The Fundamental Dilemma of Bayesian Active Meta-learning
CoRR(2023)
摘要
Many applications involve estimation of parameters that generalize across multiple diverse, but related, data-scarce task environments. Bayesian active meta-learning, a form of sequential optimal experimental design, provides a framework for solving such problems. The active meta-learner's goal is to gain transferable knowledge (estimate the transferable parameters) in the presence of idiosyncratic characteristics of the current task (task-specific parameters). We show that in such a setting, greedy pursuit of this goal can actually hurt estimation of the transferable parameters (induce so-called negative transfer). The learner faces a dilemma akin to but distinct from the exploration--exploitation dilemma: should they spend their acquisition budget pursuing transferable knowledge, or identifying the current task-specific parameters? We show theoretically that some tasks pose an inevitable and arbitrarily large threat of negative transfer, and that task identification is critical to reducing this threat. Our results generalize to analysis of prior misspecification over nuisance parameters. Finally, we empirically illustrate circumstances that lead to negative transfer.
更多查看译文
关键词
active,meta-learning
AI 理解论文
溯源树
样例
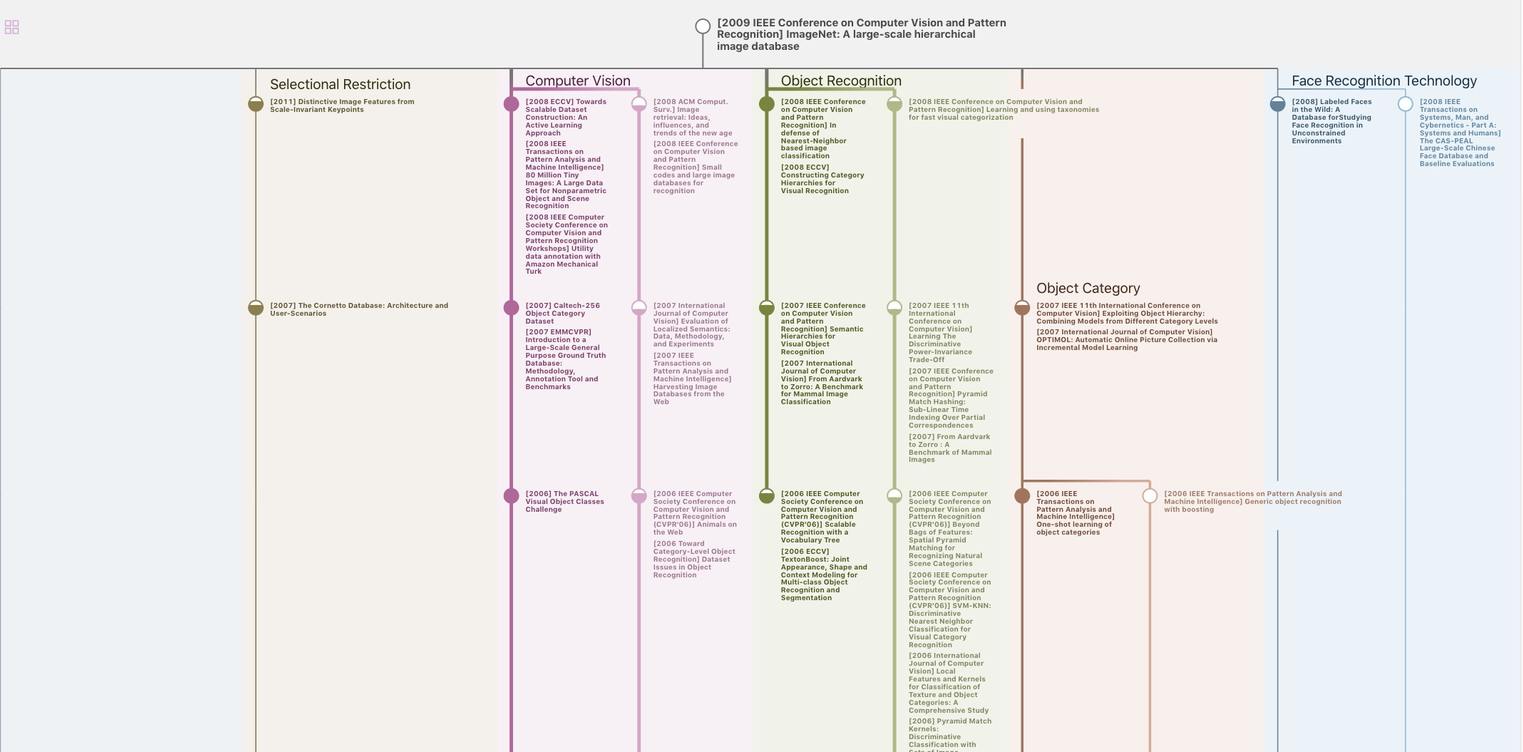
生成溯源树,研究论文发展脉络
Chat Paper
正在生成论文摘要