Rating Text Classification with Weighted Negative Supervision on Classifier Layer
CHINESE JOURNAL OF ELECTRONICS(2023)
摘要
Bidirectional encoder representations from transformers (BERT) gives full play to the advantages of the attention mechanism, improves the performance of sentence representation, and provides a better choice for various natural language understanding (NLU) tasks. Many methods using BERT as the pre-trained model achieve state-of-the-art performance in almost various text classification scenarios. Among them, the multitask learning framework combining the negative supervision and the pre-trained model solves the issue of the model performance degradation that occurs as the semantic similarity of texts conflicts with the classification standards. The current model does not consider the degree of difference between labels, which leads to insufficient difference information learned by the model, and affects classification performance, especially in the rating classification tasks. On the basis of the multi-task learning model, this paper fully considers the degree of difference between labels, which is expressed by using weights to solve the above problems. We supervise negative samples on the classifier layer instead of the encoder layer, so that the classifier layer can also learn the difference information between the labels. Experimental results show that our model can not only performs well in 2-class and multi-class rating text classification tasks, but also performs well in different languages.
更多查看译文
关键词
Natural language understanding,Rating text classification,Weighted negative supervision,Degree of difference
AI 理解论文
溯源树
样例
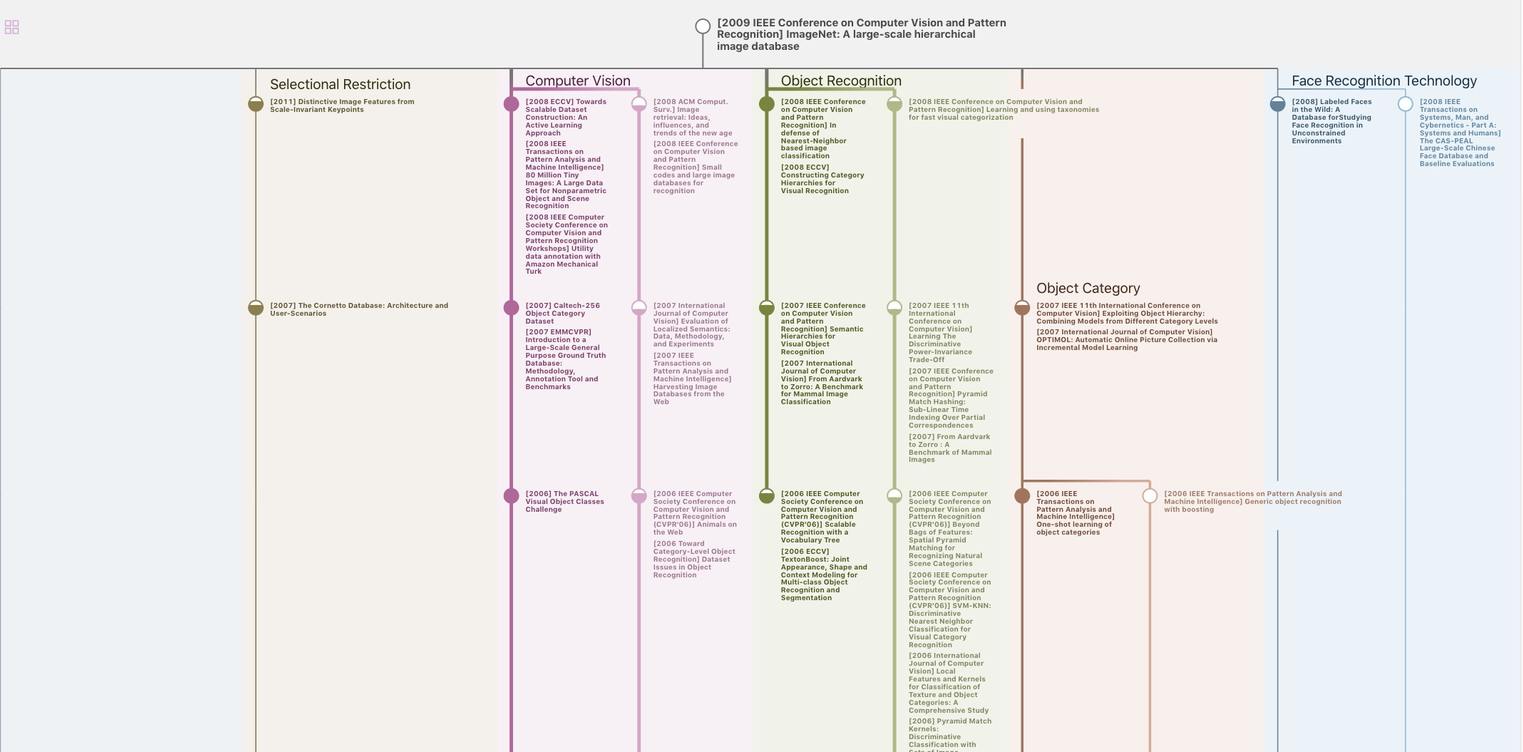
生成溯源树,研究论文发展脉络
Chat Paper
正在生成论文摘要