Counterfactual Adversarial Learning for Recommendation
PROCEEDINGS OF THE 32ND ACM INTERNATIONAL CONFERENCE ON INFORMATION AND KNOWLEDGE MANAGEMENT, CIKM 2023(2023)
摘要
Long-term user responses, i.e., clicks or purchases on e-commerce platforms, are crucial for sequential recommender systems. Recent off-policy evaluation methods involve these responses by simultaneously maximizing expected cumulative rewards. However, two aspects of these methods require further consideration. Firstly, from the system's point of view, candidates with various values are interchangeable, which may result in contradictory future recommendations despite having the same interaction history. Secondly, rewards are manually designed, which necessitates a trial-and-error approach to strike a balance between training stabilization and reward distinction. To address these issues, we propose a new sequential recommender system called NCM4Rec. Specifically, for the distinction problem, NCM4Rec achieves counterfactual consistency via a neural causal model, which is learnable yet equally expressive as classic structural causal models. Such consistency is maintained by a Gumbel-Max design. For the representing problem, NCM4Rec encodes different types of responses as one-hot vectors and captures the long-term preference via adversarial learning. As a consequence, NCM4Rec is both adaptive and identifiable. Both theoretical analyses of the consistency and empirical studies over two real-world datasets demonstrate the effectiveness of our method.
更多查看译文
关键词
sequential recommendations,neural causal models,counterfactual
AI 理解论文
溯源树
样例
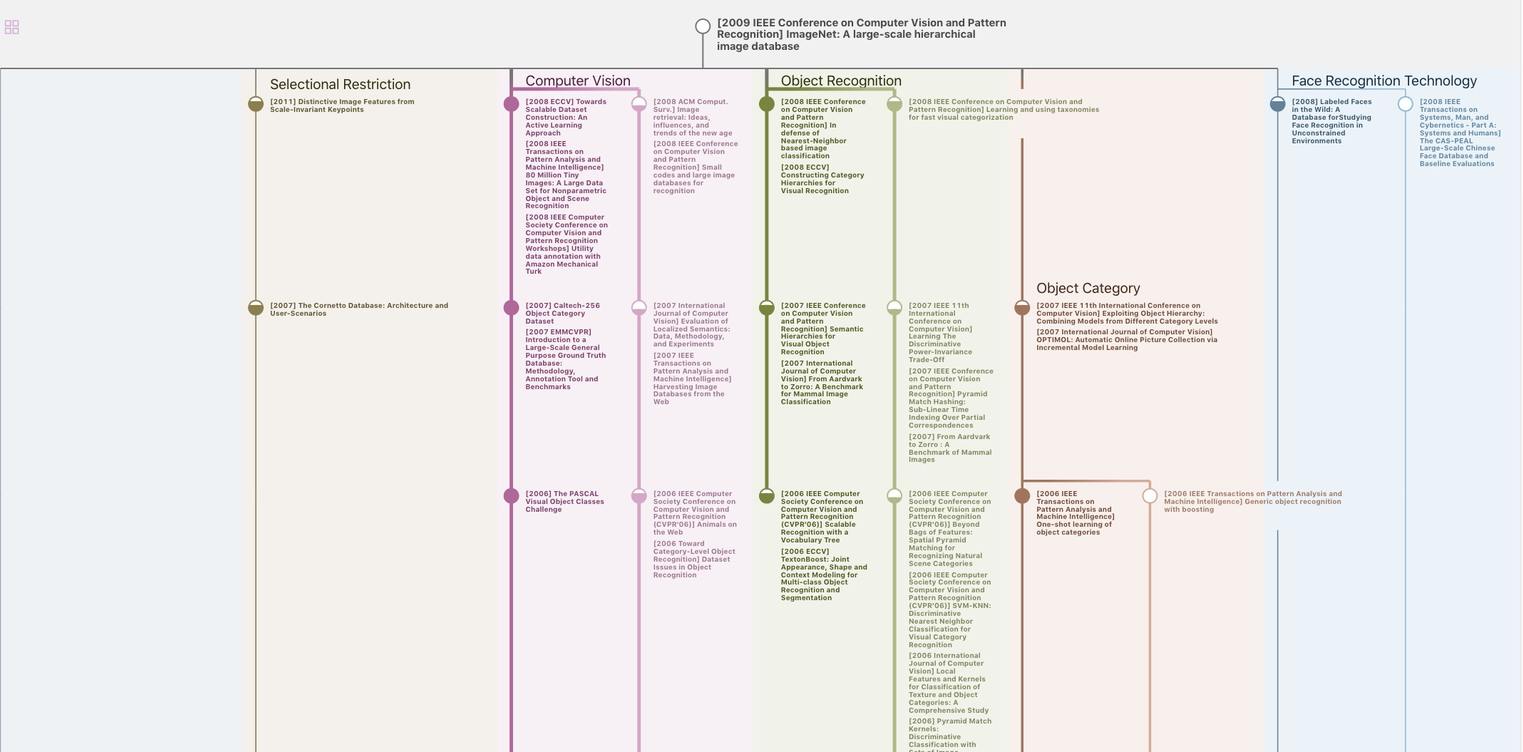
生成溯源树,研究论文发展脉络
Chat Paper
正在生成论文摘要