FD-Align: Feature Discrimination Alignment for Fine-tuning Pre-Trained Models in Few-Shot Learning
NeurIPS(2023)
摘要
Due to the limited availability of data, existing few-shot learning methods trained from scratch fail to achieve satisfactory performance. In contrast, large-scale pre-trained models such as CLIP demonstrate remarkable few-shot and zero-shot capabilities. To enhance the performance of pre-trained models for downstream tasks, fine-tuning the model on downstream data is frequently necessary. However, fine-tuning the pre-trained model leads to a decrease in its generalizability in the presence of distribution shift, while the limited number of samples in few-shot learning makes the model highly susceptible to overfitting. Consequently, existing methods for fine-tuning few-shot learning primarily focus on fine-tuning the model's classification head or introducing additional structure. In this paper, we introduce a fine-tuning approach termed Feature Discrimination Alignment (FD-Align). Our method aims to bolster the model's generalizability by preserving the consistency of spurious features across the fine-tuning process. Extensive experimental results validate the efficacy of our approach for both ID and OOD tasks. Once fine-tuned, the model can seamlessly integrate with existing methods, leading to performance improvements. Our code can be found in https://github.com/skingorz/FD-Align.
更多查看译文
关键词
feature discrimination alignment,learning,models,fd-align,fine-tuning,pre-trained,few-shot
AI 理解论文
溯源树
样例
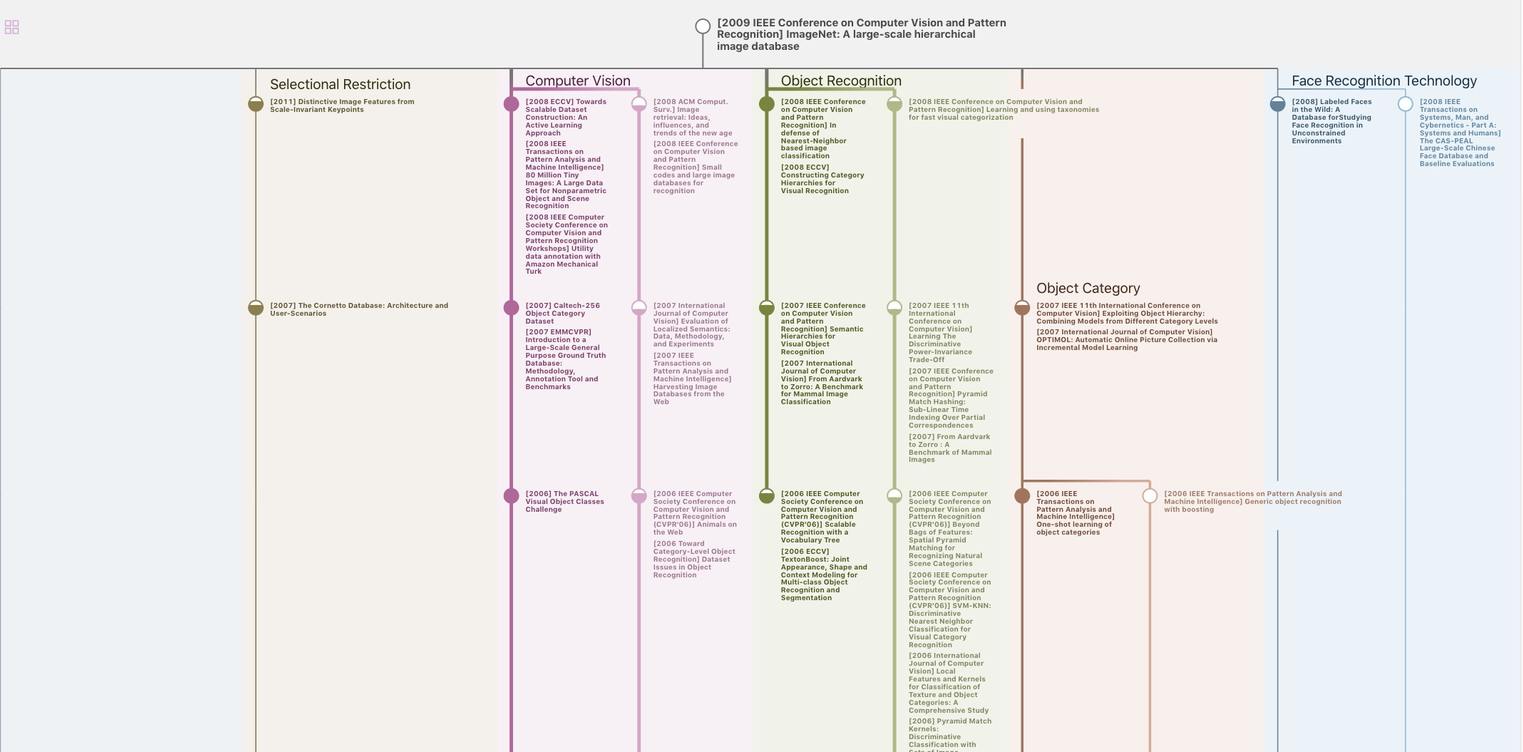
生成溯源树,研究论文发展脉络
Chat Paper
正在生成论文摘要