A projected decentralized variance-reduction algorithm for constrained optimization problems
Neural Computing and Applications(2024)
摘要
Solving constrained optimization problems that require processing large-scale data is of significant value in practical applications, and such problems can be described as the minimization of a finite-sum of local convex functions. Many existing works addressing constrained optimization problems have achieved a linear convergence rate to the exact optimal solution if the constant step-size was sufficiently small. However, they still suffer from low computational efficiency because of the computation of the local batch gradients at each iteration. Considering high computational efficiency to resolve the constrained optimization problems, we introduce the projection operator and variance-reduction technique to propose a novel projected decentralized variance-reduction algorithm, namely P-DVR, to tackle the constrained optimization problem subject to a closed convex set. Theoretical analysis shows that if the local function is strongly convex and smooth, the P-DVR algorithm can converge to the exact optimal solution at a linear convergence rate 𝒪 ( λ̂^k ) with a sufficiently small step-size, where 0< λ̂ < 1 . Finally, we experimentally validate the effectiveness of the algorithm, i.e., the algorithm possesses high computational efficiency and exact convergence.
更多查看译文
关键词
Decentralized constrained optimization,Variance-reduction,Linear convergence,Stochastic algorithm.
AI 理解论文
溯源树
样例
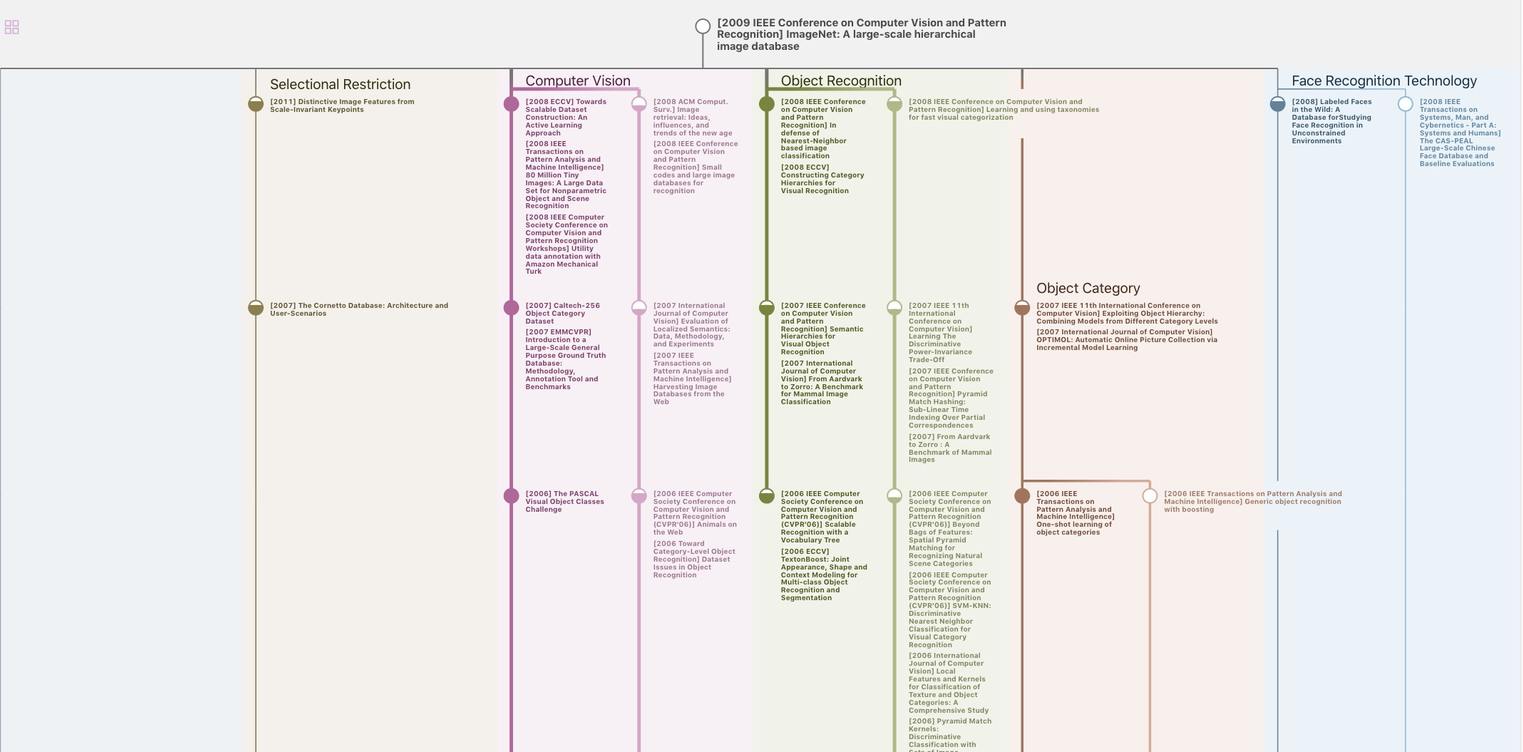
生成溯源树,研究论文发展脉络
Chat Paper
正在生成论文摘要