A Novel Neural-Network Device Modeling Based on Physics-Informed Machine Learning
IEEE Transactions on Electron Devices(2023)
摘要
In this work, we present a novel physics-informed machine learning (PIML)-based neural-network device modeling that predicts both device performance and spatial physical quantities in real-time. Using cutting-edge technologies such as physics-informed neural network (NN) and physics-informed deep operator networks, our approach suggests interpolation and extrapolation strategies in device physics modeling. Despite being trained with a small number of bias voltages, our model demonstrates remarkable accuracy, with a mean absolute percentage error (MAPE) of 0.12% for predicting potential for interpolation and 0.19% for extrapolation. Our approach can be used for data-efficient NN modeling for TCAD and real-time physics analysis in the spatial domain.
更多查看译文
关键词
Deep operator network, nanowire, neural network (NN), physics-informed machine learning (PIML)
AI 理解论文
溯源树
样例
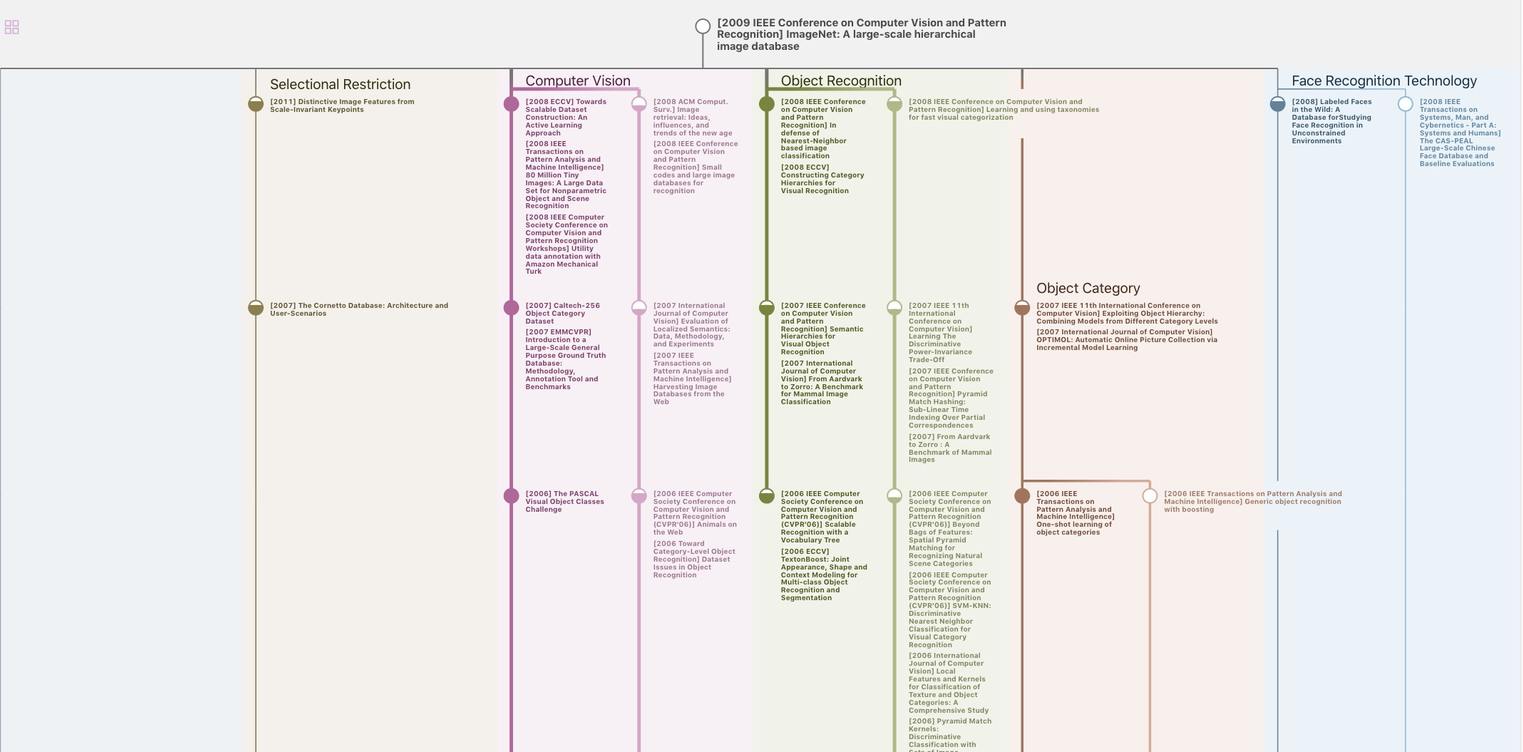
生成溯源树,研究论文发展脉络
Chat Paper
正在生成论文摘要