Modeling groundwater levels in California's Central Valley by hierarchical Gaussian process and neural network regression
CoRR(2023)
摘要
Modeling groundwater levels continuously across California's Central Valley (CV) hydrological system is challenging due to low-quality well data which is sparsely and noisily sampled across time and space. A novel machine learning method is proposed for modeling groundwater levels by learning from a 3D lithological texture model of the CV aquifer. The proposed formulation performs multivariate regression by combining Gaussian processes (GP) and deep neural networks (DNN). Proposed hierarchical modeling approach constitutes training the DNN to learn a lithologically informed latent space where non-parametric regression with GP is performed. The methodology is applied for modeling groundwater levels across the CV during 2015 - 2020. We demonstrate the efficacy of GP-DNN regression for modeling non-stationary features in the well data with fast and reliable uncertainty quantification. Our results indicate that the 2017 and 2019 wet years in California were largely ineffective in replenishing the groundwater loss caused during previous drought years.
更多查看译文
关键词
hierarchical gaussian process,groundwater levels,neural network,central valley
AI 理解论文
溯源树
样例
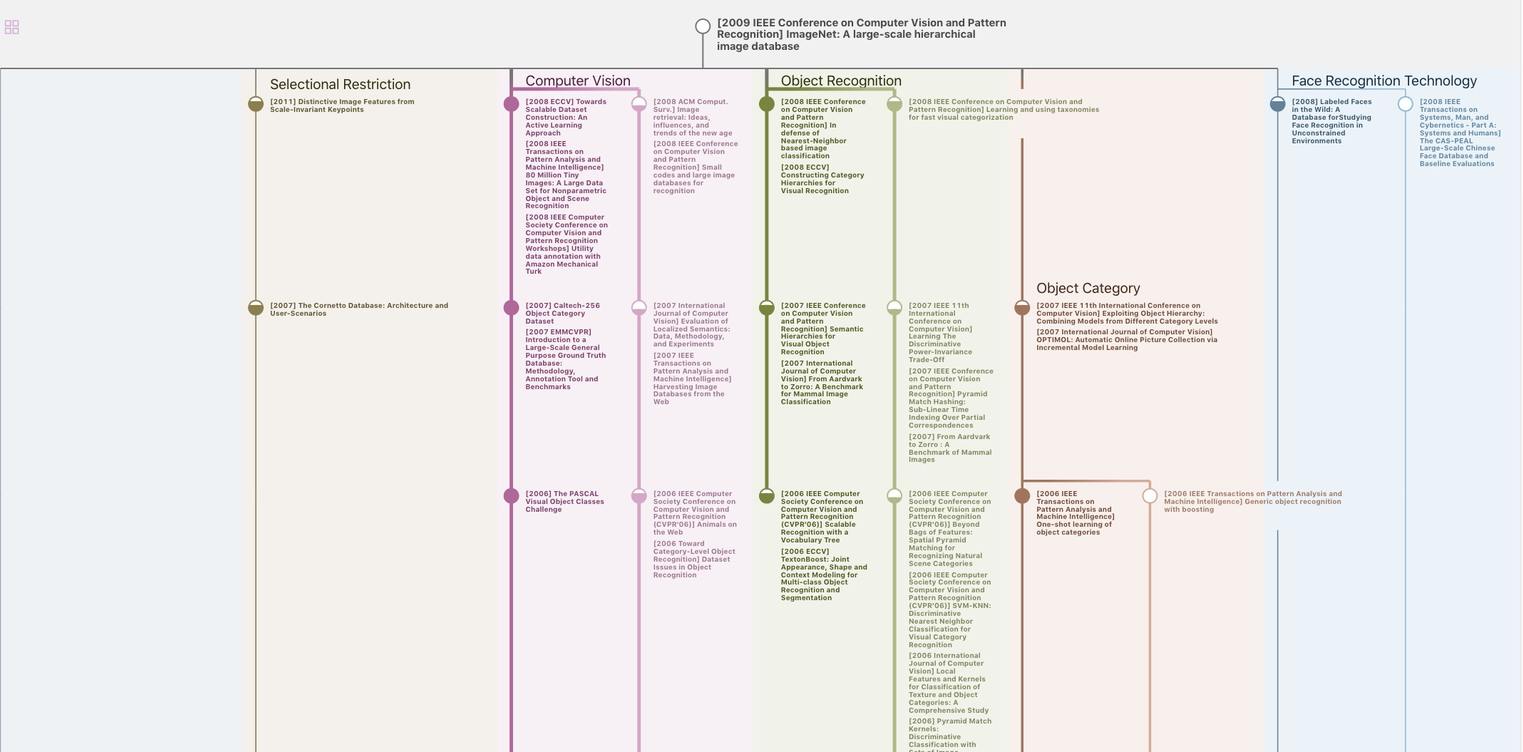
生成溯源树,研究论文发展脉络
Chat Paper
正在生成论文摘要