Sparse Bayesian neural networks for regression: Tackling overfitting and computational challenges in uncertainty quantification
CoRR(2023)
摘要
Neural networks (NNs) are primarily developed within the frequentist statistical framework. Nevertheless, frequentist NNs lack the capability to provide uncertainties in the predictions, and hence their robustness can not be adequately assessed. Conversely, the Bayesian neural networks (BNNs) naturally offer predictive uncertainty by applying Bayes' theorem. However, their computational requirements pose significant challenges. Moreover, both frequentist NNs and BNNs suffer from overfitting issues when dealing with noisy and sparse data, which render their predictions unwieldy away from the available data space. To address both these problems simultaneously, we leverage insights from a hierarchical setting in which the parameter priors are conditional on hyperparameters to construct a BNN by applying a semi-analytical framework known as nonlinear sparse Bayesian learning (NSBL). We call our network sparse Bayesian neural network (SBNN) which aims to address the practical and computational issues associated with BNNs. Simultaneously, imposing a sparsity-inducing prior encourages the automatic pruning of redundant parameters based on the automatic relevance determination (ARD) concept. This process involves removing redundant parameters by optimally selecting the precision of the parameters prior probability density functions (pdfs), resulting in a tractable treatment for overfitting. To demonstrate the benefits of the SBNN algorithm, the study presents an illustrative regression problem and compares the results of a BNN using standard Bayesian inference, hierarchical Bayesian inference, and a BNN equipped with the proposed algorithm. Subsequently, we demonstrate the importance of considering the full parameter posterior by comparing the results with those obtained using the Laplace approximation with and without NSBL.
更多查看译文
关键词
sparse bayesian neural networks,uncertainty quantification,regression
AI 理解论文
溯源树
样例
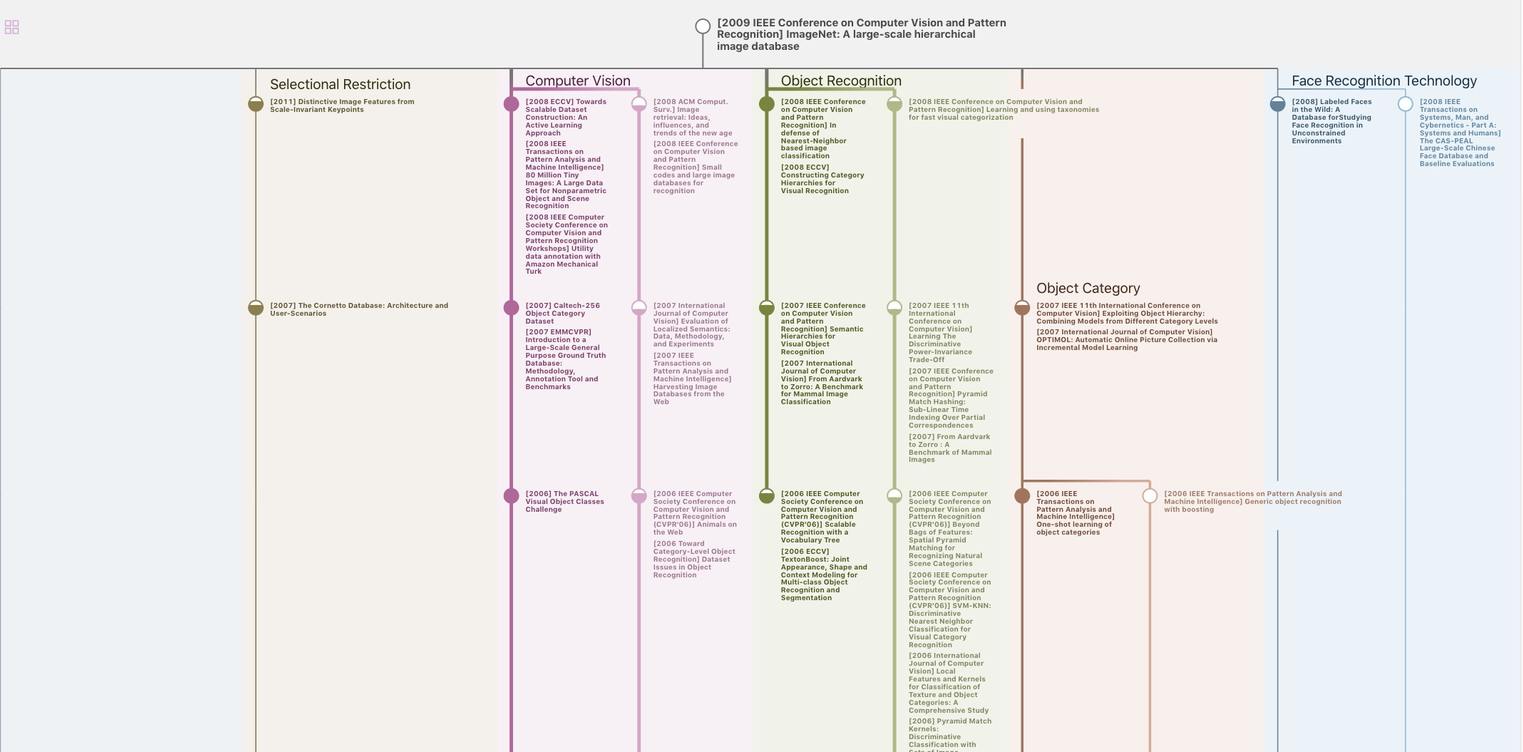
生成溯源树,研究论文发展脉络
Chat Paper
正在生成论文摘要