Communication-Efficient and Byzantine-Robust Federated Learning for Mobile Edge Computing Networks
IEEE NETWORK(2023)
摘要
Federated learning in mobile edge computing allows a larger number of devices to jointly train an accurate machine learning model without collecting local data from edge nodes. However, there are two major challenges to using federated learning for mobile edge computing. The first is that mobile edge computing networks only tolerate a limited communication overhead, that is, communication overhead between edge nodes, edge servers, and cloud servers cannot be excessive. Unfortunately, federated learning clients send a large number of local model update that do not meet realistic requirements. The second is that resource-constrained edge nodes are vulnera-ble to attacks, that is, these edge nodes are highly vulnerable to potential adversary compromise and can be used to launch Byzantine attacks. To address the aforementioned challenges, we propose a communication-efficient and Byzan-tine-robust federated learning for mobile edge computing networks named CBFL. Specifically, edge nodes compress local model updates by taking element-wise signs for local model updates. Then, edge nodes sends it to the edge server for local model aggregation. Finally, the cloud server uses a small evaluation dataset to evaluate the edge servers' local model aggregation results and utilizes the evaluation results for global model aggregation. Moreover, extensive experiments are also conducted to evaluate the performance of the proposed CBFL, and the results show that our CBFL can withstand Byzantine attacks while maintaining communication efficiency.
更多查看译文
关键词
Training,Threat modeling,Multi-access edge computing,Federated learning,Computational modeling,Data models,Servers,Machine learning
AI 理解论文
溯源树
样例
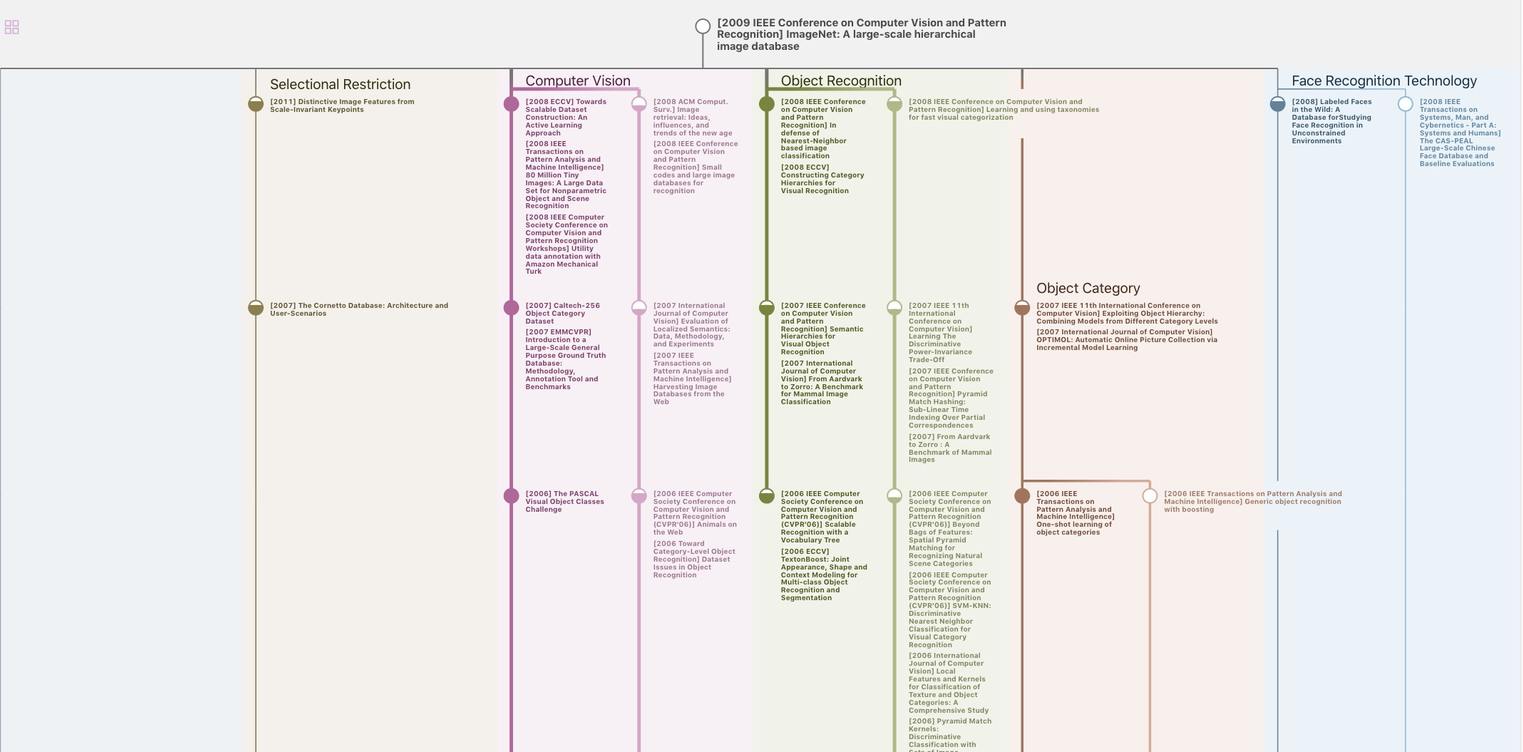
生成溯源树,研究论文发展脉络
Chat Paper
正在生成论文摘要