Causal Discovery in Temporal Domain from Interventional Data
PROCEEDINGS OF THE 32ND ACM INTERNATIONAL CONFERENCE ON INFORMATION AND KNOWLEDGE MANAGEMENT, CIKM 2023(2023)
摘要
Causal learning from observational data has garnered attention as controlled experiments can be costly. To enhance identifiability, incorporating intervention data has become a mainstream approach. However, these methods have yet to be explored in the context of time series data, despite their success in static data. To address this research gap, this paper presents a novel contribution. Firstly, a temporal interventional dataset with causal labels is introduced, derived from a data center IT room of a cloud service company. Secondly, this paper introduces TECDI, a novel approach for temporal causal discovery. TECDI leverages the smooth, algebraic characterization of acyclicity in causal graphs to efficiently uncover causal relationships. Experimental results on simulated and proposed real-world datasets validate the effectiveness of TECDI in accurately uncovering temporal causal relationships. The introduction of the temporal interventional dataset and the superior performance of TECDI contribute to advancing research in temporal causal discovery. Our datasets and codes have released at https://github.com/lpwpower/TECDI.
更多查看译文
关键词
Causal Discovery,Time Series,Intervention
AI 理解论文
溯源树
样例
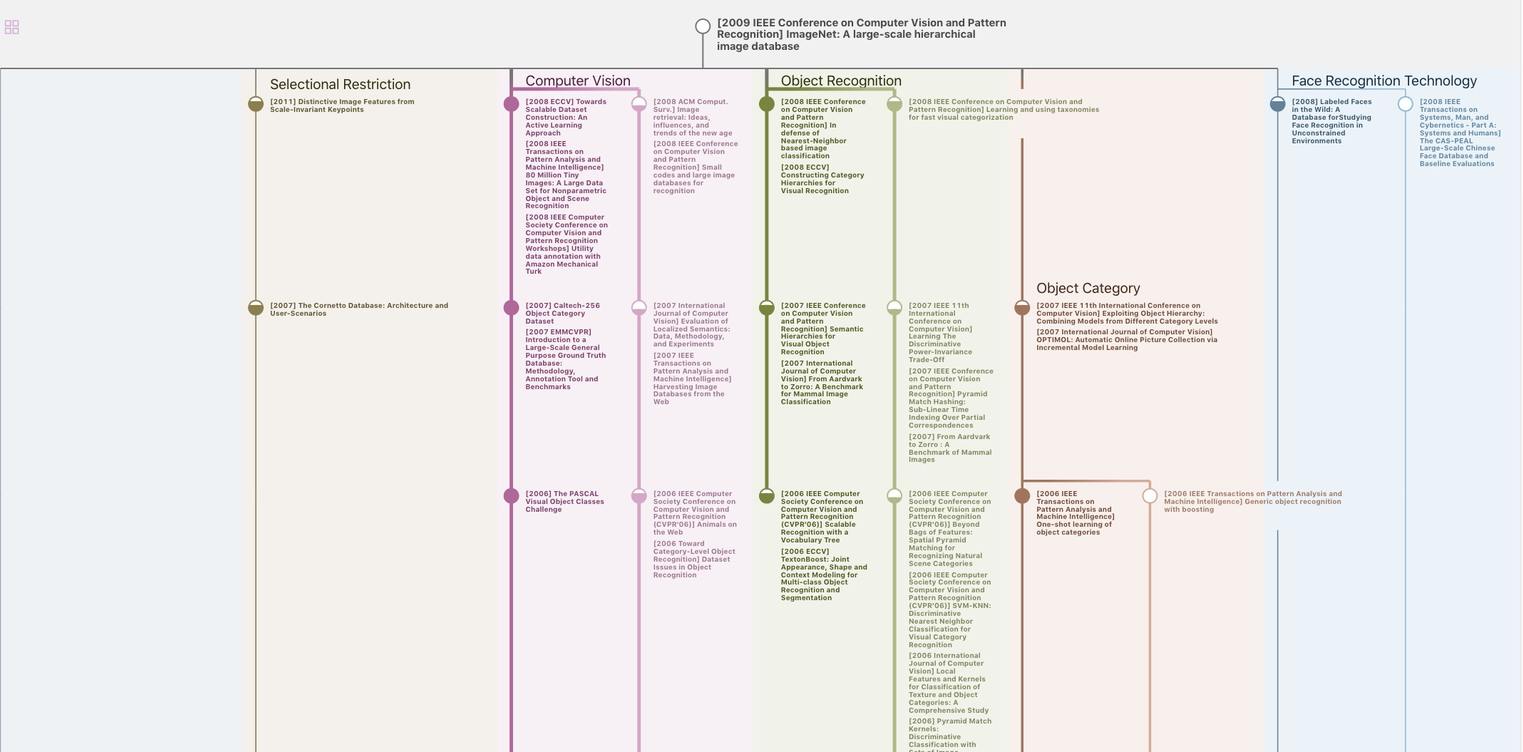
生成溯源树,研究论文发展脉络
Chat Paper
正在生成论文摘要