CrisisMatch: Semi-Supervised Few-Shot Learning for Fine-Grained Disaster Tweet Classification
CoRR(2023)
摘要
The shared real-time information about natural disasters on social media platforms like Twitter and Facebook plays a critical role in informing volunteers, emergency managers, and response organizations. However, supervised learning models for monitoring disaster events require large amounts of annotated data, making them unrealistic for real-time use in disaster events. To address this challenge, we present a fine-grained disaster tweet classification model under the semi-supervised, few-shot learning setting where only a small number of annotated data is required. Our model, CrisisMatch, effectively classifies tweets into fine-grained classes of interest using few labeled data and large amounts of unlabeled data, mimicking the early stage of a disaster. Through integrating effective semi-supervised learning ideas and incorporating TextMixUp, CrisisMatch achieves performance improvement on two disaster datasets of 11.2\% on average. Further analyses are also provided for the influence of the number of labeled data and out-of-domain results.
更多查看译文
关键词
tweet classification,disaster
AI 理解论文
溯源树
样例
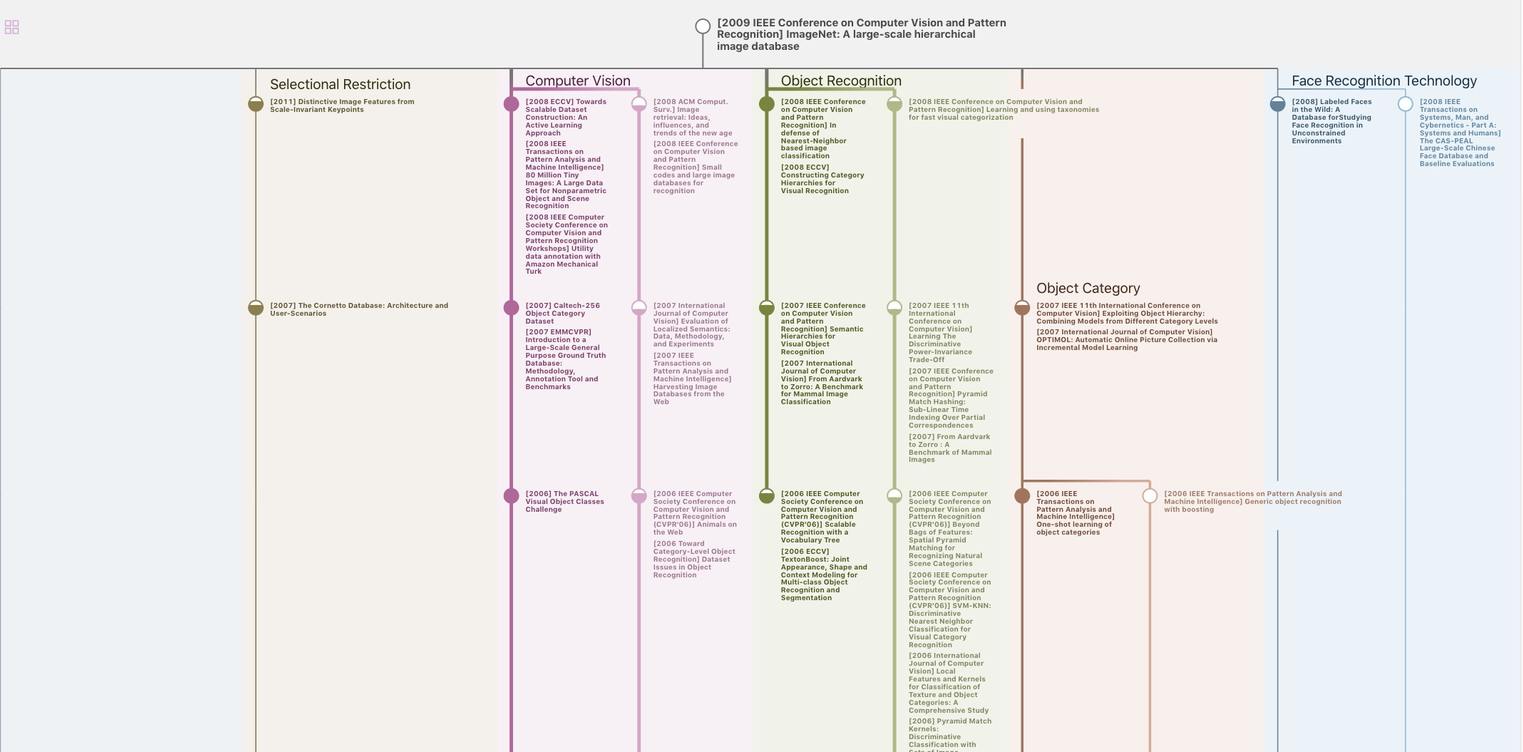
生成溯源树,研究论文发展脉络
Chat Paper
正在生成论文摘要