Prefix-Tuning Based Unsupervised Text Style Transfer.
CoRR(2023)
摘要
Unsupervised text style transfer aims at training a generative model that can alter the style of the input sentence while preserving its content without using any parallel data. In this paper, we employ powerful pre-trained large language models and present a new prefix-tuning-based method for unsupervised text style transfer. We construct three different kinds of prefixes, i.e., \textit{shared prefix, style prefix}, and \textit{content prefix}, to encode task-specific information, target style, and the content information of the input sentence, respectively. Compared to embeddings used by previous works, the proposed prefixes can provide richer information for the model. Furthermore, we adopt a recursive way of using language models in the process of style transfer. This strategy provides a more effective way for the interactions between the input sentence and GPT-2, helps the model construct more informative prefixes, and thus, helps improve the performance. Evaluations on the well-known datasets show that our method outperforms the state-of-the-art baselines. Results, analysis of ablation studies, and subjective evaluations from humans are also provided for a deeper understanding of the proposed method.
更多查看译文
关键词
text,style,prefix-tuning
AI 理解论文
溯源树
样例
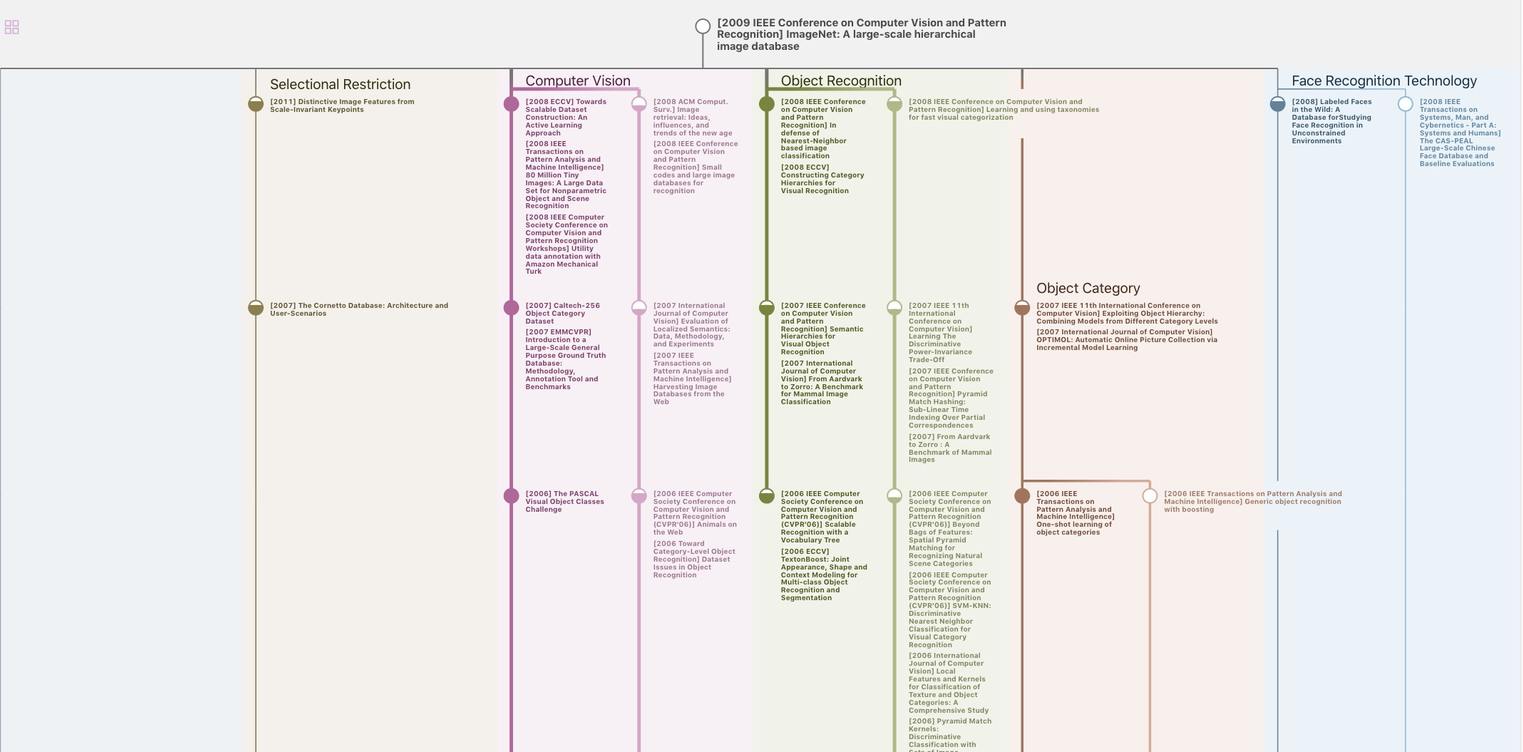
生成溯源树,研究论文发展脉络
Chat Paper
正在生成论文摘要