Sequential Text-based Knowledge Update with Self-Supervised Learning for Generative Language Models
PROCEEDINGS OF THE 32ND ACM INTERNATIONAL CONFERENCE ON INFORMATION AND KNOWLEDGE MANAGEMENT, CIKM 2023(2023)
摘要
This work proposes a new natural language processing (NLP) task to tackle the issue of multi-round, sequential text-based knowledge update. The study introduces a hybrid learning architecture and a novel self-supervised training strategy to enable generative language models to consolidate knowledge in the same way as humans. A dataset was also created for evaluation and results showed the effectiveness of our methodology. Experimental results confirm the superiority of the proposed approach over existing models and large language models (LLMs). The proposed task and model framework have the potential to significantly improve the automation of knowledge organization, making text-based knowledge an increasingly crucial resource for powerful LLMs to perform various tasks for humans.
更多查看译文
关键词
natural language generation,temporal knowledge modeling,update summarization,self-supervision
AI 理解论文
溯源树
样例
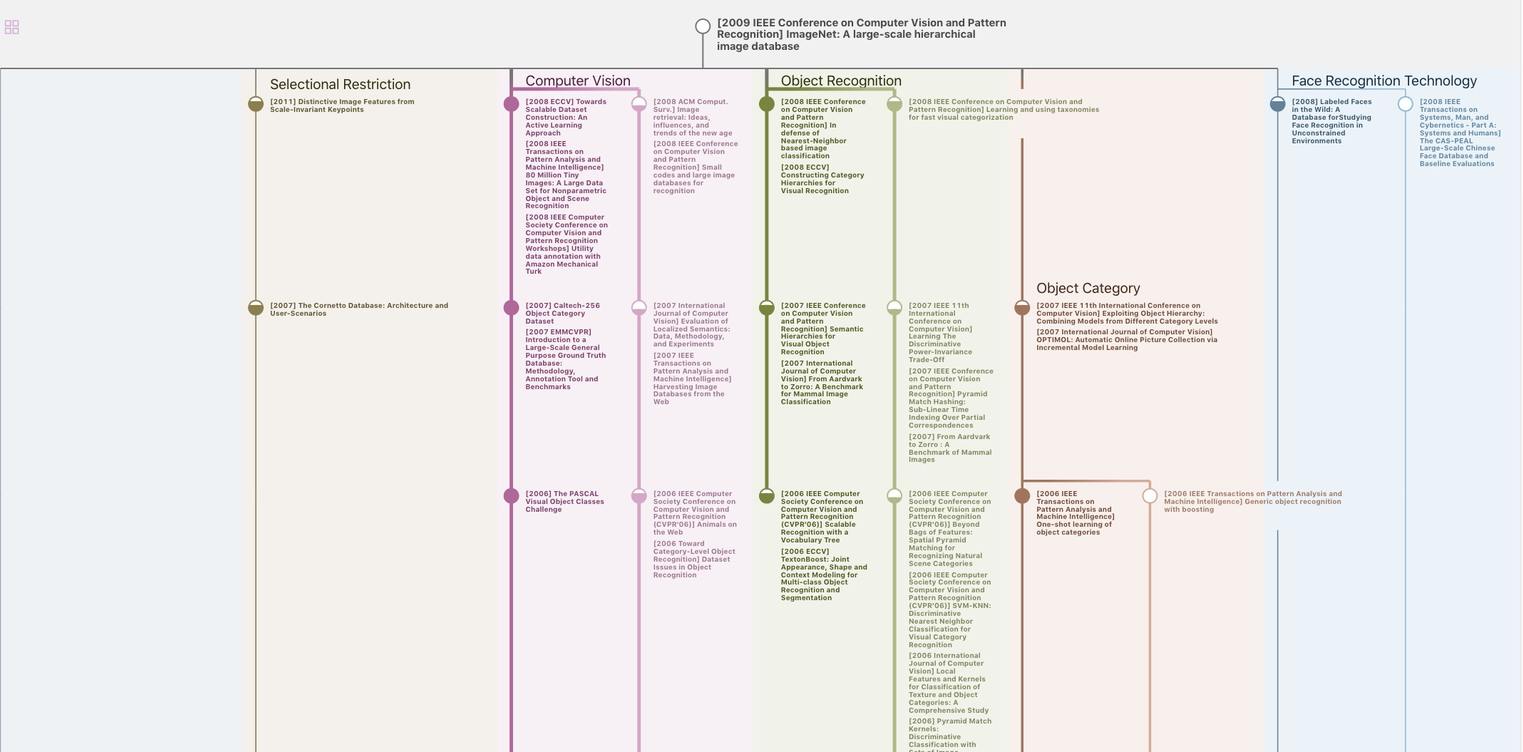
生成溯源树,研究论文发展脉络
Chat Paper
正在生成论文摘要