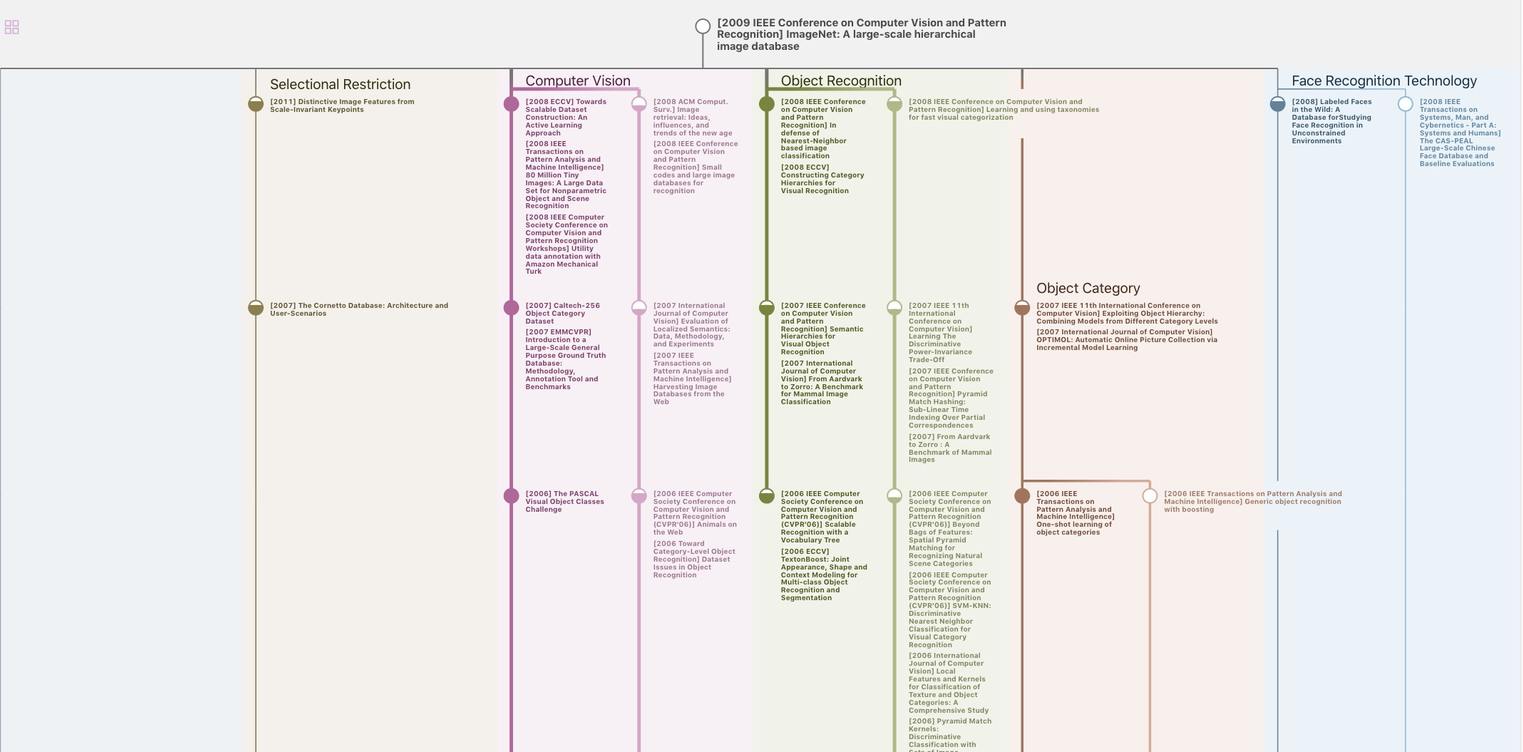
生成溯源树,研究论文发展脉络
Chat Paper
正在生成论文摘要