Machine learning prediction of mild cognitive impairment and its progression to Alzheimer's disease.
Health science reports(2023)
摘要
It is estimated that the number of people with dementia will reach 78 million by 2030 and 139 million by 2050, costing over 2.8 trillion dollars worldwide.1 Effective screening for mild cognitive impairment (MCI) as a risk factor for developing Alzheimer's disease (AD) is a crucial step in helping aging population with their needs.2 Early detection and automated screening for MCI and dementia could offer opportunities for deliberate study and recruitment into trials for developing other potentially useful therapeutics or interventions.3-5 Here, we systematically compare multiple automated machine learning (ML) models in predicting MCI and its progression to AD using real-world structured and unstructured electronic health records (EHRs) data. Our objective is to comprehensively evaluate the predictive accuracy, measured by the area under the curve (AUC) of the receiver operating characteristic (ROC), for future MCI and progression to AD based on routine EHR data, among a diverse population of primary care patients aged 65 years or older. This is a retrospective cohort study using Stanford Healthcare data from 1999 to 2022. The use of this data for this study was approved by Stanford's Institutional Review Board. Our data are formatted in the Observational Medical Outcomes Partnership (OMOP) model.6 The cohort consists of 157,804 (MCI and non-MCI) patients, who had at least one primary care visit after reaching the age of 65; with an average age of 73 and 57.7% were females. 15.1% of patients were Asian, 6.4% were Black, 0.2% were American Indian, 0.9% were Native Hawaiian, 64.3% were White, and 13.1% had other/unknown races or declined to state their race. Our study includes two main components: (a) MCI prediction and (b) MCI to AD progression prediction. We extracted 531,387 primary care visits (for all 157,804 patients in our cohort; each patient has multiple visits) where the patients were at least 65 years old at the time of their appointment. All historical EHR records, including diagnoses, prescriptions, procedures, and clinical notes before the primary care visits, were extracted. Note clinical note features are pre-processed and extracted in the form of standardized SNOMED structure concepts from patients' notes as part of OMOP data model.7 The OMOP Common Data Model standardizes healthcare data for research. By standardizing the representation of patient information and healthcare data elements, OMOP enables researchers to produce reliable evidence, conduct large-scale and multisite studies, and develop predictive models using data from multiple institutions, enhancing our understanding of health outcomes and treatment effectiveness. MCI prediction component was created using supervised ML models including logistic regression,8 random forest,9 and xgboost10 to predict MCI diagnosis within 1 year of primary care visit and using 480 predictors extracted from structured and unstructured EHR data. Models were trained using data in or before 2019 and tested using data in 2020 and after. The second component, MCI to AD progression prediction model, was trained using 7425 MCI patients' data and 373 predictors extracted from structured and unstructured EHR data before MCI onset. Further, we analyzed and presented possible risk factors for progression from MCI to AD in our data. Table 1 shows the MCI and MCI to AD progression prediction results. Random forest was the best-performing model in predicting MCI onset as well as predicting its progression to AD. Additionally, we utilized age-stratified test data to evaluate the performance of our models. We divided our test data sets into distinct age groups (65–74, 75–85, and 85+ years old), and tested our models separately on each age group. For MCI prediction, the random forest model outperformed the other models in the age groups of 65–74 (ROC-AUC = 64.3 ± $\,\pm \,$ 1.2), 75–84 (ROC-AUC = 60.6 ± $\,\pm \,$ 1.4), and 85 years and older (ROC-AUC = 60.8 ± $\,\pm \,$ 2.2). Similarly, in MCI to AD progression prediction, the random forest model exhibited the highest ROC-AUC compared to all other models in the age groups of 65–74 (62.4 ± $\,\pm \,$ 4.1), 75–84 (58.2 ± $\,\pm \,$ 1.9), and 85 years and older (62.0 ± $\,\pm \,$ 3.6). This approach allowed us to examine the effectiveness of our models in different age cohorts, providing insights into potential age-related variations in model performance. The utilization of age-stratified data in our analysis enhances the robustness and generalizability of our findings, as it accounts for potential age-related differences and enables a more nuanced understanding of our ML model's performance. MCI to AD <1 year MCI to AD <2 years MCI to AD <3 years MCI to AD <4 years MCI to AD <5 years Table 2 shows the top 10 variables significantly associated with the progression from MCI to AD. The majority of these variables are the predictors extracted from patients' clinical notes. Variables related to mental health disorder diagnosis and more memory loss-related concepts in patients' clinical notes are among the top variables that are predictive of progression to AD. Given the complex nature of MCI and AD and sparsity of these events, especially at a visit-based level, random forest can detect MCI and progression to AD reasonably well. Our results also showed that clinical notes include signals that provide increased power in discriminating MCI patients who progressed to AD from MCI patients with no further AD diagnosis. Results illustrate that it is possible to predict MCI onset and AD progression with moderate levels of discrimination accuracy. This suggests an opportunity for population-wide screening mechanisms to identify patients at potential risk, who could then undergo more specific confirmatory evaluation to consider early treatment or recruitment into clinical trials. Novel elements here include the use of extracted clinical note elements that are typically underutilized in clinical risk models, which further illustrate some of the key documented features that are predictive of such important conditions. Expected effects and utilization of this study include an automated tool for primary care providers and specialists for early detection of ADs. Automated multifactor models demonstrated superior predictive ability in assessing the risk of dementia.11 Despite the current scarcity of clinical interventions with proven efficacy in altering the progression of MCI and dementia, the identification of individuals at risk can facilitate targeted recruitment into clinical trials, enabling the study of emerging interventions that may demonstrate effectiveness in the early stages of the disease. Furthermore, the acquisition and dissemination of personalized diagnostic evaluation strategies provide an immediately applicable approach to enhance the timely diagnostic assessment of MCI cases, enhance therapeutic approaches to postpone the AD onset,12-14 improve care or socioeconomic factors15, 16 for the patients at risk, and facilitate the prompt identification of potentially reversible factors such as endocrine, nutritional, and infectious causes. Note this study is limited as a single-site study; however, the models can be applied to any other site with OMOP data model. The proposed models serve as decision support systems that should be utilized under the supervision of trained healthcare providers, including primary care providers and specialists. Although the proposed ML models may not be as accurate as deliberate diagnostics such as MOCA, they are able to evaluate population-wide automatically through data systems without requiring deliberate in-person evaluation of everyone. Sajjad Fouladvand: Conceptualization; data curation; formal analysis; methodology; project administration; validation; writing—original draft. Morteza Noshad: Conceptualization; methodology; writing—review and editing. V. J. Periyakoil: Conceptualization; funding acquisition; methodology; supervision; writing—review and editing. Jonathan H. Chen: Conceptualization; funding acquisition; methodology; supervision; writing—review and editing. This research (study design, interpretation of data and writing of the report) was supported in part by Stanford Aging and Ethnogeriatrics (SAGE) Research Center under NIH/NIA Grant P30AG059307 and NIH/National Library of Medicine via Award R56LM013365. This research used data or services provided by STARR, Sanford medicine Research data Repository. Sajjad Fouladvand has received consulting fees from Roche, a multinational company with two primary divisions: Pharmaceuticals and Diagnostics. VJ Periyakoil declared no conflict of interest. Morteza Noshad is a cofounder of Shyld AI and a scientist at Vida Health. Jonathan H. Chen reported receiving grants from the NIH/National Institute on Drug Abuse Clinical Trials Network (UG1DA015815–CTN-0136), Stanford Artificial Intelligence in Medicine and Imaging–Human-Centered Artificial Intelligence Partnership Grant, Doris Duke Charitable Foundation—Covid-19 Fund to Retain Clinical Scientists (20211260), Google Inc (in a research collaboration to leverage health data to predict clinical outcomes), and the American Heart Association—Strategically Focused Research Network—Diversity in Clinical Trials. The lead author Sajjad Fouladvand, Sajjad Fouladvand affirms that this manuscript is an honest, accurate, and transparent account of the study being reported; that no important aspects of the study have been omitted; and that any discrepancies from the study as planned (and, if relevant, registered) have been explained. Due to confidentiality restrictions, the data used in this study are not available for public access or sharing.
更多查看译文
关键词
mild cognitive impairment,alzheimer,machine learning,prediction
AI 理解论文
溯源树
样例
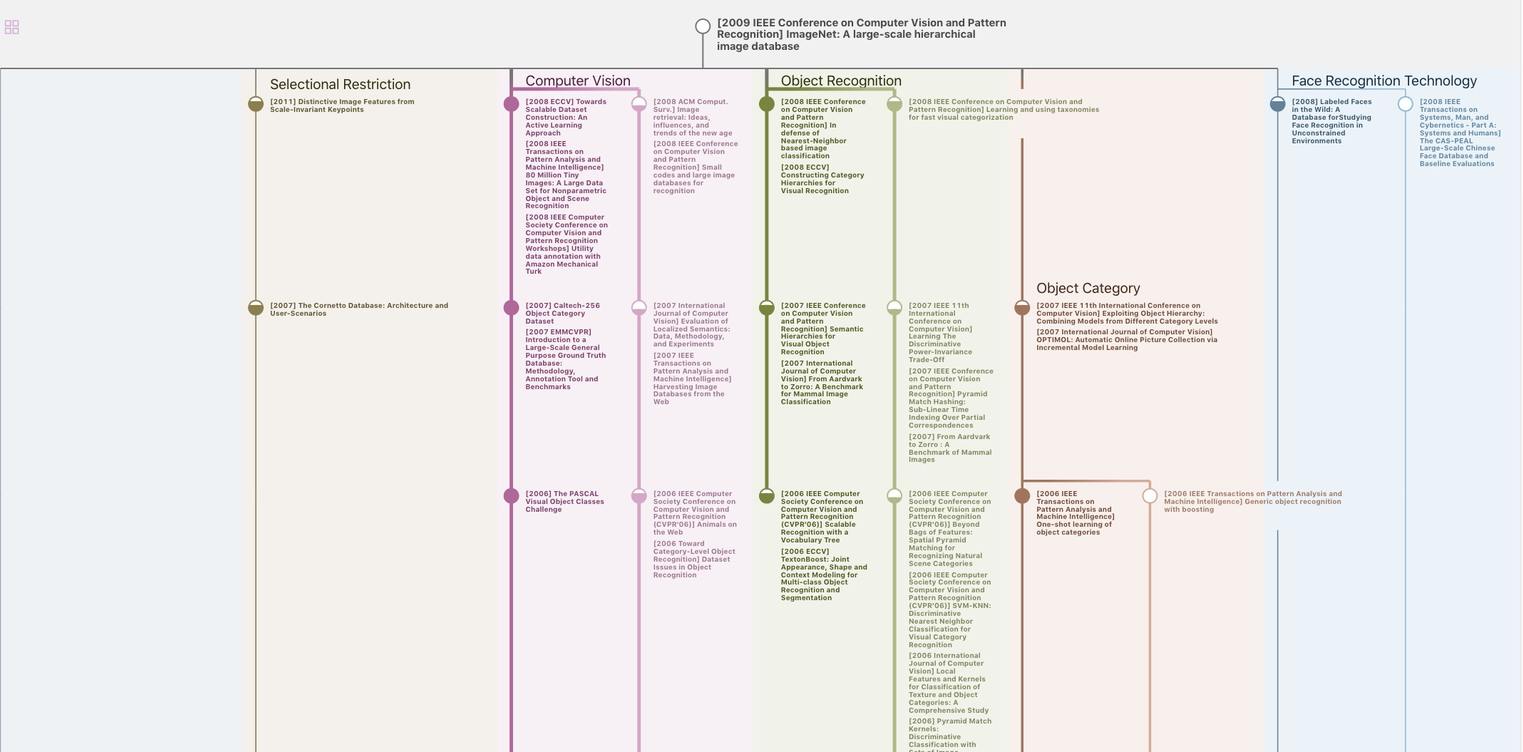
生成溯源树,研究论文发展脉络
Chat Paper
正在生成论文摘要