Label Space Partition Selection for Multi-Object Tracking Using Two-Layer Partitioning
CoRR(2023)
摘要
Estimating the trajectories of multi-objects poses a significant challenge due to data association ambiguity, which leads to a substantial increase in computational requirements. To address such problems, a divide-and-conquer manner has been employed with parallel computation. In this strategy, distinguished objects that have unique labels are grouped based on their statistical dependencies, the intersection of predicted measurements. Several geometry approaches have been used for label grouping since finding all intersected label pairs is clearly infeasible for large-scale tracking problems. This paper proposes an efficient implementation of label grouping for label-partitioned generalized labeled multi-Bernoulli filter framework using a secondary partitioning technique. This allows for parallel computation in the label graph indexing step, avoiding generating and eliminating duplicate comparisons. Additionally, we compare the performance of the proposed technique with several efficient spatial searching algorithms. The results demonstrate the superior performance of the proposed approach on large-scale data sets, enabling scalable trajectory estimation.
更多查看译文
关键词
Multi-object tracking,Random finite set,Generalized labeled multi-Bernoulli,Label grouping
AI 理解论文
溯源树
样例
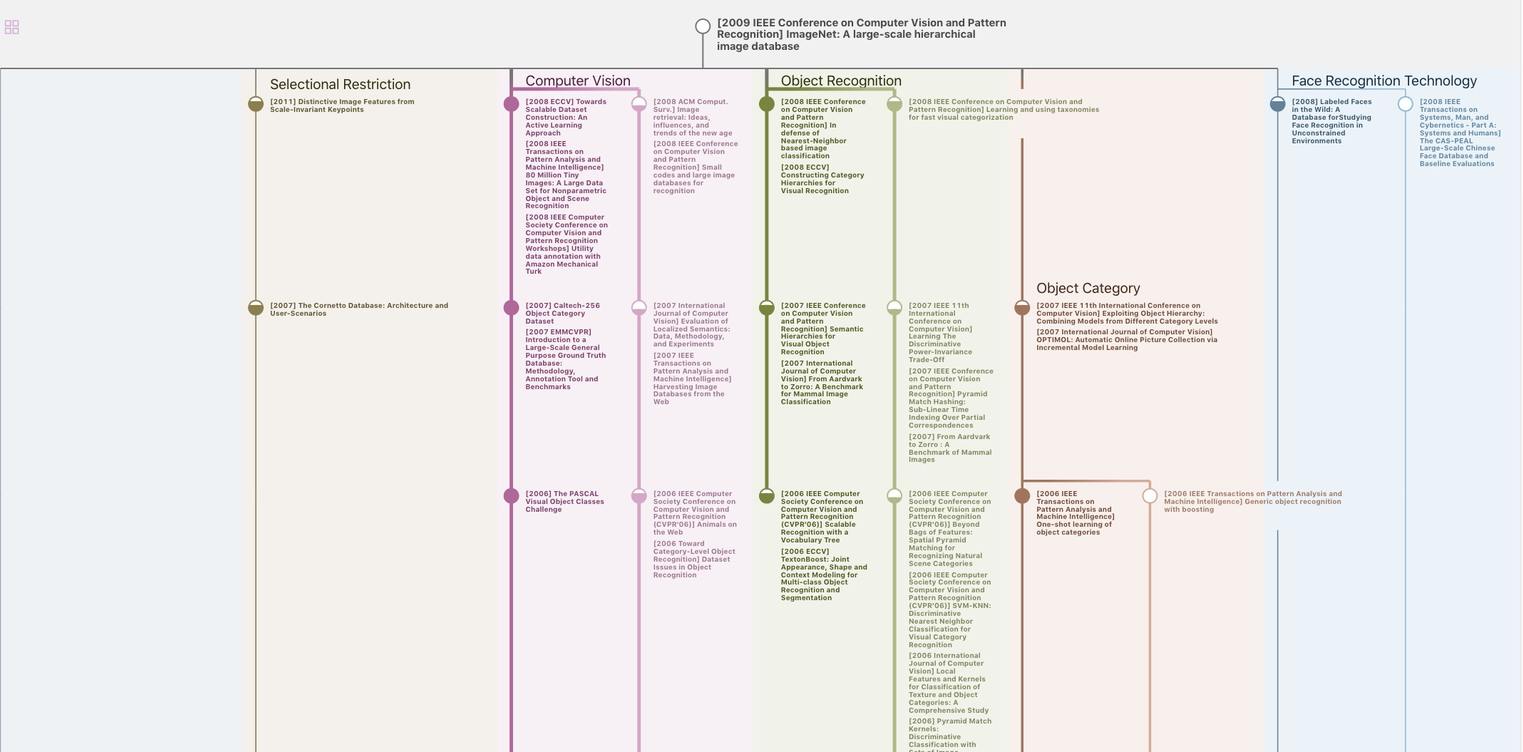
生成溯源树,研究论文发展脉络
Chat Paper
正在生成论文摘要