DespNet: A residual learning based deep convolutional neural network for the despeckling of optical coherence tomography images
MULTIMEDIA TOOLS AND APPLICATIONS(2023)
摘要
OCT (Optical Coherence Tomography) is a non-invasive diagnostic tool for detecting and treating a wide range of retinal diseases. However, the OCT image formation method produces speckle noise, degrading the quality of OCT images significantly, and these low-quality images negatively impact subsequent illness diagnosis. Traditional approaches to remove speckle noise include spatial/transform domain filtering, dictionary learning, or hybridizing these methods. By adopting a hierarchical network topology, deep Convolutional Neural Networks (CNN) have expanded the capacity to harness spatial correlations and extract data at multiple resolutions, making image denoising algorithms more robust. This paper proposes a residual learning-based despeckling CNN architecture (DespNet) for removing speckle noise from OCT images. Trained on 1440 augmented OCT images, DespNet generates the residual images that contain the detailed noise pattern of input images, which, when subtracted from noisy images, results in the denoised version. Quantitative and qualitative analyses have been done, and the experimental results show that the images despeckled using DespNet architecture substantially reduce speckle noise while preserving texture and structure that aids in retinal layer segmentation and consequent illness diagnosis.
更多查看译文
关键词
Optical coherence tomography,Speckle noise,Convolutional neural networks,Residual learning,Batch normalisation,Image denoising
AI 理解论文
溯源树
样例
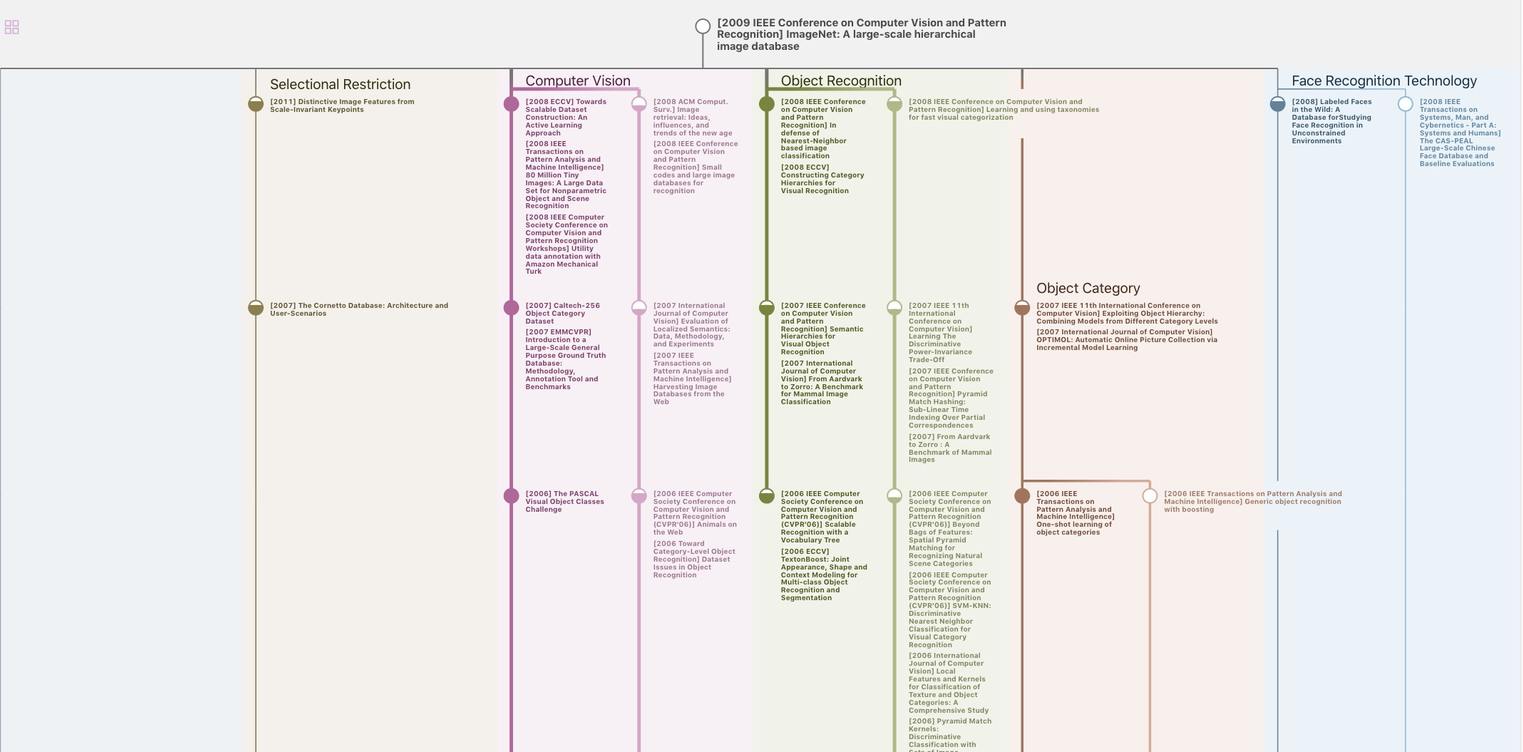
生成溯源树,研究论文发展脉络
Chat Paper
正在生成论文摘要