Urban-scale POI Updating with Crowd Intelligence
PROCEEDINGS OF THE 32ND ACM INTERNATIONAL CONFERENCE ON INFORMATION AND KNOWLEDGE MANAGEMENT, CIKM 2023(2023)
摘要
Points of Interest (POIs), such as entertainment, dining, and living, are crucial for urban planning and location-based services. However, the high dynamics and expensive updating costs of POIs pose a key roadblock for their urban applications. This is especially true for developing countries, where active economic activities lead to frequent POI updates (e.g., merchants closing down and new ones opening). Therefore, POI updating, i.e., detecting new POIs and different names of the same POIs (alias) to update the POI database, has become an urgent but challenging problem to address. In this paper, we attempt to answer the research question of how to detect and update large-scale POIs via a low-cost approach. To do so, we propose a novel framework called UrbanPOI, which formulates the POI updating problem as a tagging and detection problem based on multi-modal logistics delivery data. UrbanPOI consists of two key modules: (i) a hierarchical POI candidate generation module based on the POINet model that detects POIs from shipping addresses; and (ii) a new POI detection module based on the Siamese Attention Network that models multi-modal data and crowd intelligence. We evaluate our framework on real-world logistics delivery datasets from two Chinese cities. Extensive results show that our model outperforms state-of-the-art models in Beijing City by 26.2% in precision and 10.7% in F1-score, respectively.
更多查看译文
关键词
POI updating,last-mile delivery,smart city
AI 理解论文
溯源树
样例
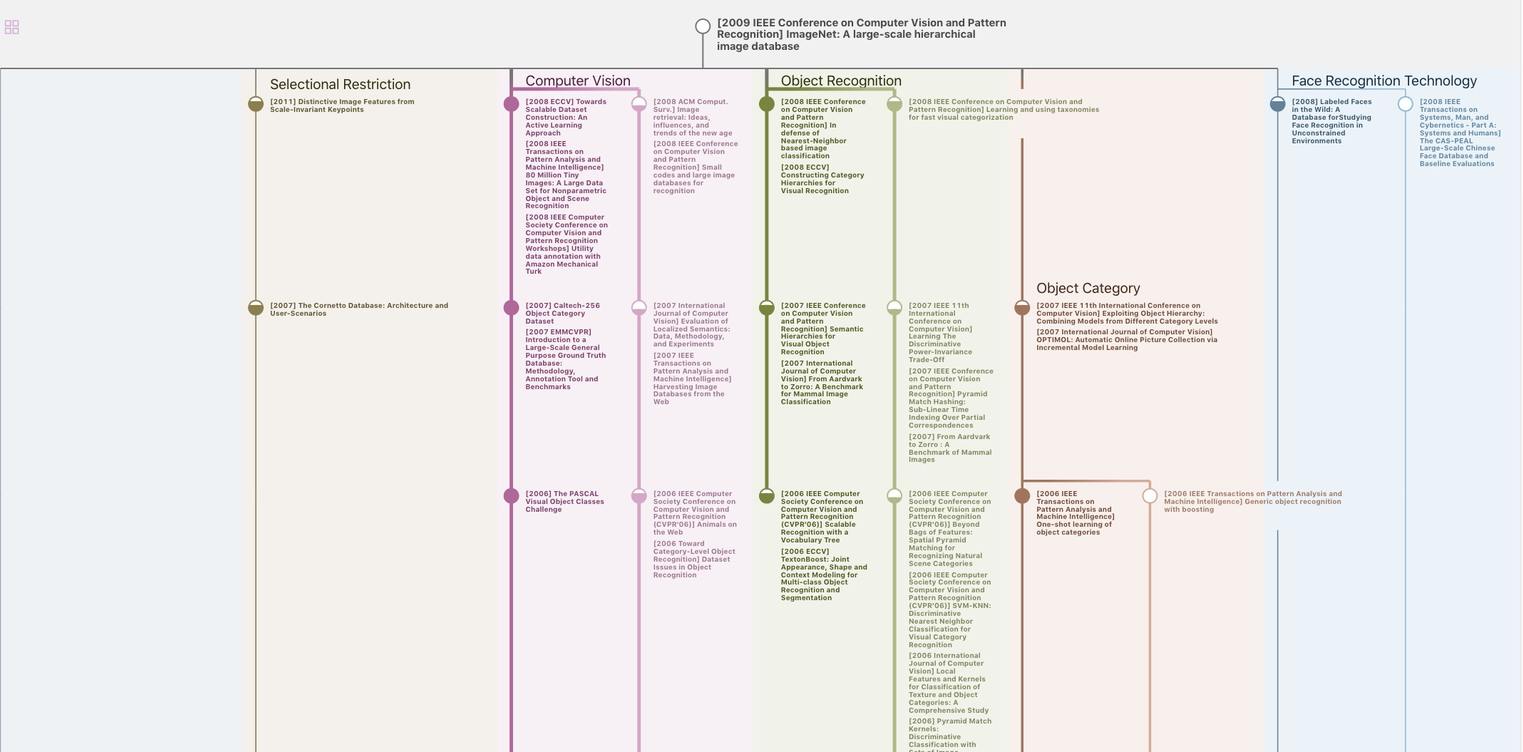
生成溯源树,研究论文发展脉络
Chat Paper
正在生成论文摘要