Cross-modality Multiple Relations Learning for Knowledge-based Visual Question Answering
ACM TRANSACTIONS ON MULTIMEDIA COMPUTING COMMUNICATIONS AND APPLICATIONS(2024)
摘要
Knowledge-based visual question answering not only needs to answer the questions based on images but also incorporates external knowledge to study reasoning in the joint space of vision and language. To bridge the gap between visual content and semantic cues, it is important to capture the question-related and semantics-rich vision-language connections. Most existing solutions model simple intra-modality relation or represent cross-modality relation using a single vector, which makes it difficult to effectively model complex connections between visual features and question features. Thus, we propose a cross-modality multiple relations learning model, aiming to better enrich cross-modality representations and construct advanced multi-modality knowledge triplets. First, we design a simple yet effective method to generate multiple relations that represent the rich cross-modality relations. The various cross-modality relations link the textual question to the related visual objects. These multi-modality triplets efficiently align the visual objects and corresponding textual answers. Second, to encourage multiple relations to better align with different semantic relations, we further formulate a novel global-local loss. The global loss enables the visual objects and corresponding textual answers close to each other through cross-modality relations in the vision-language space, and the local loss better preserves semantic diversity among multiple relations. Experimental results on the Outside Knowledge VQA and Knowledge-Routed Visual Question Reasoning datasets demonstrate that our model outperforms the state-of-the-art methods.
更多查看译文
关键词
Cross-modality relation,external knowledge,visual question answering
AI 理解论文
溯源树
样例
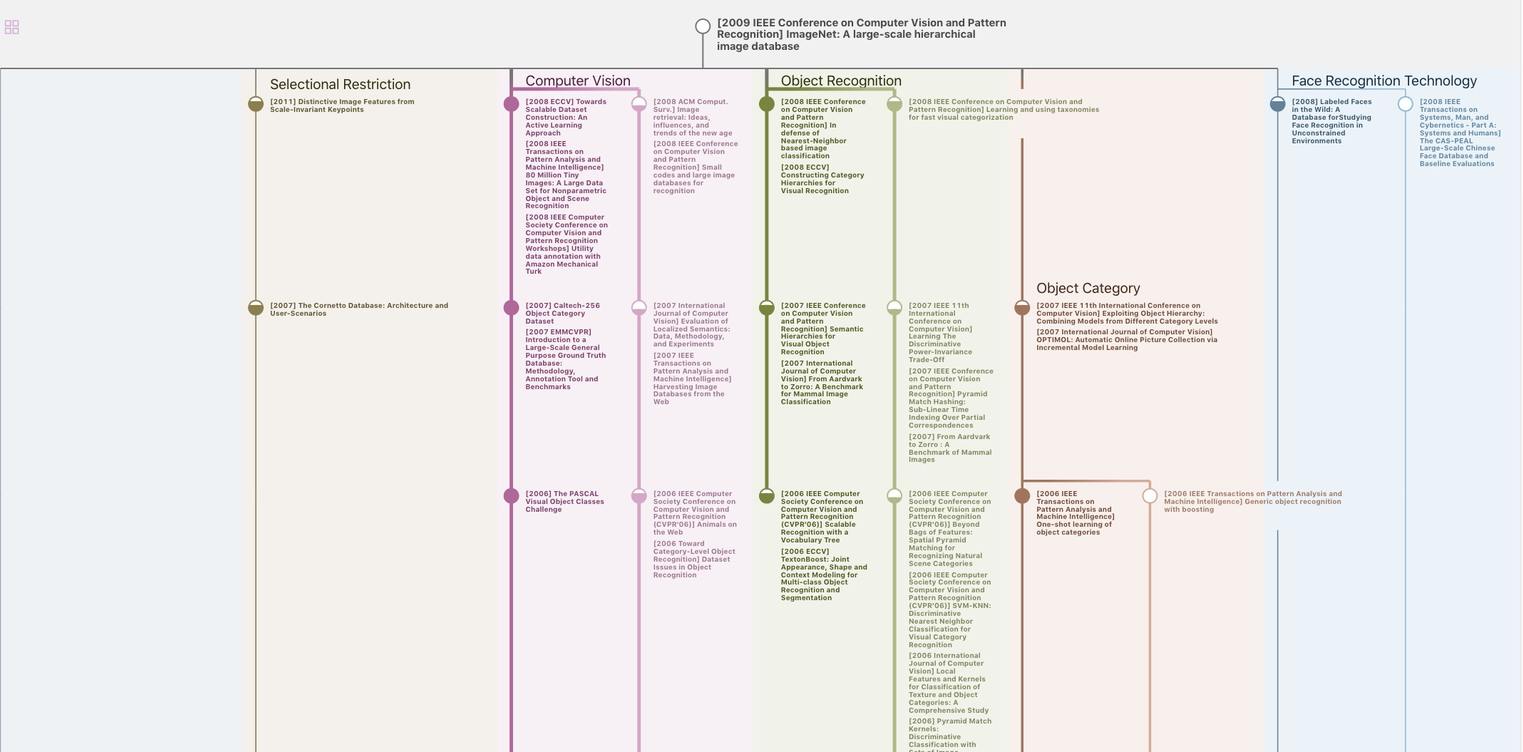
生成溯源树,研究论文发展脉络
Chat Paper
正在生成论文摘要