Improving Diversity in Unsupervised Keyphrase Extraction with Determinantal Point Process
PROCEEDINGS OF THE 32ND ACM INTERNATIONAL CONFERENCE ON INFORMATION AND KNOWLEDGE MANAGEMENT, CIKM 2023(2023)
摘要
Keyphrase extraction aims to provide readers with high-level information about the central ideas or important topics described in a given source text. Recent advances in embedding-based models have made remarkable progress on unsupervised keyphrase extraction, demonstrated through improved quality metrics such as F1-score. However, the diversity in the keyphrase extraction task needs to be addressed. In this paper, we focus on diverse keyphrase extraction, which entails extracting keyphrases that cover different central information or essential topics in the document. To achieve this goal, we propose a re-ranking-based approach that employs determinantal point processes utilizing BERT as kernels, which we call DiversityRank. Specifically, DiversityRank jointly considers phrase-document relevance and cross-phrase similarities to select candidate keyphrases that are document-relevant and diverse. Results demonstrate that our re-ranking strategy outperforms the state-of-the-art unsupervised keyphrase extraction baselines on three benchmark datasets.
更多查看译文
关键词
information extraction,keyphrase extraction,unsupervised learning,determinantal point processes
AI 理解论文
溯源树
样例
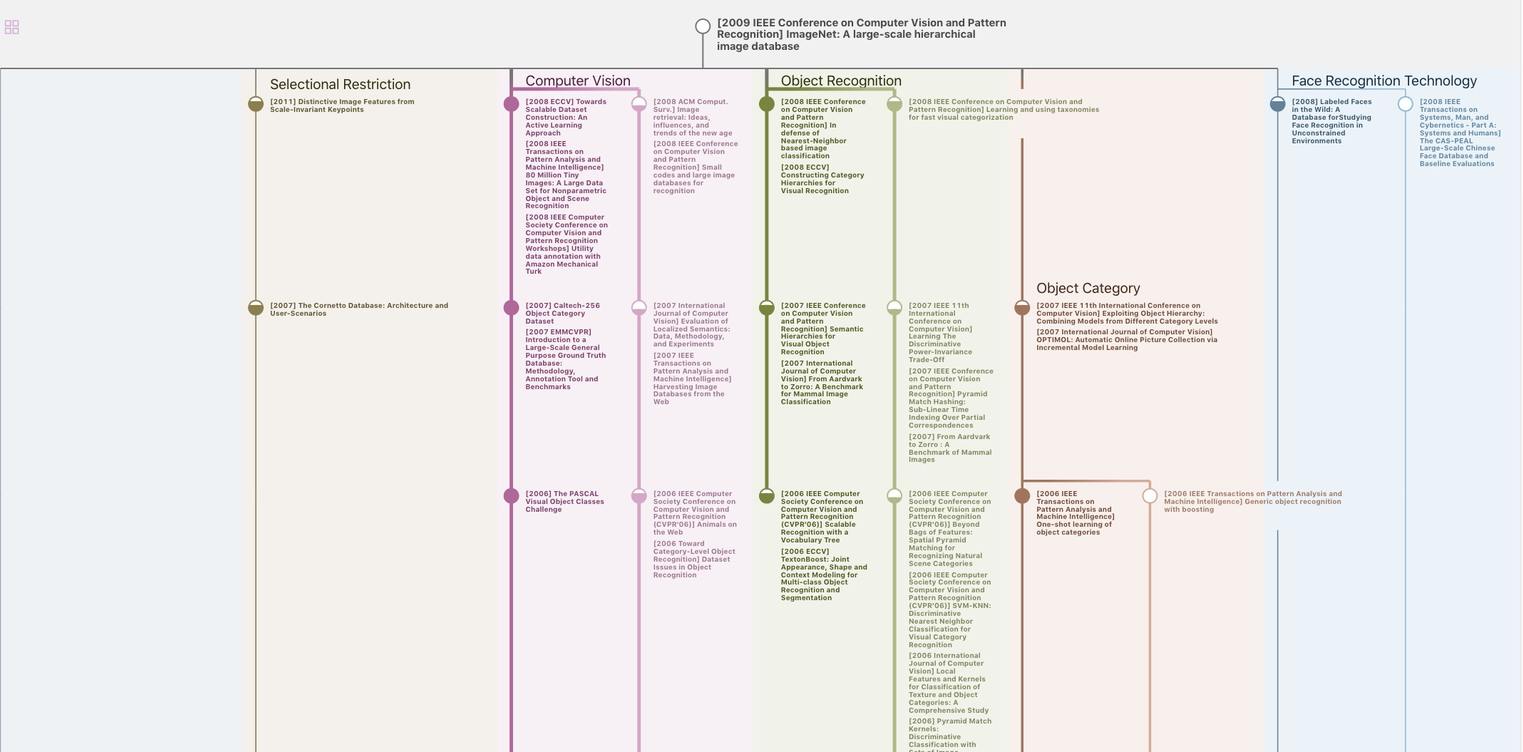
生成溯源树,研究论文发展脉络
Chat Paper
正在生成论文摘要