Evaluating Spatial Understanding of Large Language Models
CoRR(2023)
摘要
Large language models (LLMs) show remarkable capabilities across a variety of tasks. Despite the models only seeing text in training, several recent studies suggest that LLM representations implicitly capture aspects of the underlying grounded concepts. Here, we explore LLM representations of a particularly salient kind of grounded knowledge -- spatial relationships. We design natural-language navigation tasks and evaluate the ability of LLMs, in particular GPT-3.5-turbo, GPT-4, and Llama2 series models, to represent and reason about spatial structures, and compare these abilities to human performance on the same tasks. These tasks reveal substantial variability in LLM performance across different spatial structures, including square, hexagonal, and triangular grids, rings, and trees. We also discover that, similar to humans, LLMs utilize object names as landmarks for maintaining spatial maps. Finally, in extensive error analysis, we find that LLMs' mistakes reflect both spatial and non-spatial factors. These findings suggest that LLMs appear to capture certain aspects of spatial structure implicitly, but room for improvement remains.
更多查看译文
关键词
large language models,language models,spatial understanding
AI 理解论文
溯源树
样例
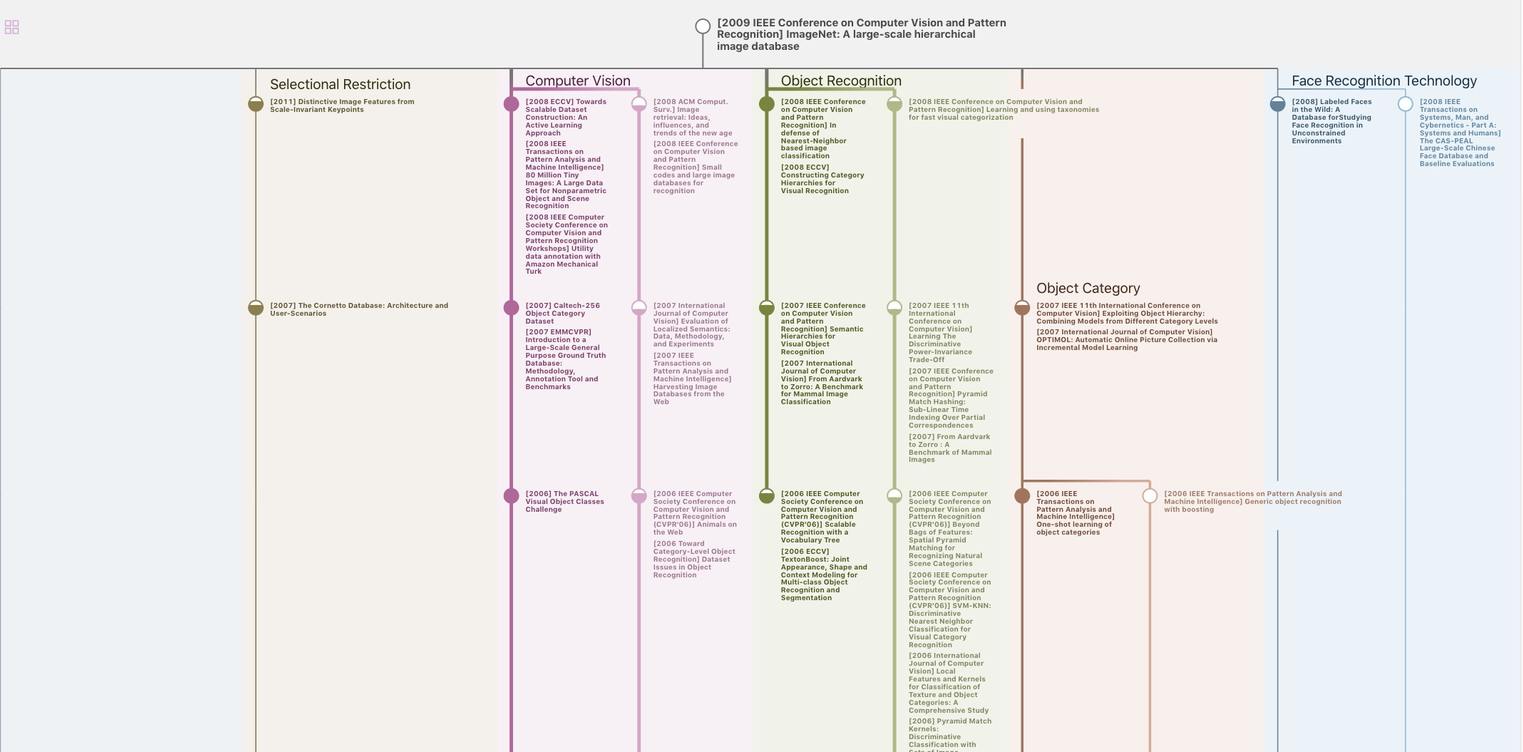
生成溯源树,研究论文发展脉络
Chat Paper
正在生成论文摘要