Image Segmentation using U-Net Architecture for Powder X-ray Diffraction Images
CoRR(2023)
摘要
Scientific researchers frequently use the in situ synchrotron high-energy powder X-ray diffraction (XRD) technique to examine the crystallographic structures of materials in functional devices such as rechargeable battery materials. We propose a method for identifying artifacts in experimental XRD images. The proposed method uses deep learning convolutional neural network architectures, such as tunable U-Nets to identify the artifacts. In particular, the predicted artifacts are evaluated against the corresponding ground truth (manually implemented) using the overall true positive rate or recall. The result demonstrates that the U-Nets can consistently produce great recall performance at 92.4% on the test dataset, which is not included in the training, with a 34% reduction in average false positives in comparison to the conventional method. The U-Nets also reduce the time required to identify and separate artifacts by more than 50%. Furthermore, the exclusion of the artifacts shows major changes in the integrated 1D XRD pattern, enhancing further analysis of the post-processing XRD data.
更多查看译文
关键词
segmentation,powder,images,u-net,x-ray
AI 理解论文
溯源树
样例
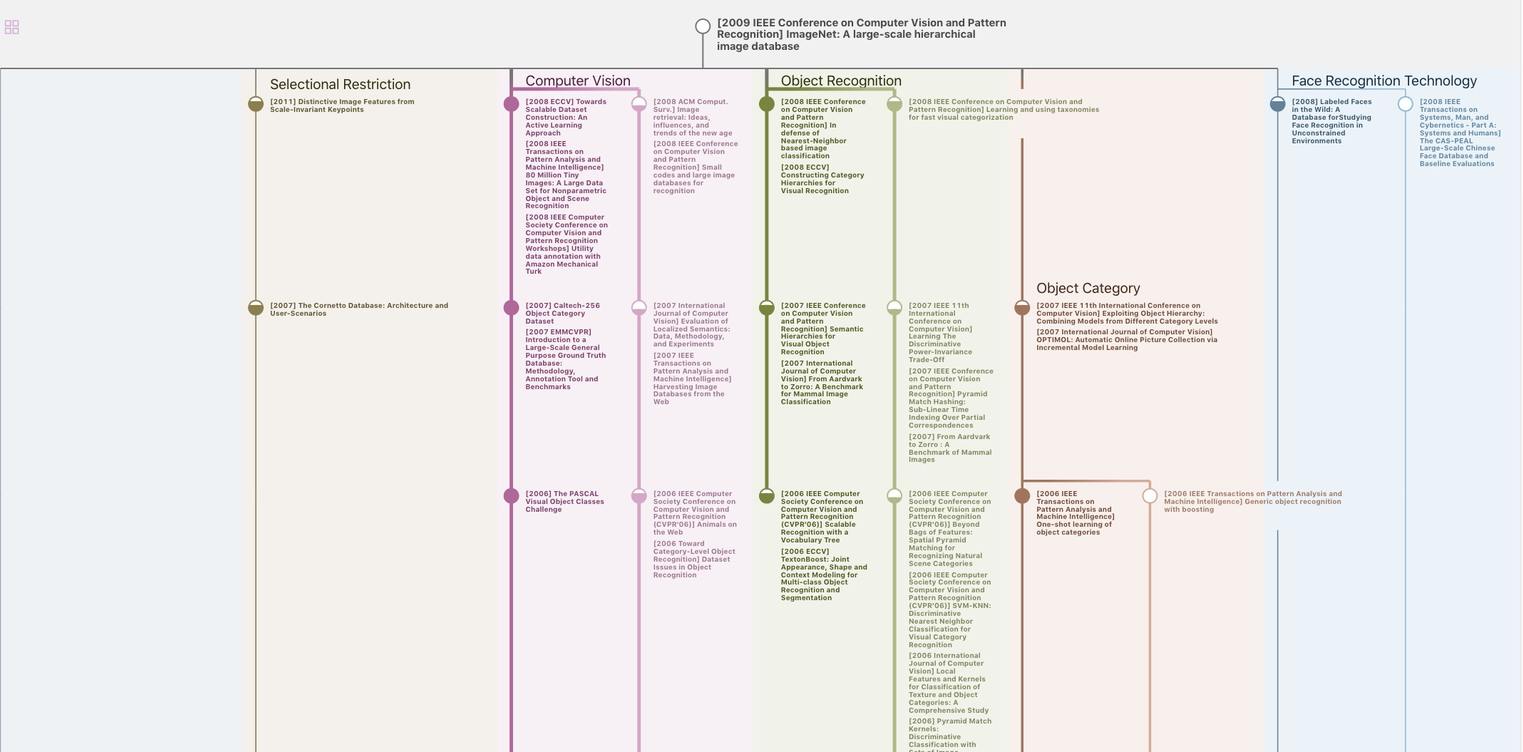
生成溯源树,研究论文发展脉络
Chat Paper
正在生成论文摘要