Using Sparse Autoencoders to Perform Blind Source Separation of High-Density Myoelectric Signal
2023 IEEE 33rd International Workshop on Machine Learning for Signal Processing (MLSP)(2023)
摘要
A recent approach to address the blind source separation (BSS) problem relies on autoencoders with a discriminator as a generative adversarial network (GAN). However, this model is difficult to train due to the lack of metrics that ensure the convergence of a GAN. In this study, we try to provide an alternative solution by replacing the discriminator with appropriate regularization functions based on prior information on the sources. We tested this solution in the high-density electromyogram (HD-EMG) separation problem for a scenario with synthetic but realistic data and compared it with a classic algorithm from the literature. An interesting feature of the proposal is that it can be easily adapted for other source separation problems. Moreover, although the performance is slightly worse than the state-of the-art HD-EMG algorithm, which considers very detailed information on the problem, our proposal provides good results with a mean rate of agreement of 91.88% between the true and estimated spikes.
更多查看译文
关键词
Blind Source Separation,Sparse Autoencoders,Generative Adversarial Networks,HD-EMG
AI 理解论文
溯源树
样例
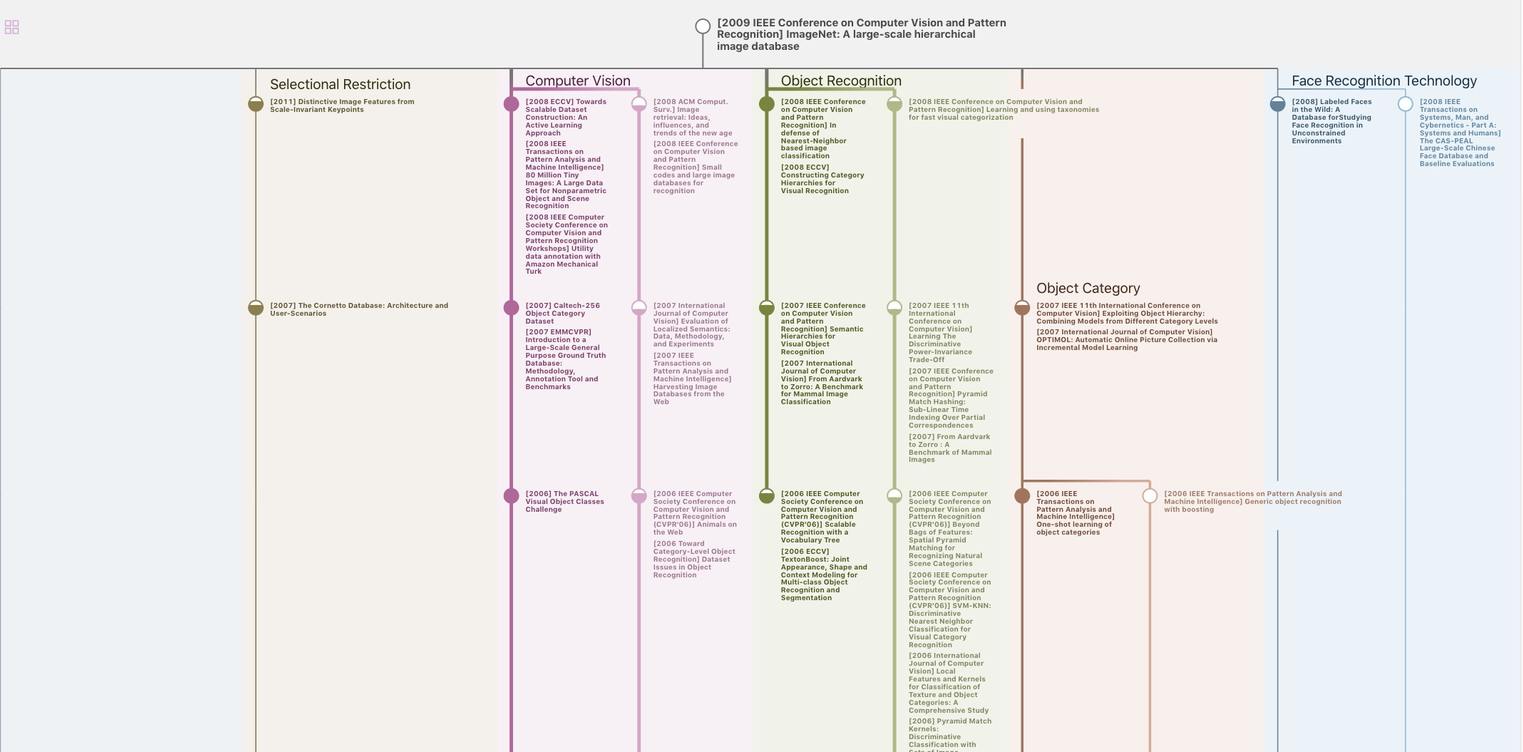
生成溯源树,研究论文发展脉络
Chat Paper
正在生成论文摘要