First-Shot Unsupervised Anomalous Sound Detection With Unknown Anomalies Estimated by Metadata-Assisted Audio Generation
ICASSP 2024 - 2024 IEEE International Conference on Acoustics, Speech and Signal Processing (ICASSP)(2023)
摘要
First-shot (FS) unsupervised anomalous sound detection (ASD) is a brand-new
task introduced in DCASE 2023 Challenge Task 2, where the anomalous sounds for
the target machine types are unseen in training. Existing methods often rely on
the availability of normal and abnormal sound data from the target machines.
However, due to the lack of anomalous sound data for the target machine types,
it becomes challenging when adapting the existing ASD methods to the first-shot
task. In this paper, we propose a new framework for the first-shot unsupervised
ASD, where metadata-assisted audio generation is used to estimate unknown
anomalies, by utilising the available machine information (i.e., metadata and
sound data) to fine-tune a text-to-audio generation model for generating the
anomalous sounds that contain unique acoustic characteristics accounting for
each different machine type. We then use the method of Time-Weighted Frequency
domain audio Representation with Gaussian Mixture Model (TWFR-GMM) as the
backbone to achieve the first-shot unsupervised ASD. Our proposed FS-TWFR-GMM
method achieves competitive performance amongst top systems in DCASE 2023
Challenge Task 2, while requiring only 1
validated in our experiments.
更多查看译文
关键词
Unsupervised learning,anomalous sound detection,audio generation,metadata,latent diffusion model
AI 理解论文
溯源树
样例
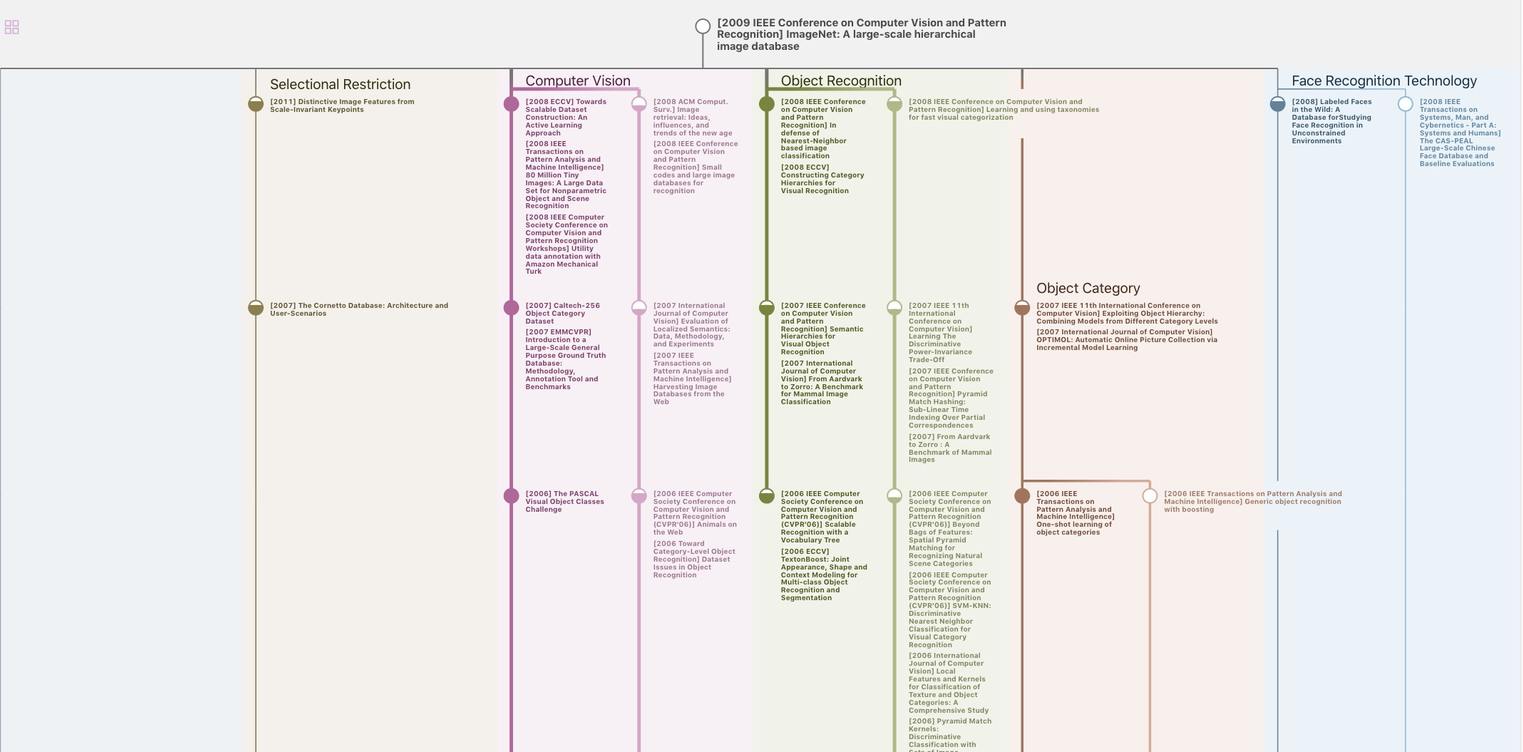
生成溯源树,研究论文发展脉络
Chat Paper
正在生成论文摘要