Breaking the Language Barrier: Improving Cross-Lingual Reasoning with Structured Self-Attention.
CoRR(2023)
摘要
In this work, we study whether multilingual language models (MultiLMs) can transfer logical reasoning abilities to other languages when they are fine-tuned for reasoning in a different language. We evaluate the cross-lingual reasoning abilities of MultiLMs in two schemes: (1) where the language of the context and the question remain the same in the new languages that are tested (i.e., the reasoning is still monolingual, but the model must transfer the learned reasoning ability across languages), and (2) where the language of the context and the question is different (which we term code-switched reasoning). On two logical reasoning datasets, RuleTaker and LeapOfThought, we demonstrate that although MultiLMs can transfer reasoning ability across languages in a monolingual setting, they struggle to transfer reasoning abilities in a code-switched setting. Following this observation, we propose a novel attention mechanism that uses a dedicated set of parameters to encourage cross-lingual attention in code-switched sequences, which improves the reasoning performance by up to 14% and 4% on the RuleTaker and LeapOfThought datasets, respectively.
更多查看译文
关键词
language barrier,cross-lingual,self-attention
AI 理解论文
溯源树
样例
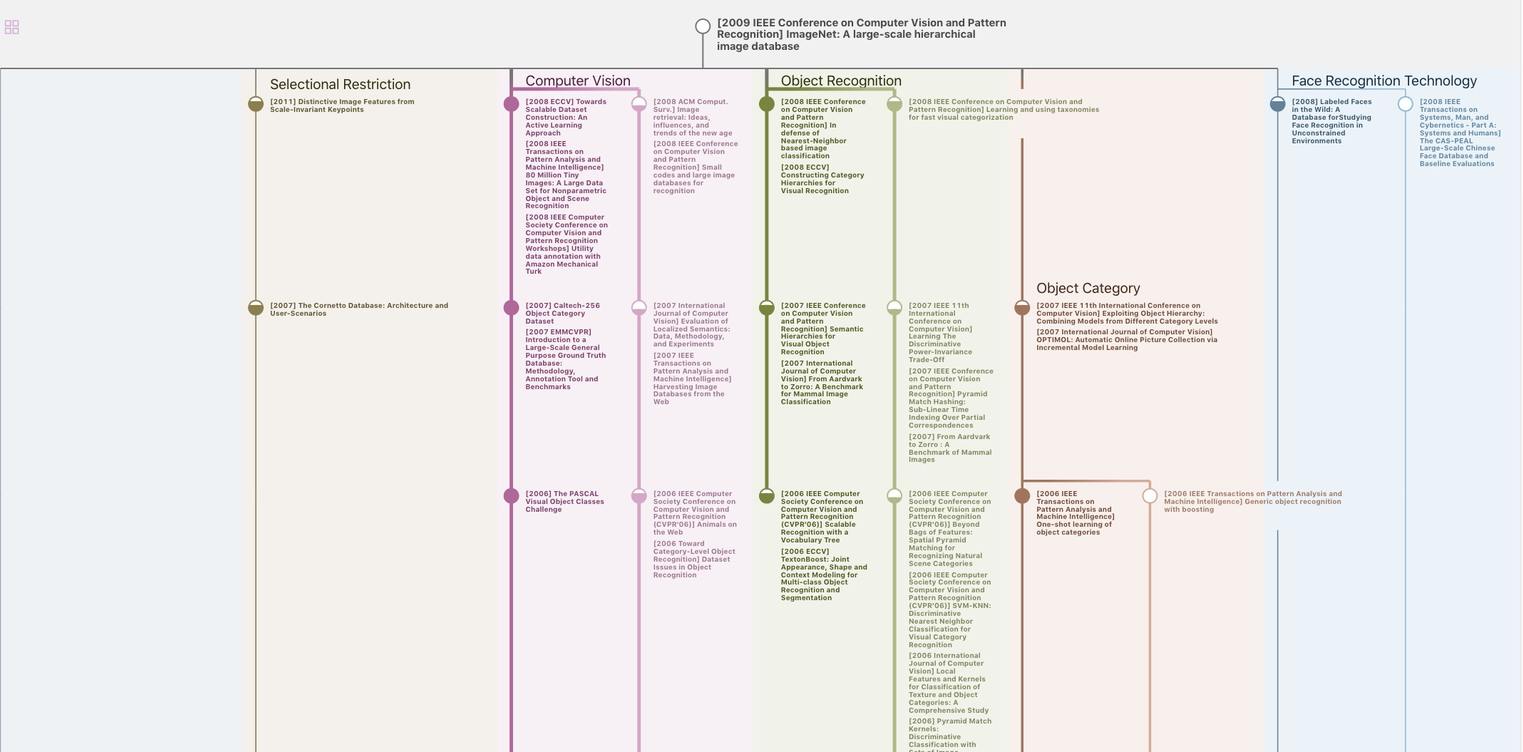
生成溯源树,研究论文发展脉络
Chat Paper
正在生成论文摘要