Prescription Recommendation based on Intention Retrieval Network and Multimodal Medical Indicators
Proceedings of the 1st International Workshop on Deep Multimodal Learning for Information Retrieval(2023)
摘要
Knowledge based Clinical Decision Support Systems can provide precise and interpretable results for prescription recommendation. Many existing knowledge based prescription recommendation systems take into account multi-modal historical medical events to learn from past experiences. However, such approaches treat those events as independent, static information and neglect the fact that patient history is a set of chronological events. Hence, they lack the ability to extract the dynamics of prescription intentions and cannot provide precise and interpretable results for chronological disease patients with long-term or repeating visits. To address these limitations, we propose a novel Intention Aware Conditional Generation Net (IACoGNet), which introduces an optimized copy-or-predict mechanism to learn precription intentions from multi-modal health datasets and generate drug recommendations. IACoGNet first designs a knowledge representation model that captures multi-modal patient features. Then, it proposes a novel prescription intention representation model in the multi-visit scenario and predicts the diagnostic intention. Finally, it constructs a prescription recommendation framework utilizing the above two knowledge representations. We validate IACoGNet on the public MIMIC data set, and the experimental results show that IACoGNet can achieve optimum in F1 score and average precision.
更多查看译文
关键词
Multimodal information extraction,Prescription recommendation,Electronic health record
AI 理解论文
溯源树
样例
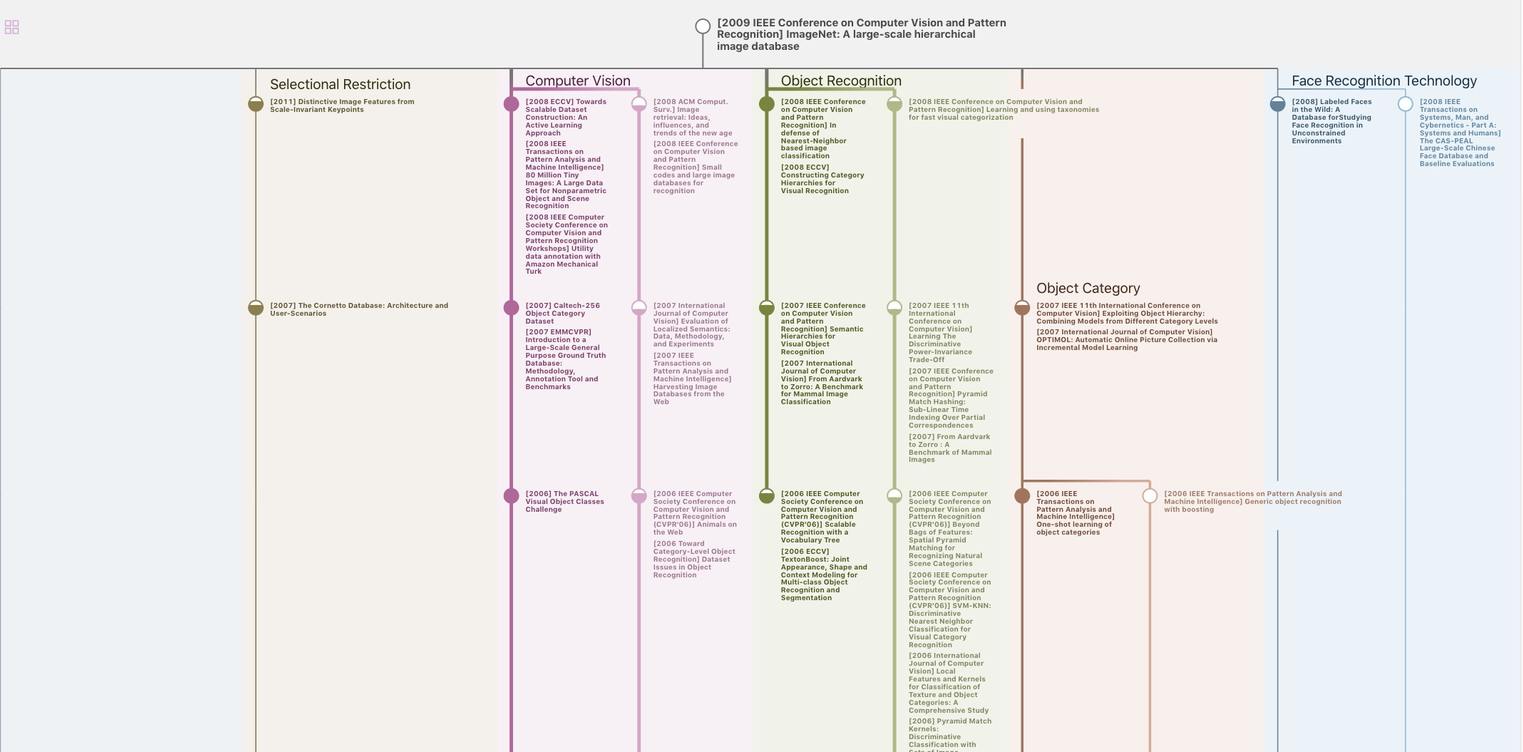
生成溯源树,研究论文发展脉络
Chat Paper
正在生成论文摘要