Recurrent Linear Transformers
CoRR(2023)
摘要
The self-attention mechanism in the transformer architecture is capable of capturing long-range dependencies and it is the main reason behind its effectiveness in processing sequential data. Nevertheless, despite their success, transformers have two significant drawbacks that still limit their broader applicability: (1) In order to remember past information, the self-attention mechanism requires access to the whole history to be provided as context. (2) The inference cost in transformers is expensive. In this paper we introduce recurrent alternatives to the transformer self-attention mechanism that offer a context-independent inference cost, leverage long-range dependencies effectively, and perform well in practice. We evaluate our approaches in reinforcement learning problems where the aforementioned computational limitations make the application of transformers nearly infeasible. We quantify the impact of the different components of our architecture in a diagnostic environment and assess performance gains in 2D and 3D pixel-based partially-observable environments. When compared to a state-of-the-art architecture, GTrXL, inference in our approach is at least 40% cheaper while reducing memory use in more than 50%. Our approach either performs similarly or better than GTrXL, improving more than 37% upon GTrXL performance on harder tasks.
更多查看译文
关键词
recurrent linear transformers
AI 理解论文
溯源树
样例
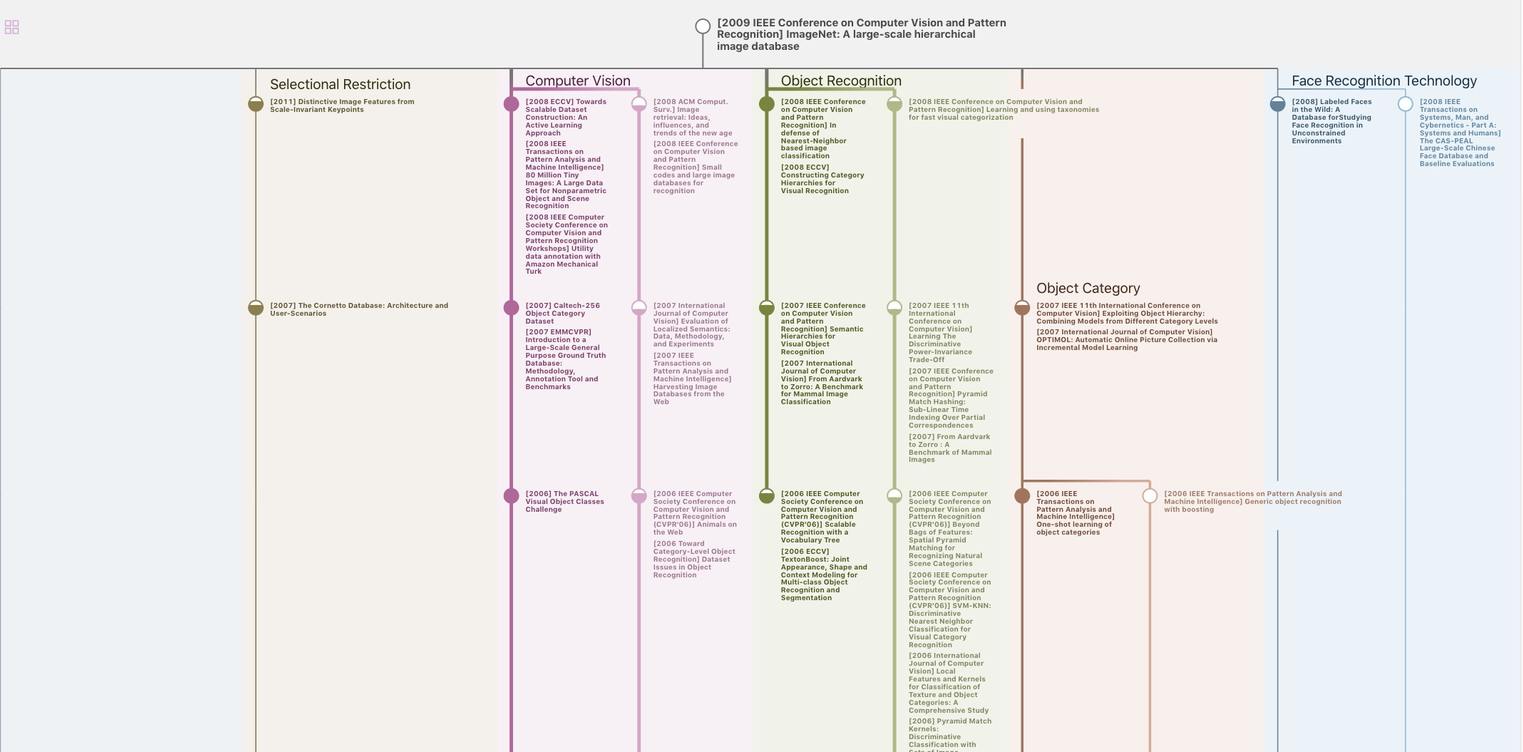
生成溯源树,研究论文发展脉络
Chat Paper
正在生成论文摘要