Reinforcement Learning for Resource Allocation with Periodic Traffic Patterns
2023 IEEE INTERNATIONAL CONFERENCE ON COMMUNICATIONS WORKSHOPS, ICC WORKSHOPS(2023)
摘要
It is common to formulate resource-allocation problems in communication networks as Markov decision process (MDP) and solve it by using deep reinforcement learning (DRL) techniques. However, this approach often cannot find the optimal action policy when task (demand) arrivals present a periodic pattern since the systems do not satisfy the underlying mathematical properties of the MDP. On the other hand, solving the periodic MDP, which can precisely model the problems under consideration, may need to generate many policies, thus requiring a prohibitive amount of computation resources and excessive training time. To achieve a balanced trade-off, we propose a DRL framework that includes procedures to determine the period of the task arrival process and partition the period into time intervals so that a sequence of MDPs are used to model the resource-allocation problems. Furthermore, a method is proposed for choosing the appropriate number of MDPs used in the framework. By using the practical task arrivals in the Alibaba dataset, our experimental results reveal that the task utilities obtained by using the proposed framework of sequential policies using DRL can yield an average improvement of 23% over those from an RL solution with one single policy.
更多查看译文
关键词
Deep Reinforcement Learning,Periodic Markov Decision Process,Resource Allocation
AI 理解论文
溯源树
样例
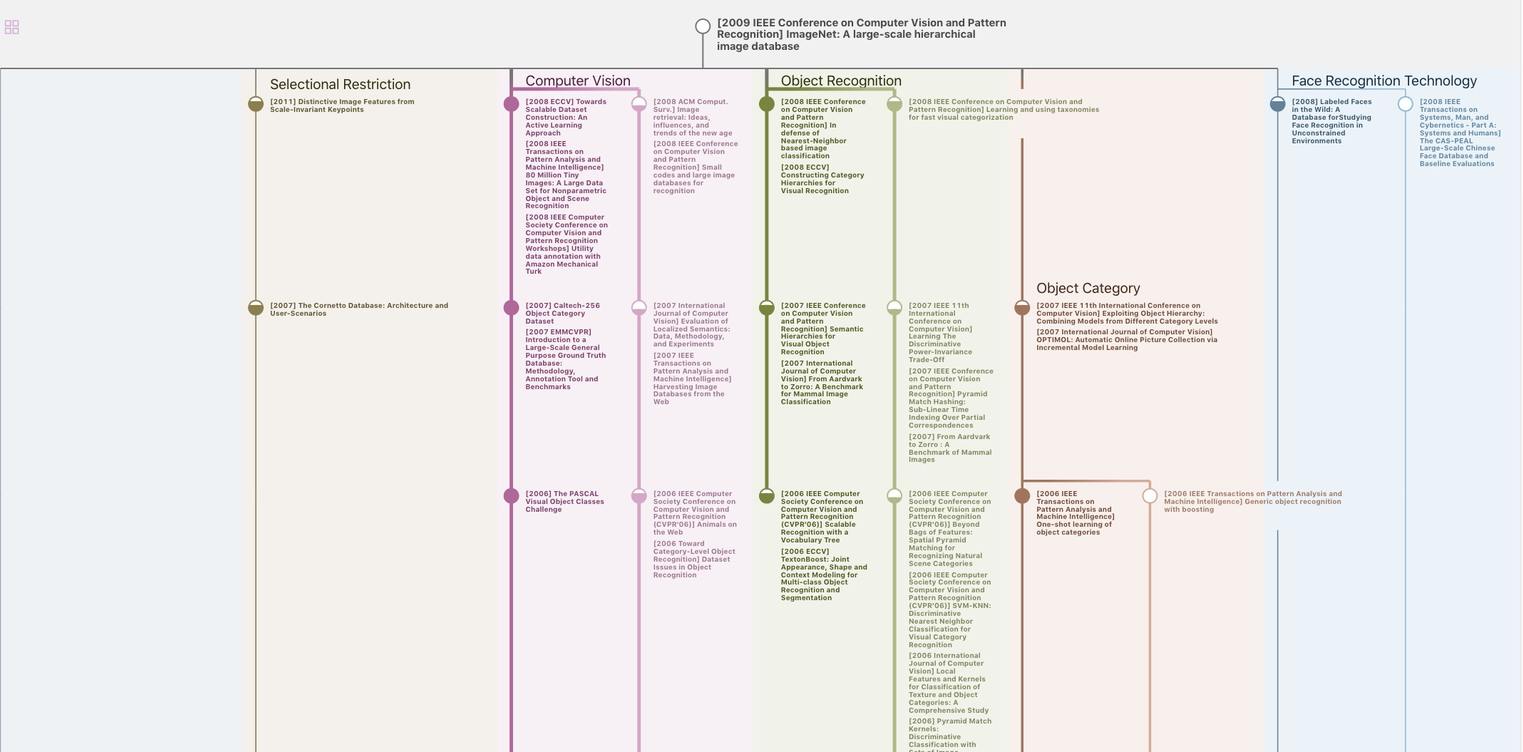
生成溯源树,研究论文发展脉络
Chat Paper
正在生成论文摘要