Graph Learning for Exploratory Query Suggestions in an Instant Search System
PROCEEDINGS OF THE 32ND ACM INTERNATIONAL CONFERENCE ON INFORMATION AND KNOWLEDGE MANAGEMENT, CIKM 2023(2023)
摘要
Search systems in online content platforms are typically biased toward a minority of highly consumed items, reflecting the most common user behavior of navigating toward content that is already popular. Query suggestions are a powerful tool to support query formulation and to encourage exploratory search and discovery. However, classic approaches for query suggestions typically rely either on semantic similarity, which lacks diversity and does not reflect user searching behavior, or on a collaborative similarity measure mined from search logs, which suffers from sparsity and is biased by popular queries. In this work, we argue that the task of query suggestion can be modelled as a link prediction task on a heterogeneous graph including queries and documents, enabling Graph Learning to generate query suggestions encompassing both semantic and collaborative information. We perform an offline evaluation on an internal Spotify dataset of search logs and on two public datasets, showing that node2vec leads to an accurate and diversified set of results, especially on the large scale real-world data. We then describe the implementation in an instant search scenario and discuss a set of additional challenges tied to the specific production environment. Finally, we report the results of a large scale A/B test involving millions of users and prove that node2vec query suggestions lead to an increase in online metrics such as coverage (+1.42% shown search results pages with suggestions) and engagement (+1.21% clicks), with a specifically notable boost in the number of clicks on exploratory search queries (+9.37%).
更多查看译文
关键词
graph learning,query suggestions,exploratory search,spotify
AI 理解论文
溯源树
样例
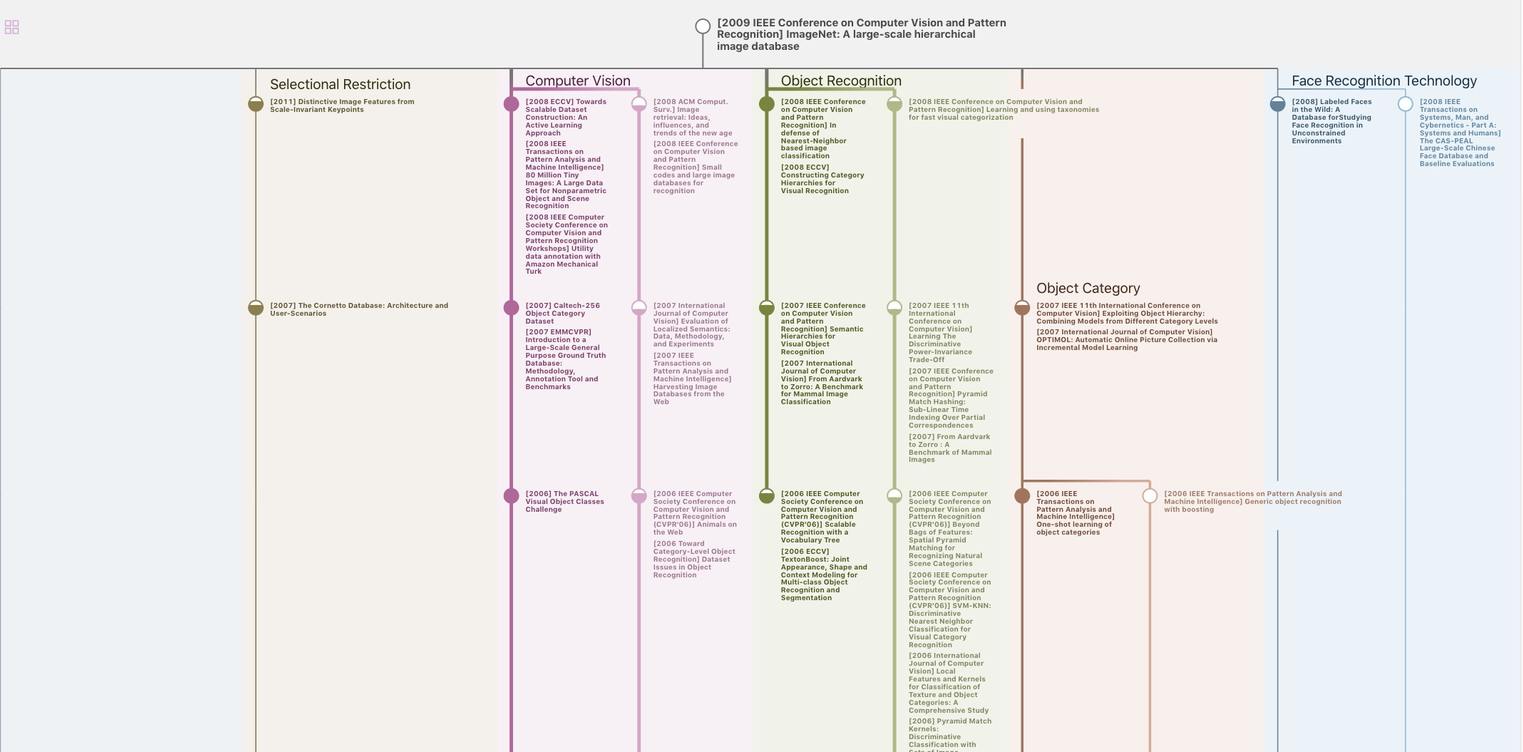
生成溯源树,研究论文发展脉络
Chat Paper
正在生成论文摘要