NP-SSL: A Modular and Extensible Self-supervised Learning Library with Neural Processes
PROCEEDINGS OF THE 32ND ACM INTERNATIONAL CONFERENCE ON INFORMATION AND KNOWLEDGE MANAGEMENT, CIKM 2023(2023)
摘要
Neural Processes (NPs) are a family of supervised density estimators devoted to probabilistic function approximation with meta-learning. Despite extensive research on the subject, the absence of a unified framework for NPs leads to varied architectural solutions across diverse studies. This non-consensus poses challenges to reproducing and benchmarking different NPs. Moreover, existing codebases mainly prioritize generative density estimation, yet rarely consider expanding the capability of NPs to self-supervised representation learning, which however has gained growing importance in data mining applications. To this end, we present NP-SSL, a modular and configurable framework with built-in support, requiring minimal effort to 1) implement classical NPs architectures; 2) customize specific components; 3) integrate hybrid training scheme (e.g., contrastive); and 4) extend NPs to act as a self-supervised learning toolkit, producing latent representations of data, and facilitating diverse downstream predictive tasks. To illustrate, we discuss a case study that applies NP-SSL to model time-series data. We interpret that NP-SSL can handle different predictive tasks such as imputation and forecasting, by a simple switch in data samplings, without significant change to the underlying structure. We hope this study can reduce the workload of future research on leveraging NPs to tackle more a broader range of real-world data mining applications. Code and documentation are at https://github.com/zyecs/NP-SSL.
更多查看译文
关键词
Neural Processes,probabilistic meta-learning,self-supervised learning
AI 理解论文
溯源树
样例
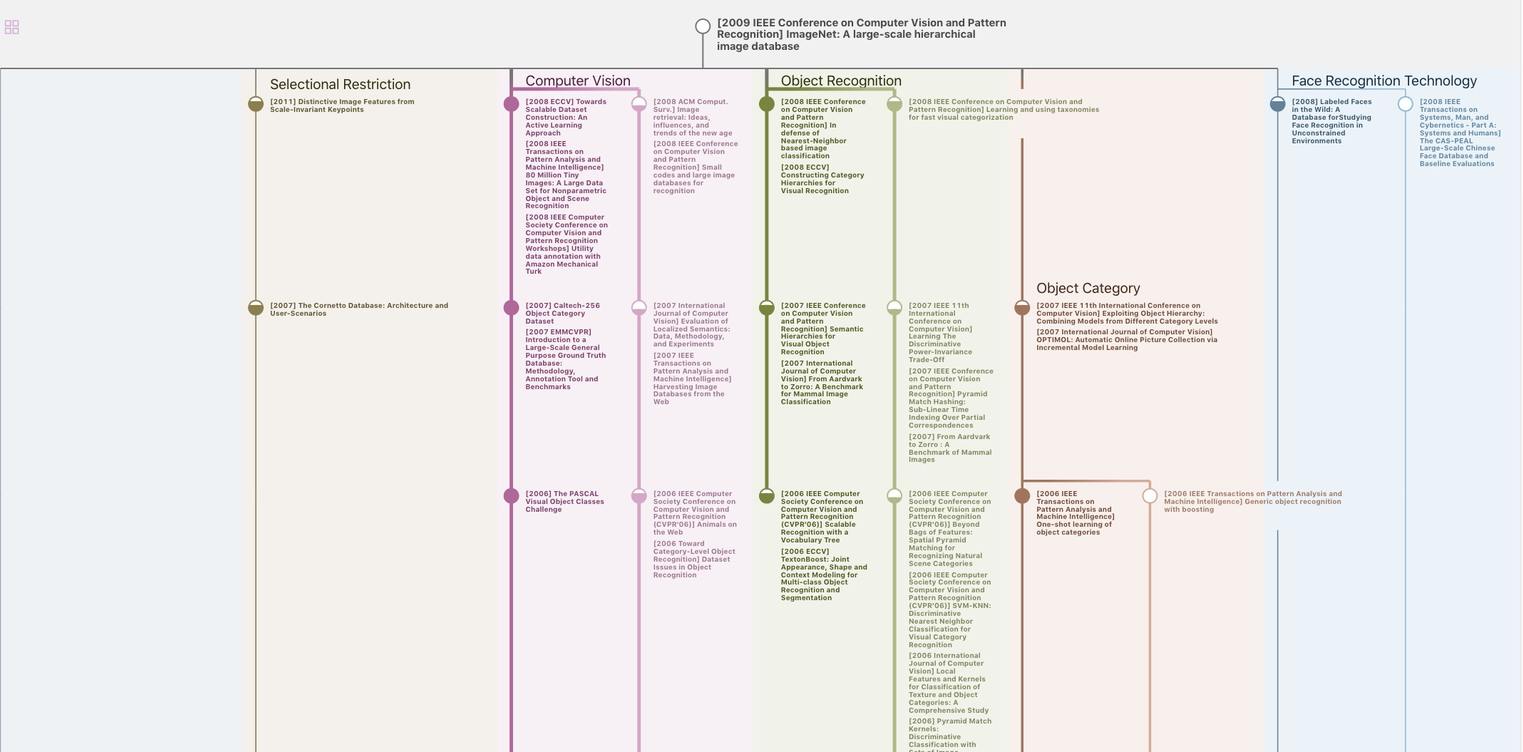
生成溯源树,研究论文发展脉络
Chat Paper
正在生成论文摘要