Understanding the Multi-vector Dense Retrieval Models
PROCEEDINGS OF THE 32ND ACM INTERNATIONAL CONFERENCE ON INFORMATION AND KNOWLEDGE MANAGEMENT, CIKM 2023(2023)
摘要
While dense retrieval has become a promising alternative to the traditional text retrieval models, such as BM25, some recent studies show that multi-vector dense retrieval models are more effective than the single-vector method in retrieval tasks. However, due to a lack of interpretability, why the multi-vector method outperforms its single-vector counterpart has not been fully studied. To fill this research gap, in this work, we investigate and compare the behaviors of single-vector and multi-vector models in retrieval. Specifically, we analyze the vocabulary distribution of dense representations by mapping them back to the sparse, vocabulary space. Our empirical findings show that the multi-vector representation has more lexical overlaps between queries and passages. Additionally, we show that this feature of multi-vector representation can enhance its ranking performance when a given passage can fulfill different information needs and thus can be retrieved by different queries. These results shed light on the internal mechanisms of multi-vector representation and may provide new perspectives for future research.
更多查看译文
关键词
document retrieval,dense retrieval,explainability
AI 理解论文
溯源树
样例
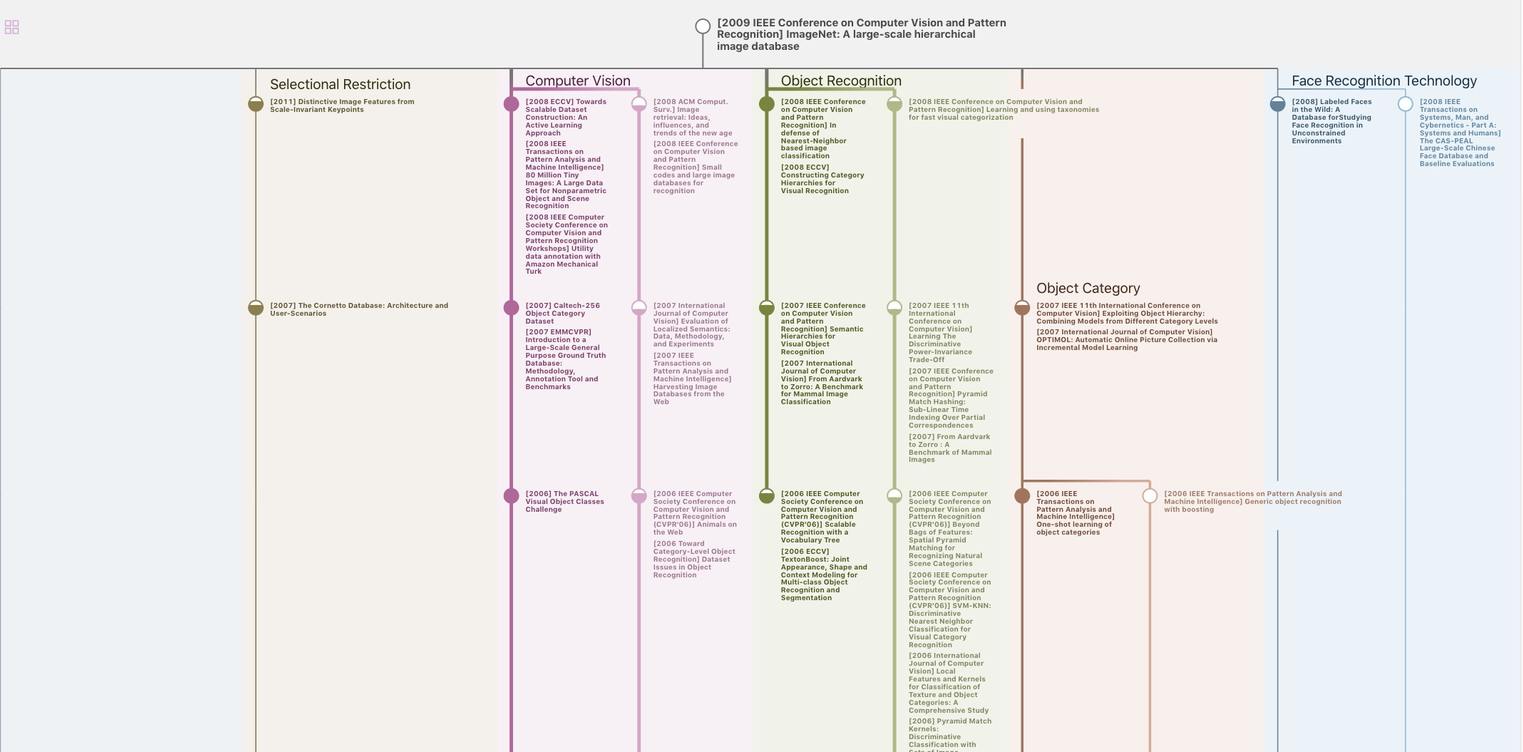
生成溯源树,研究论文发展脉络
Chat Paper
正在生成论文摘要