Super-resolution image reconstruction based on convolutional sparse coding and generative adversarial networks
CHINESE JOURNAL OF LIQUID CRYSTALS AND DISPLAYS(2023)
摘要
To address the problems of high-frequency information missing and increased noise in images generated by existing image super-resolution reconstruction algorithms, this paper proposes an image super-resolution reconstruction model based on convolutional sparse coding and generative adversarial networks. Firstly,convolutional networks are employed to implement sparse coding and obtain a sparse representation of the image,which makes full use of the prior information of the image and effectively avoids the problems of high-frequency information missing and increased noise in the reconstructed image. After obtaining the sparse representation of the low-resolution image,the sparse representation is reconstructed by the reconstruction module to obtain the super- resolution image. Subsequently, the discriminator discriminates the reconstructed image to alleviate the problem that the reconstructed image tends to be smooth due to the PSNR-dominated algorithm. After continuous adversarial training,the final generated super-resolution images are made to have better visual effects. The super-resolution reconstruction experiments are performed on Set5,Set14,BSD100 and Urban100 general test datasets at 2x and 4x and compared with Bicubic,SRGAN,EDSR and ESRGAN methods. Compared with ESRGAN,the average PSNR improvement is about 0. 702 8 dB,the average SSIM improvement is about 0. 047,and the average LPIPS improvement is 0. 016 on the four datasets. Experimental results show that the proposed model is highly competitive and enables the recovery of more fine-texture details with better definition.
更多查看译文
关键词
image processing,image super resolution,sparse representation,generative adversarial network
AI 理解论文
溯源树
样例
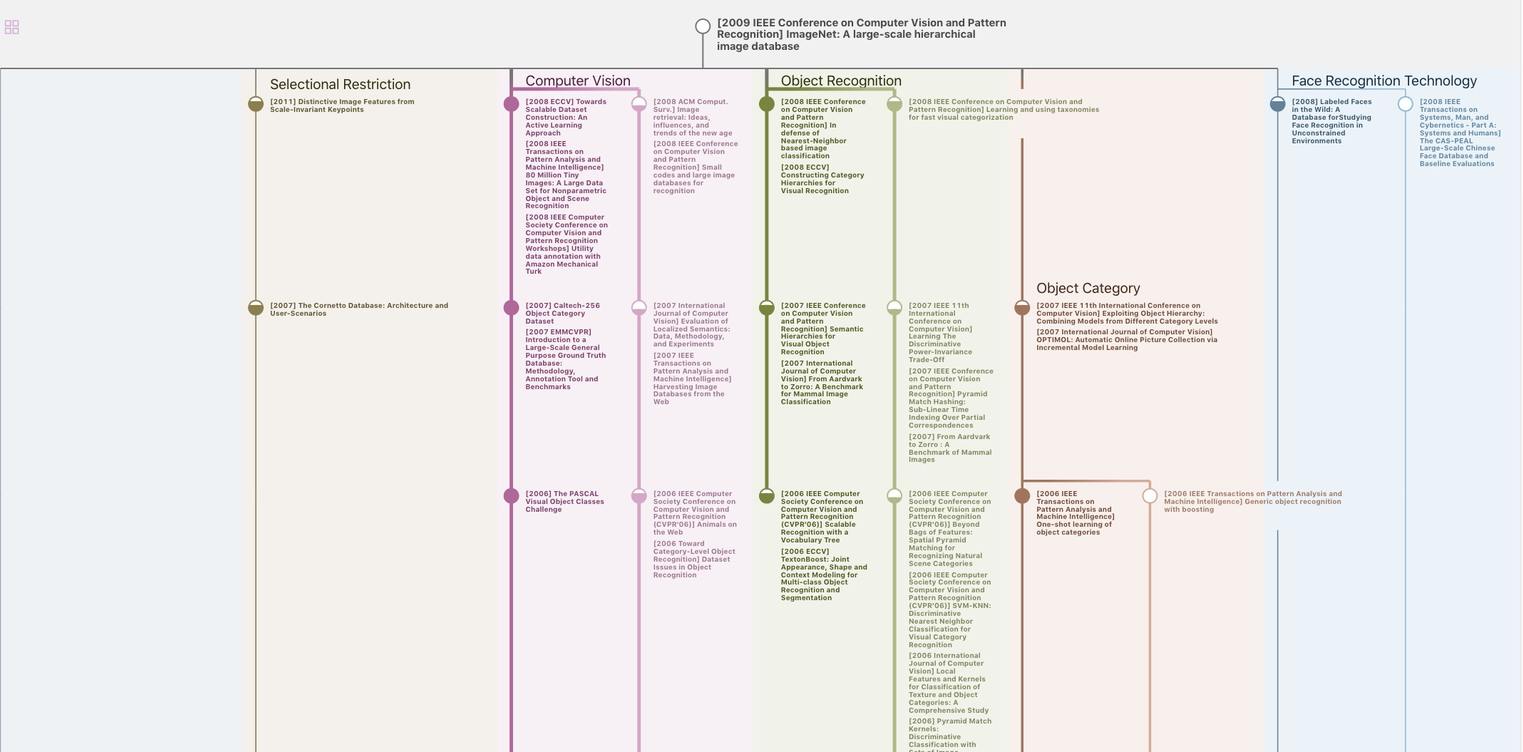
生成溯源树,研究论文发展脉络
Chat Paper
正在生成论文摘要