Quantitative Decomposition of Prediction Errors Revealing Multi-Cause Impacts: An Insightful Framework for MLOps
PROCEEDINGS OF THE 32ND ACM INTERNATIONAL CONFERENCE ON INFORMATION AND KNOWLEDGE MANAGEMENT, CIKM 2023(2023)
摘要
As machine learning applications expand in various industries, MLOps, which enables continuous model operation and improvement, becomes increasingly significant. Identifying causes of prediction errors, such as low model performance or anomalous samples, and implementing appropriate countermeasures are essential for effective MLOps. Furthermore, quantitatively evaluating each cause's impact is necessary to determine the effectiveness of countermeasures. In this study, we propose a method to quantitatively decompose a single sample's prediction error into contributions from multiple causes. Our method involves four steps: calculating the prediction error, computing metrics related to error causes, using a regression model to learn the relationship between the error and metrics, and applying SHAP to interpret the model's predictions and calculate the contribution of each cause to the prediction error. Numerical experiments with open data show that our method offers valuable insights for model improvement, confirming the effectiveness of our approach.
更多查看译文
关键词
MLOps,Machine Learning,Error Analysis,Interpretability
AI 理解论文
溯源树
样例
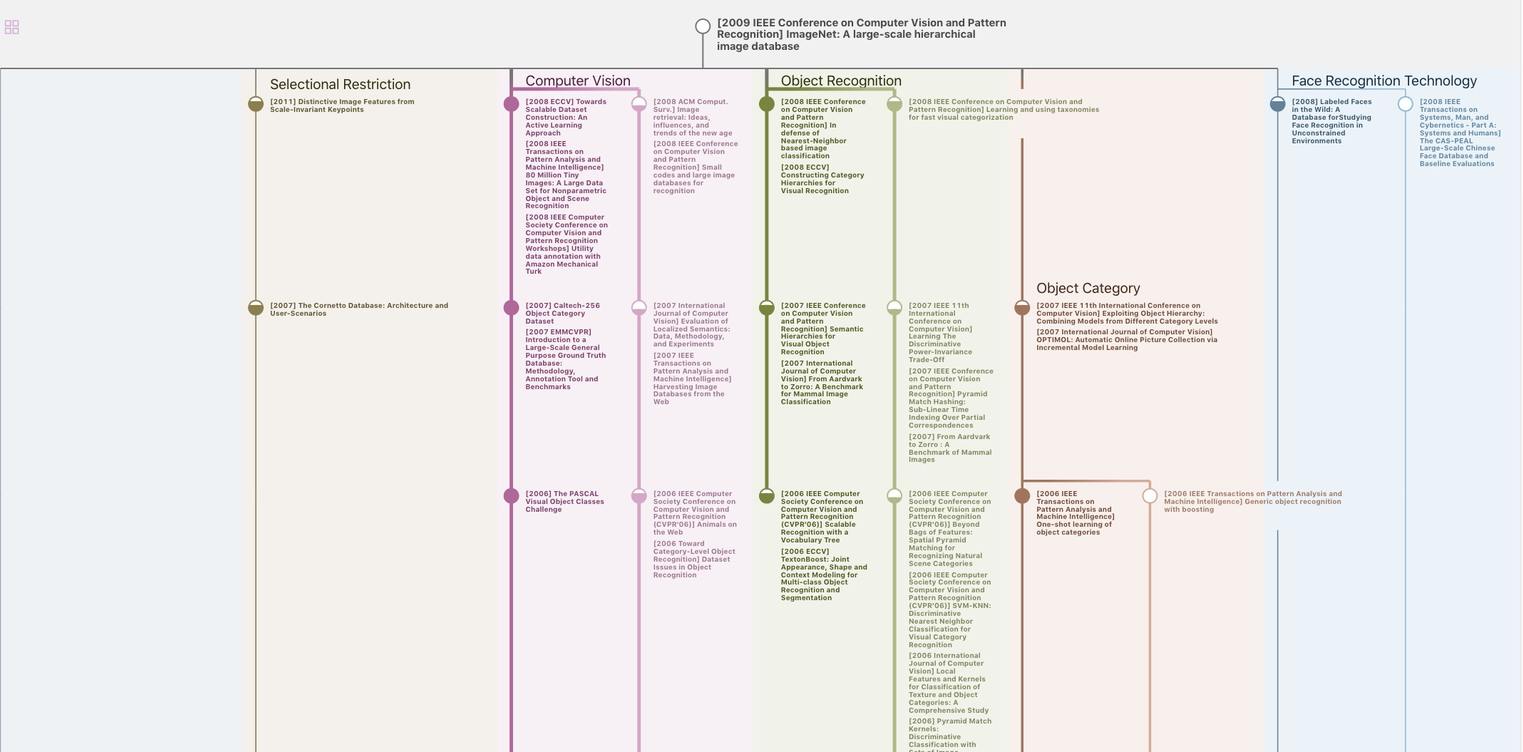
生成溯源树,研究论文发展脉络
Chat Paper
正在生成论文摘要