SLOG: A Structural Generalization Benchmark for Semantic Parsing.
CoRR(2023)
摘要
The goal of compositional generalization benchmarks is to evaluate how well models generalize to new complex linguistic expressions. Existing benchmarks often focus on lexical generalization, the interpretation of novel lexical items in syntactic structures familiar from training; structural generalization tasks, where a model needs to interpret syntactic structures that are themselves unfamiliar from training, are often underrepresented, resulting in overly optimistic perceptions of how well models can generalize. We introduce SLOG, a semantic parsing dataset that extends COGS (Kim and Linzen, 2020) with 17 structural generalization cases. In our experiments, the generalization accuracy of Transformer models, including pretrained ones, only reaches 40.6%, while a structure-aware parser only achieves 70.8%. These results are far from the near-perfect accuracy existing models achieve on COGS, demonstrating the role of SLOG in foregrounding the large discrepancy between models' lexical and structural generalization capacities.
更多查看译文
关键词
structural generalization benchmark
AI 理解论文
溯源树
样例
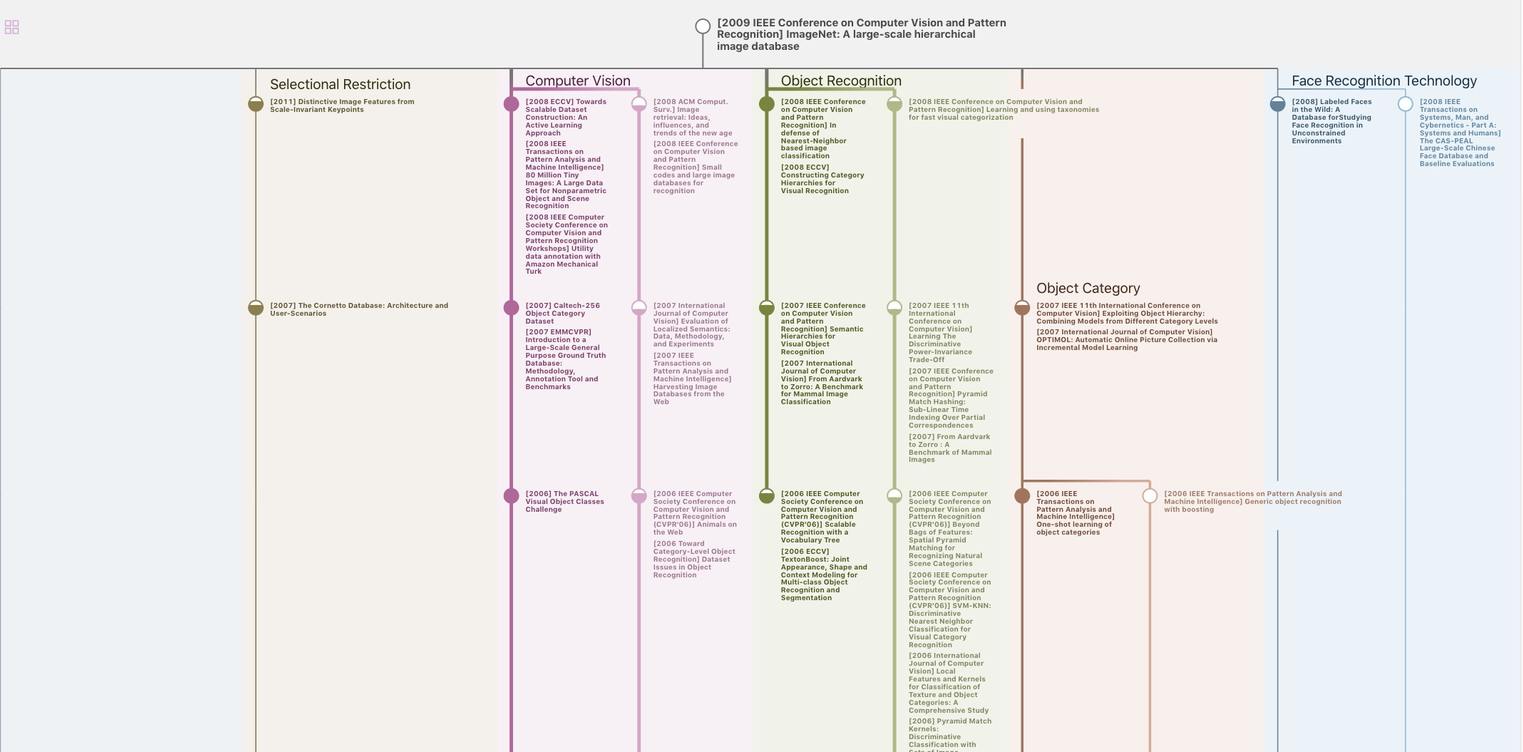
生成溯源树,研究论文发展脉络
Chat Paper
正在生成论文摘要