Improving Event Time Prediction by Learning to Partition the Event Time Space
CoRR(2023)
摘要
Recently developed survival analysis methods improve upon existing approaches by predicting the probability of event occurrence in each of a number pre-specified (discrete) time intervals. By avoiding placing strong parametric assumptions on the event density, this approach tends to improve prediction performance, particularly when data are plentiful. However, in clinical settings with limited available data, it is often preferable to judiciously partition the event time space into a limited number of intervals well suited to the prediction task at hand. In this work, we develop a method to learn from data a set of cut points defining such a partition. We show that in two simulated datasets, we are able to recover intervals that match the underlying generative model. We then demonstrate improved prediction performance on three real-world observational datasets, including a large, newly harmonized stroke risk prediction dataset. Finally, we argue that our approach facilitates clinical decision-making by suggesting time intervals that are most appropriate for each task, in the sense that they facilitate more accurate risk prediction.
更多查看译文
关键词
event time prediction,event time,learning
AI 理解论文
溯源树
样例
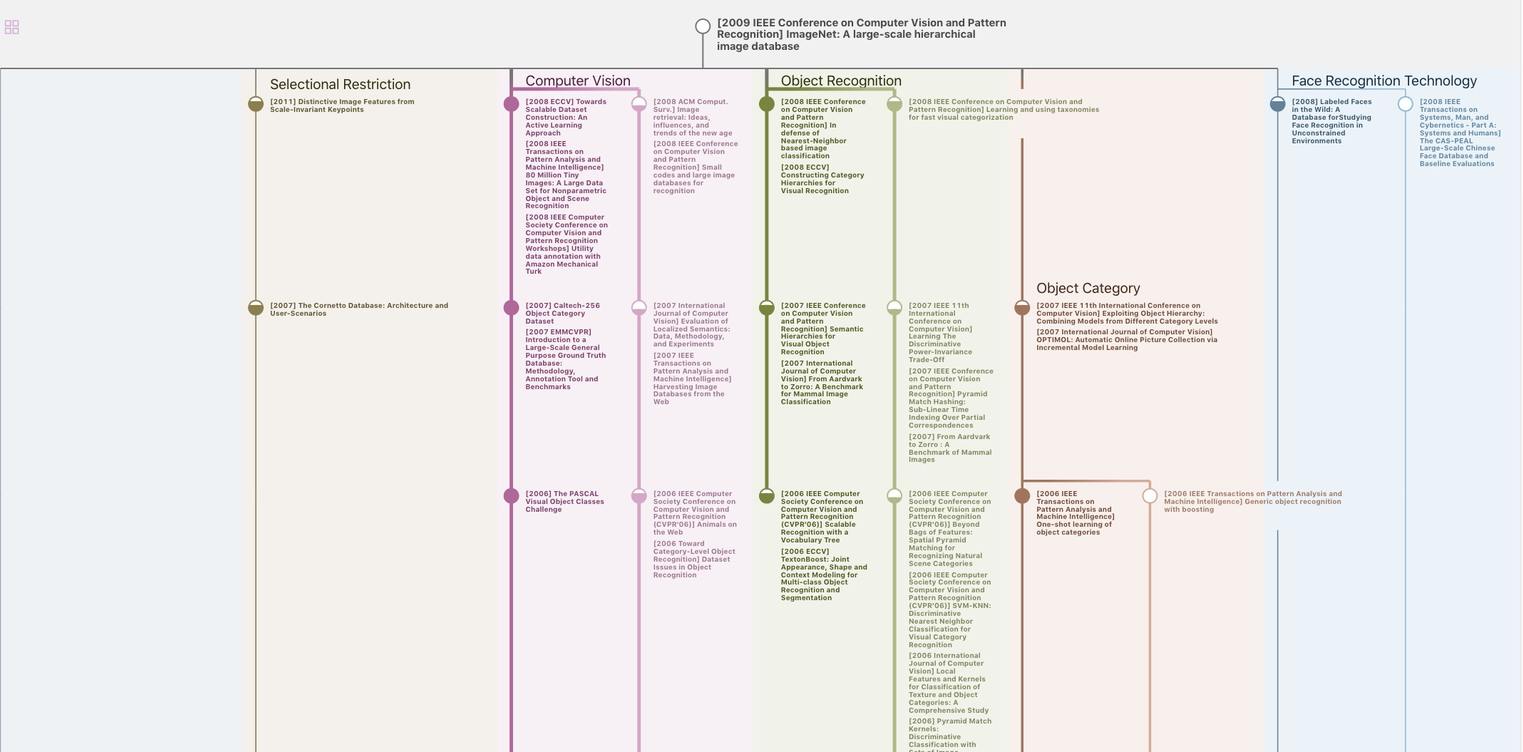
生成溯源树,研究论文发展脉络
Chat Paper
正在生成论文摘要