Reconciling Training and Evaluation Objectives in Location Agnostic Surrogate Explainers
PROCEEDINGS OF THE 32ND ACM INTERNATIONAL CONFERENCE ON INFORMATION AND KNOWLEDGE MANAGEMENT, CIKM 2023(2023)
摘要
Transparency in AI models is crucial to designing, auditing, and deploying AI systems. However, 'black box' models are still used in practice for their predictive power despite their lack of transparency. This has led to a demand for post-hoc, model-agnostic surrogate explainers which provide explanations for decisions of any model by approximating its behaviour close to a query point with a surrogate model. However, it is often overlooked how the location of the query point in the decision surface of the black box model affects the faithfulness of the surrogate explainer. Here, we show that when using standard techniques, there is a decrease in agreement between the black box and the surrogate model for query points towards the edge of the test dataset and when moving away from the decision boundary. This originates from a mismatch between the data distributions used to train and evaluate surrogate explainers. We address this by leveraging knowledge about the test data distribution captured in the class labels of the black box model. By addressing this and encouraging users to take care in understanding the alignment of training and evaluation objectives, we empower them to construct more faithful surrogate explainers.
更多查看译文
关键词
Explainabilty,Local Surrogate Explainers,Class Balancing
AI 理解论文
溯源树
样例
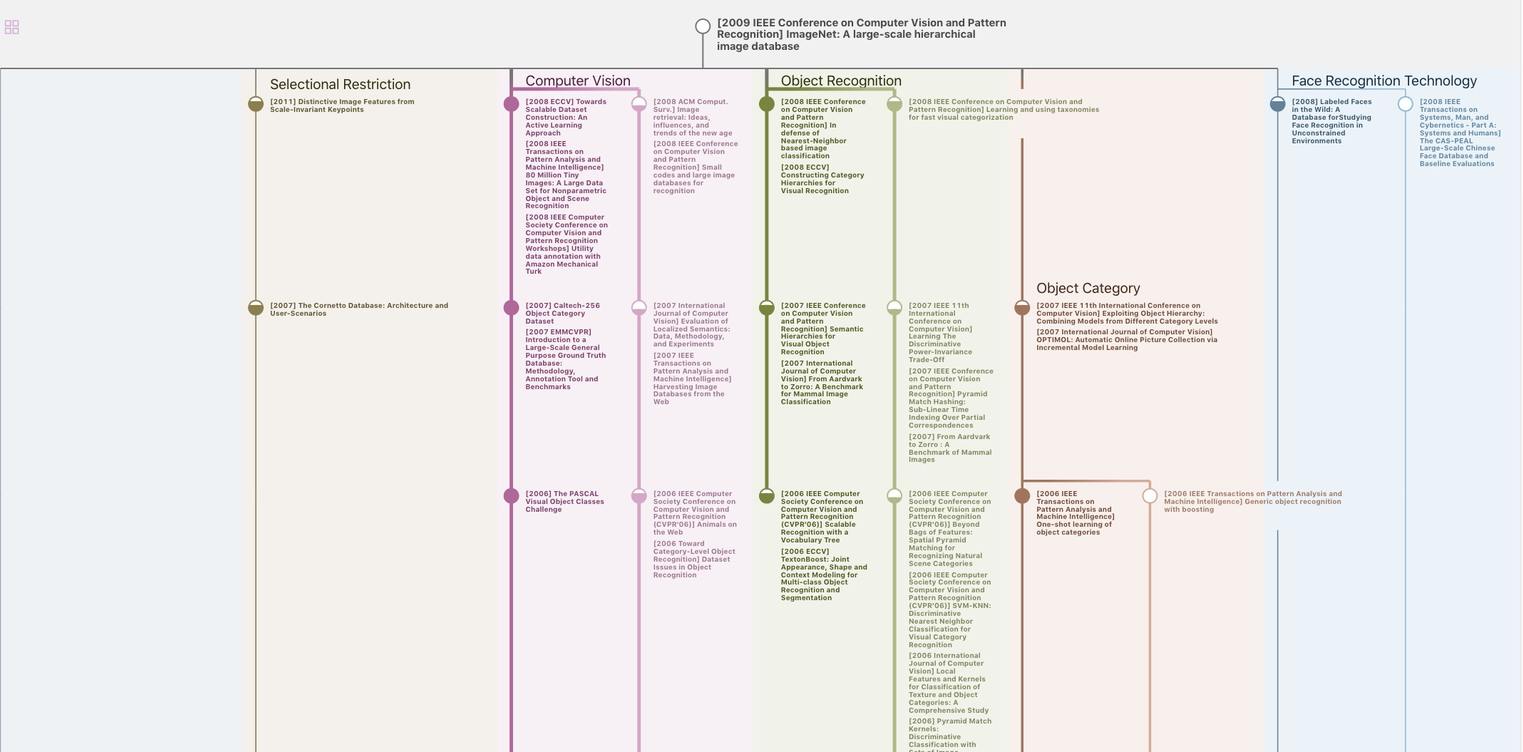
生成溯源树,研究论文发展脉络
Chat Paper
正在生成论文摘要