NumJoin: Discovering Numeric Joinable Tables with Semantically Related Columns
PROCEEDINGS OF THE 32ND ACM INTERNATIONAL CONFERENCE ON INFORMATION AND KNOWLEDGE MANAGEMENT, CIKM 2023(2023)
摘要
Join discovery is a crucial part of exploration on data lakes. It often involves finding joinable tables that are semantically relevant. However, data lakes often contain numeric tables with unreliable column headers, and ID columns whose text names have been lost. Finding semantically relevant joins over numeric tables is a challenge. State-of-the-art describes join discovery using semantic similarity, but do not consider purely numeric tables. In this paper, we describe a system, NumJoin that includes two novel approaches for discovering joinable tables in a data lake: one that maps tables to knowledge graphs, and another that leverages numeric types and distributions. We demonstrate the effectiveness of NumJoin on a large data lake, including transportation data and finance data.
更多查看译文
关键词
semantic join discovery,numeric data integration,tabular data
AI 理解论文
溯源树
样例
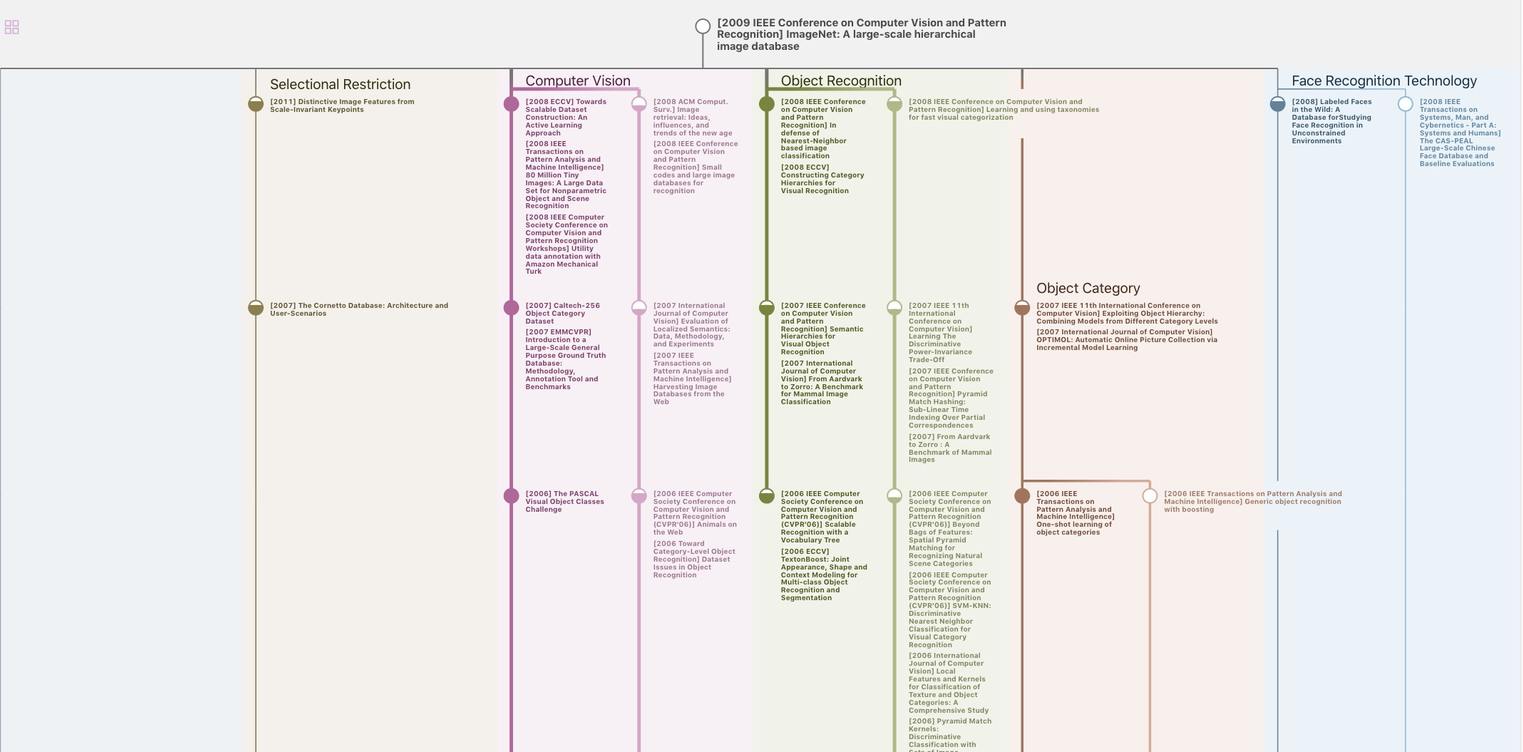
生成溯源树,研究论文发展脉络
Chat Paper
正在生成论文摘要