BLESS: Benchmarking Large Language Models on Sentence Simplification.
CoRR(2023)
摘要
We present BLESS, a comprehensive performance benchmark of the most recent state-of-the-art large language models (LLMs) on the task of text simplification (TS). We examine how well off-the-shelf LLMs can solve this challenging task, assessing a total of 44 models, differing in size, architecture, pre-training methods, and accessibility, on three test sets from different domains (Wikipedia, news, and medical) under a few-shot setting. Our analysis considers a suite of automatic metrics as well as a large-scale quantitative investigation into the types of common edit operations performed by the different models. Furthermore, we perform a manual qualitative analysis on a subset of model outputs to better gauge the quality of the generated simplifications. Our evaluation indicates that the best LLMs, despite not being trained on TS, perform comparably with state-of-the-art TS baselines. Additionally, we find that certain LLMs demonstrate a greater range and diversity of edit operations. Our performance benchmark will be available as a resource for the development of future TS methods and evaluation metrics.
更多查看译文
关键词
large language models,language models
AI 理解论文
溯源树
样例
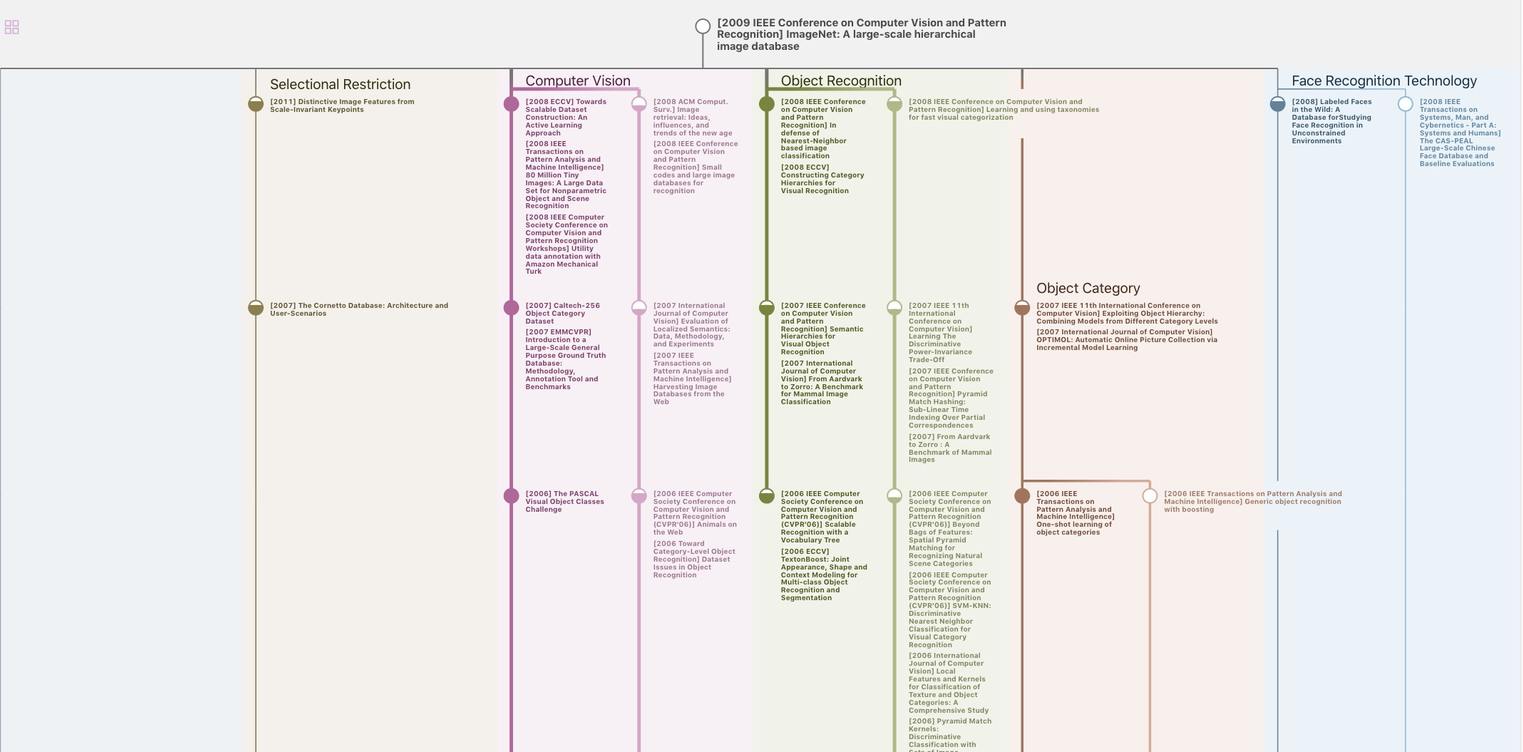
生成溯源树,研究论文发展脉络
Chat Paper
正在生成论文摘要