Boosting Meta-Learning Cold-Start Recommendation with Graph Neural Network
PROCEEDINGS OF THE 32ND ACM INTERNATIONAL CONFERENCE ON INFORMATION AND KNOWLEDGE MANAGEMENT, CIKM 2023(2023)
摘要
Meta-learning methods have shown to be effective in dealing with cold-start recommendation. However, most previous methods rely on an ideal assumption that there exists a similar data distribution between source and target tasks, which are unsuitable for the scenario that only extremely limited number of new user or item interactions are available. In this paper, we propose to boost meta-learning cold-start recommendation with graph neural network (MeGNN). First, it utilizes the global neighborhood translation learning to obtain consistent potential interactions for all new user and item nodes, which can refine their representations. Second, it employs the local neighborhood translation learning to predict specific potential interactions for each node, thus guaranteeing the personalized requirement. In experiments, we combine MeGNN with two representative meta-learning models MeLU and TaNP. Extensive results on two widely-used datasets show the superiority of MeGNN in four different scenarios.
更多查看译文
关键词
Recommendation,Meta-Learning,Graph Neural Network
AI 理解论文
溯源树
样例
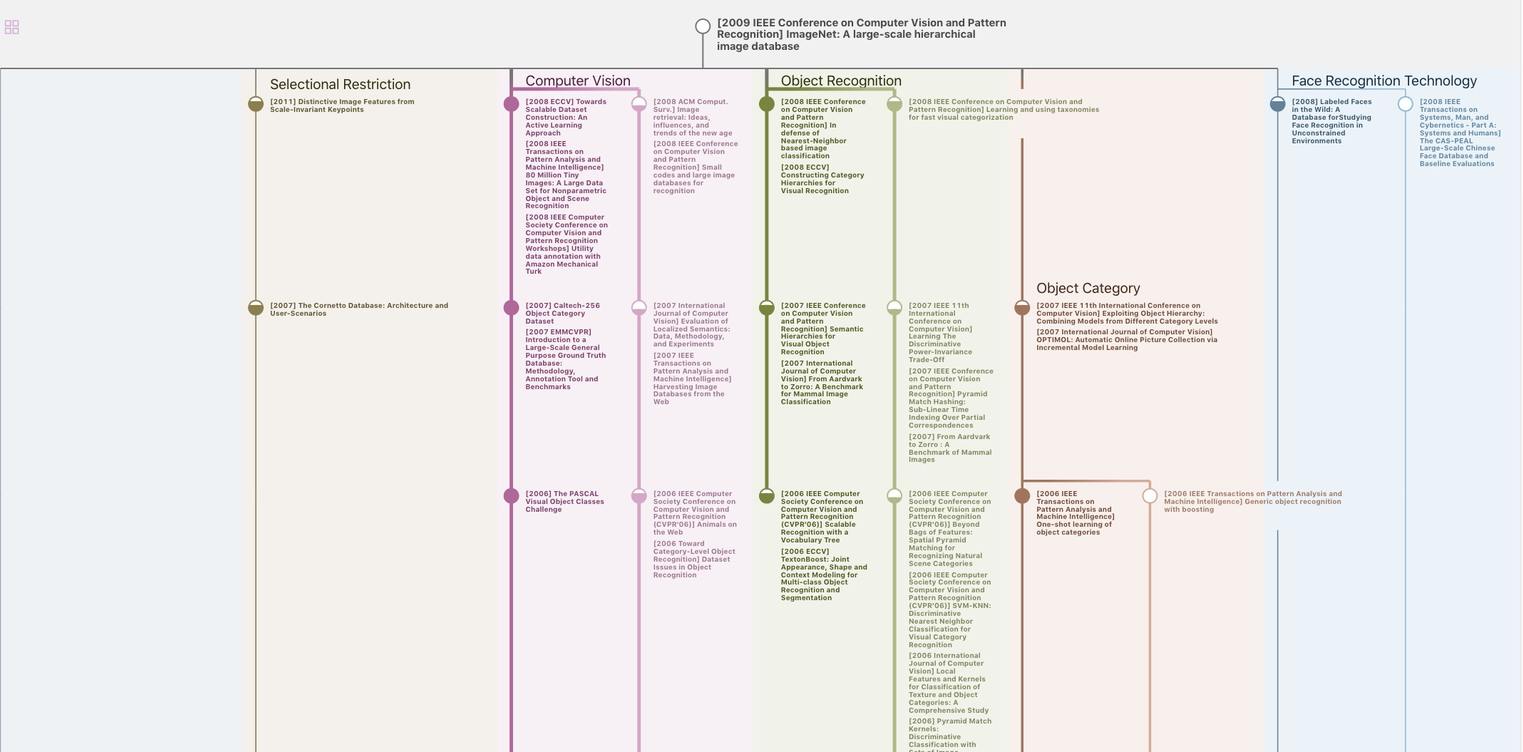
生成溯源树,研究论文发展脉络
Chat Paper
正在生成论文摘要