Distributionally Robust Optimization with Bias and Variance Reduction
CoRR(2023)
摘要
We consider the distributionally robust optimization (DRO) problem with spectral risk-based uncertainty set and $f$-divergence penalty. This formulation includes common risk-sensitive learning objectives such as regularized condition value-at-risk (CVaR) and average top-$k$ loss. We present Prospect, a stochastic gradient-based algorithm that only requires tuning a single learning rate hyperparameter, and prove that it enjoys linear convergence for smooth regularized losses. This contrasts with previous algorithms that either require tuning multiple hyperparameters or potentially fail to converge due to biased gradient estimates or inadequate regularization. Empirically, we show that Prospect can converge 2-3$\times$ faster than baselines such as stochastic gradient and stochastic saddle-point methods on distribution shift and fairness benchmarks spanning tabular, vision, and language domains.
更多查看译文
关键词
optimization,bias,variance
AI 理解论文
溯源树
样例
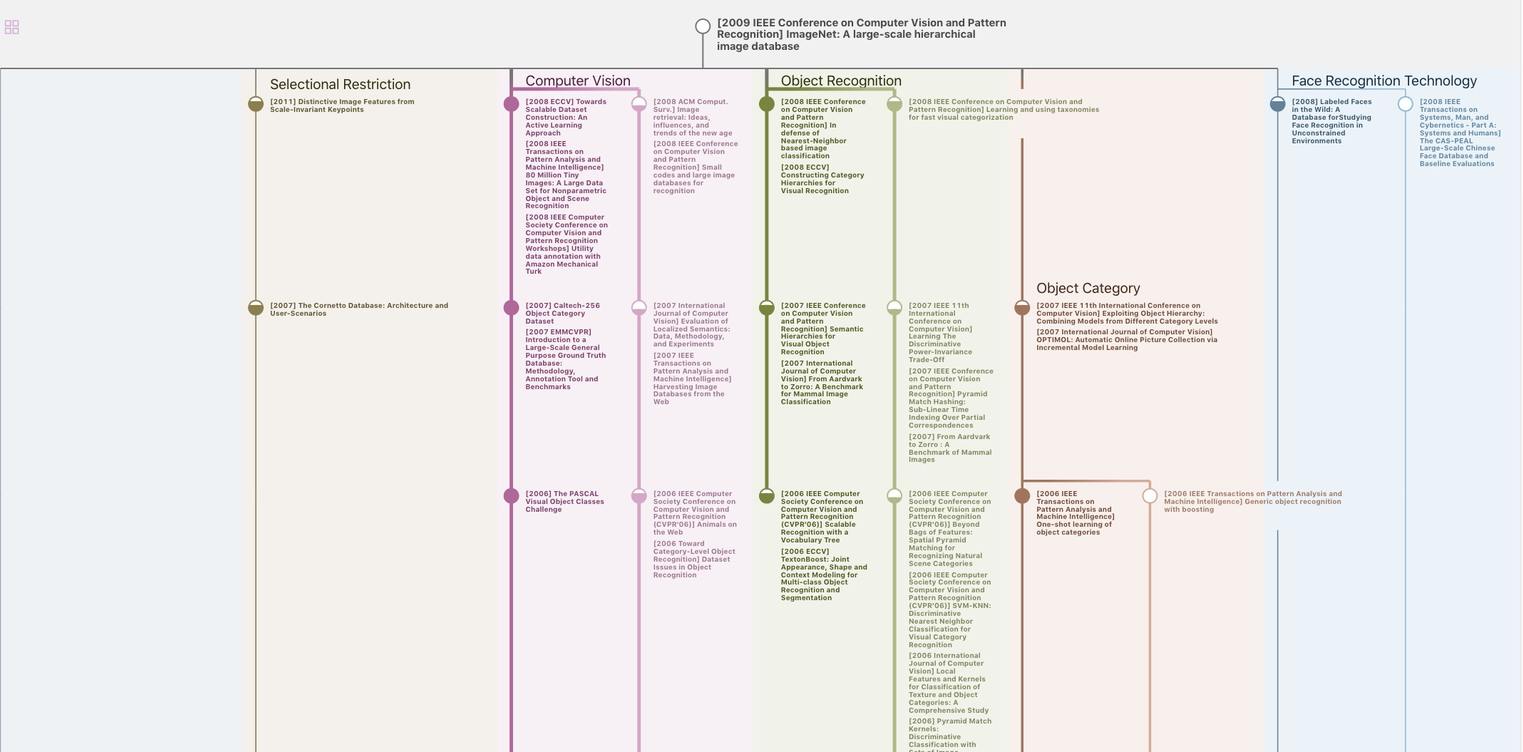
生成溯源树,研究论文发展脉络
Chat Paper
正在生成论文摘要