Unsupervised Tensor Based Feature Extraction From Multivariate Time Series
IEEE Access(2023)
摘要
Clustering and outlier detection for multivariate time series are essential tasks in data mining fields and many algorithms have been developed for this purpose. However, these tasks remain challenging because both time-wise and variable-wise associations should be taken into account to treat multivariate time series appropriately. We propose a tensor based feature extraction method called UFEKT, which focuses on subsequences to account for the time-wise association and constructs a feature vector for each subsequence by applying tensor decomposition to account for the variable-wise association. This method is simple and can be used as an effective means of preprocessing for clustering and outlier detection algorithms. We show empirically that UFEKT leads to superior performance on various popularly used clustering algorithms such as ${K}$ -means and DBSCAN and outlier detection algorithm such as the $\kappa $ -nearest neighbor and LOF.
更多查看译文
关键词
Feature extraction, multivariate time series, tensor decomposition, Tucker decomposition, clustering, outlier detection, unsupervised learning
AI 理解论文
溯源树
样例
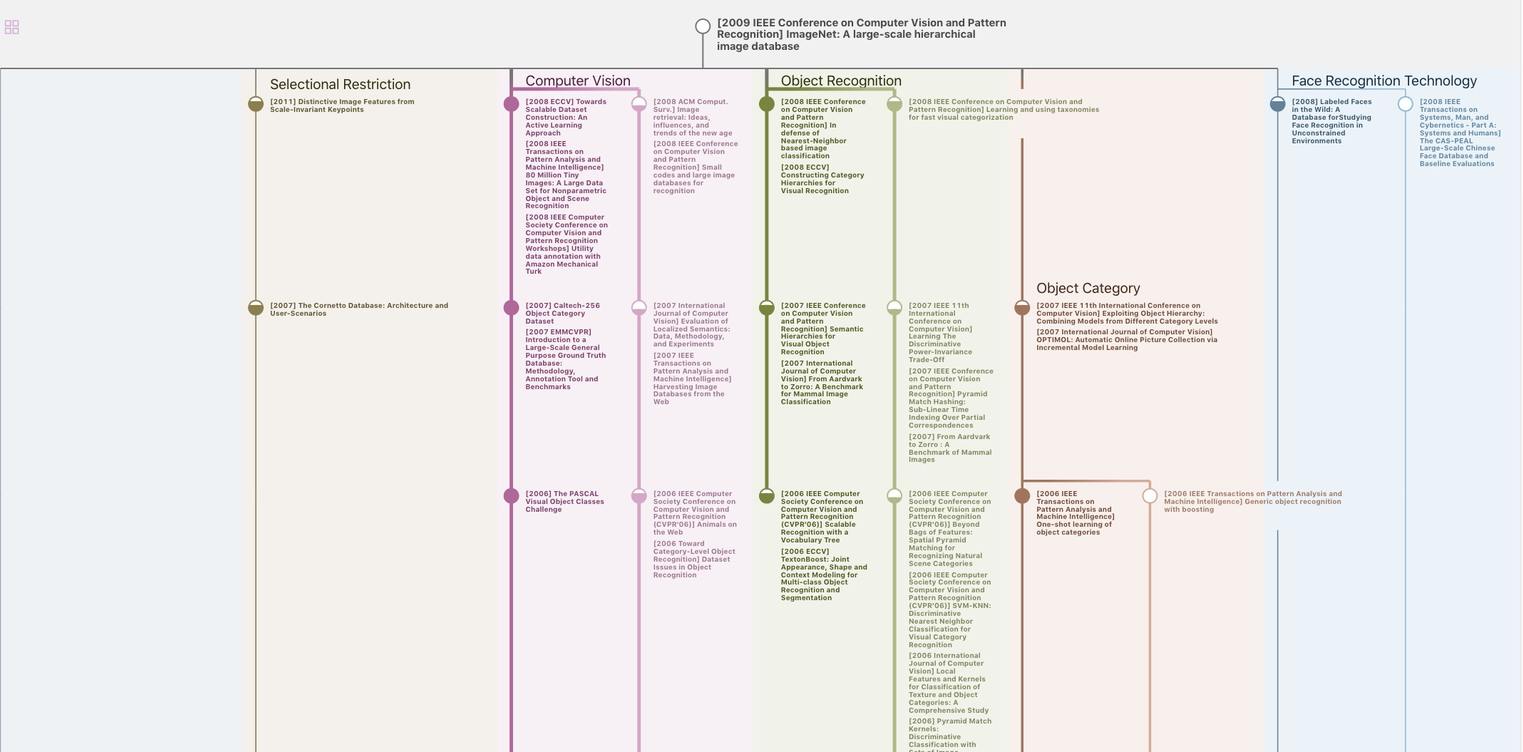
生成溯源树,研究论文发展脉络
Chat Paper
正在生成论文摘要