Towards long-tailed, multi-label disease classification from chest X-ray: Overview of the CXR-LT challenge
arxiv(2023)
摘要
Many real-world image recognition problems, such as diagnostic medical
imaging exams, are "long-tailed" x2013 there are a few common
findings followed by many more relatively rare conditions. In chest
radiography, diagnosis is both a long-tailed and multi-label problem, as
patients often present with multiple findings simultaneously. While researchers
have begun to study the problem of long-tailed learning in medical image
recognition, few have studied the interaction of label imbalance and label
co-occurrence posed by long-tailed, multi-label disease classification. To
engage with the research community on this emerging topic, we conducted an open
challenge, CXR-LT, on long-tailed, multi-label thorax disease classification
from chest X-rays (CXRs). We publicly release a large-scale benchmark dataset
of over 350,000 CXRs, each labeled with at least one of 26 clinical findings
following a long-tailed distribution. We synthesize common themes of
top-performing solutions, providing practical recommendations for long-tailed,
multi-label medical image classification. Finally, we use these insights to
propose a path forward involving vision-language foundation models for few- and
zero-shot disease classification.
更多查看译文
AI 理解论文
溯源树
样例
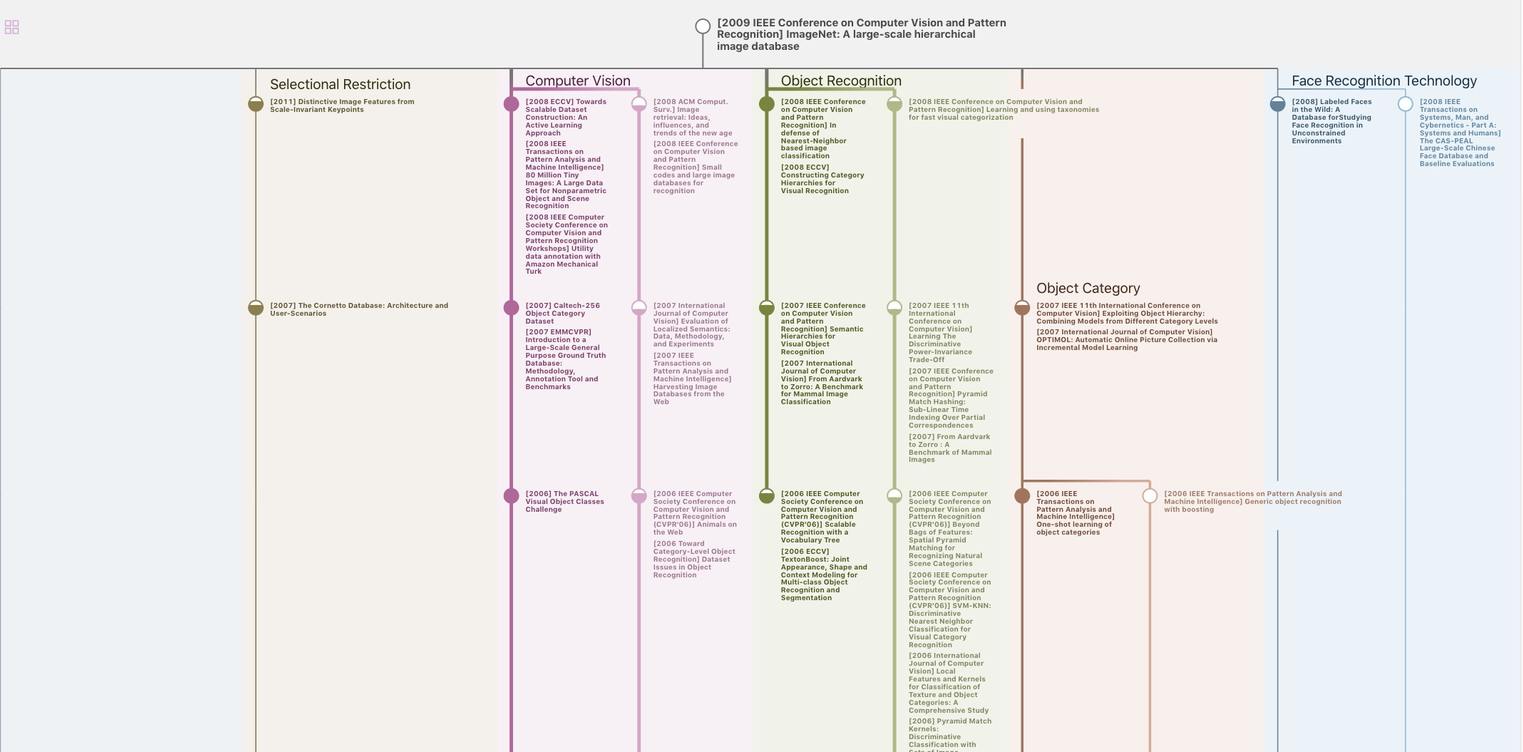
生成溯源树,研究论文发展脉络
Chat Paper
正在生成论文摘要