VersatileFL: Volatility-Resilient Federated Learning in Wireless Edge Networks
2023 20TH ANNUAL IEEE INTERNATIONAL CONFERENCE ON SENSING, COMMUNICATION, AND NETWORKING, SECON(2023)
摘要
In the era of artificial intelligence (AI), deep neural networks (DNNs) become larger using a massive amount of data, and thus, they are trained via cooperative computing devices (e.g., GPUs or servers) based on federated learning. As computation and data generation move to the edge due to privacy, latency, or bandwidth issue, DNN with edge devices has been investigated. However, edge devices are wirelessly connected and mostly incur fragile connectivity. We propose VersatileFL, a novel volatility-resilient deep learning framework under hostile environments. We address short-term and long-term volatility: 1) versatile distributed learning against short-term fluctuation by substituting the missing intermediate values with the past or approximated values and 2) model rearrangement with runtime connectivity diagnosis against long-term variation by adaptively adjusting the partitioned model for the impaired. We have demonstrated that VersatileFL has achieved 62.0 % and 31.9 % higher performance than hostile learning without a maintenance scheme against the short-term and long-term volatility, respectively.
更多查看译文
关键词
Federated Learning,Distributed Learning,Split Learning,Model Parallelism,Wireless Networks,Network Volatility,Edge Intelligence
AI 理解论文
溯源树
样例
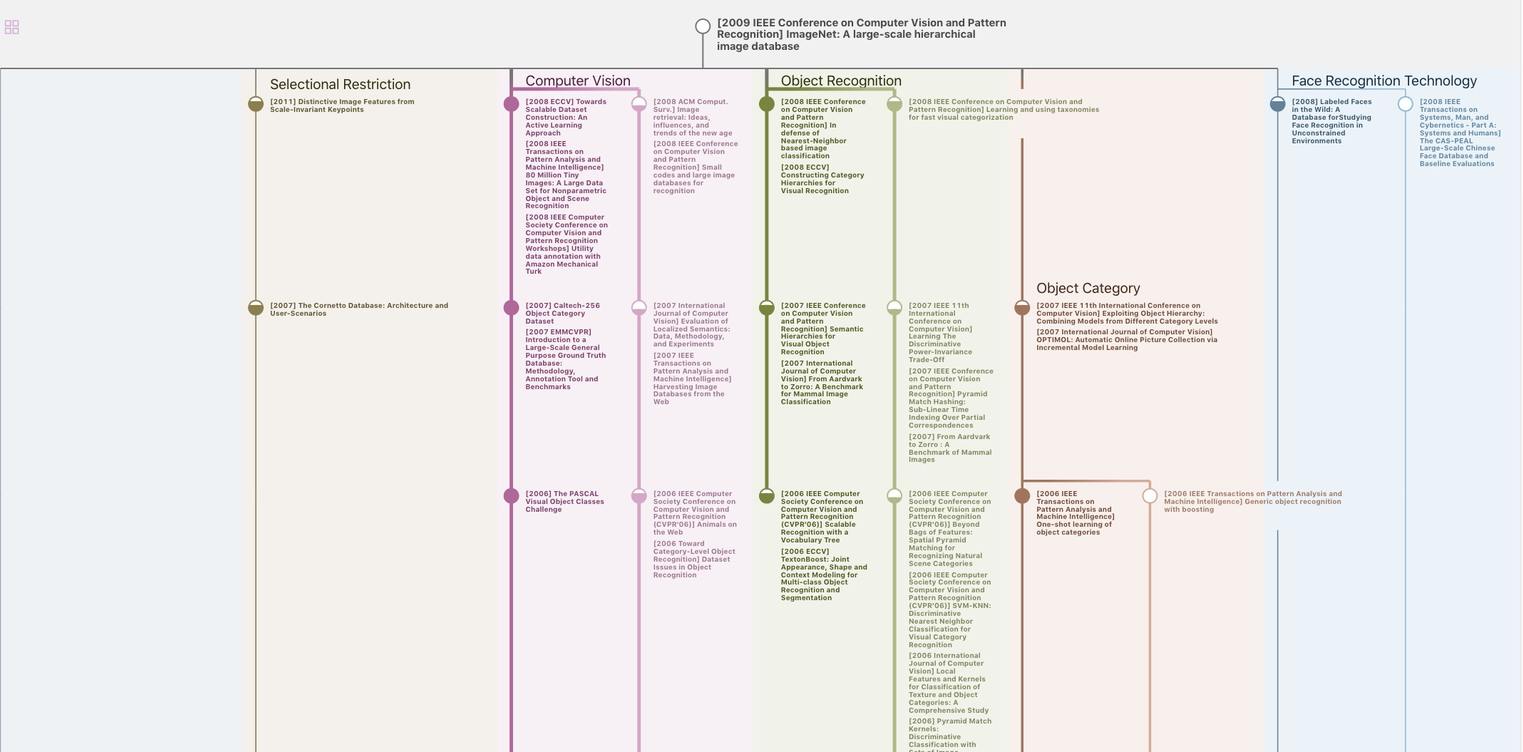
生成溯源树,研究论文发展脉络
Chat Paper
正在生成论文摘要