Multi-fidelity Deep Learning-based methodology for epistemic uncertainty quantification of turbulence models
arXiv (Cornell University)(2023)
摘要
Computational Fluid Dynamics (CFD) simulations using turbulence models are commonly used in engineering design. Of the different turbulence modeling approaches that are available, eddy viscosity based models are the most common for their computational economy. Eddy viscosity based models utilize many simplifications for this economy such as the gradient diffusion and the isotropic eddy viscosity hypotheses. These simplifications limit the degree to which eddy viscosity models can replicate turbulence physics and lead to model form uncertainty. The Eigenspace Perturbation Method (EPM) has been developed for purely physics based estimates of this model form uncertainty in turbulence model predictions. Due to its physics based nature, the EPM weighs all physically possible outcomes equally leading to overly conservative uncertainty estimates in many cases. In this investigation we use data driven Machine Learning (ML) approaches to address this limitation. Using ML models, we can weigh the physically possible outcomes by their likelihood leading to better calibration of the uncertainty estimates. Specifically, we use ML models to predict the degree of perturbations in the EPM over the flow domain. This work focuses on a Convolutional Neural Network (CNN) based model to learn the discrepancy between Reynolds Averaged Navier Stokes (RANS) and Direct Numerical Simulation (DNS) predictions. This model acts as a marker function, modulating the degree of perturbations in the EPM. We show that this physics constrained machine learning framework performs better than the purely physics or purely ML alternatives, and leads to less conservative uncertainty bounds with improved calibration.
更多查看译文
关键词
epistemic uncertainty
AI 理解论文
溯源树
样例
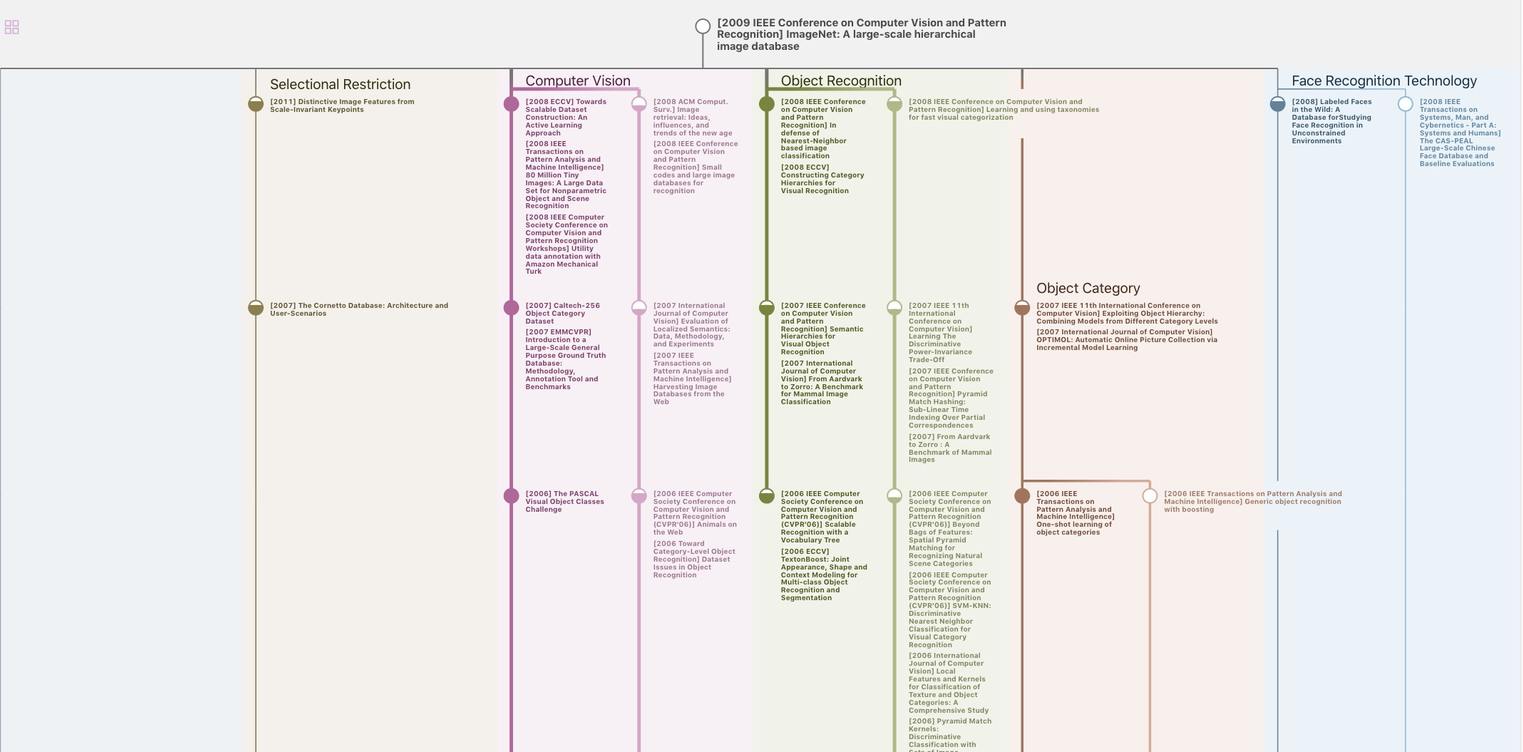
生成溯源树,研究论文发展脉络
Chat Paper
正在生成论文摘要