AutoMRM: A Model Retrieval Method Based on Multimodal Query and Meta-learning
PROCEEDINGS OF THE 32ND ACM INTERNATIONAL CONFERENCE ON INFORMATION AND KNOWLEDGE MANAGEMENT, CIKM 2023(2023)
摘要
With more and more Deep Neural Network (DNN) models are publicly available on model sharing platforms (e.g., HuggingFace), model reuse has become a promising way in practice to improve the efficiency of DNN model construction by avoiding the costs of model training. To that end, a pivotal step for model reuse is model retrieval, which facilitates discovering suitable models from a model hub that match the requirements of users. However, the existing model retrieval methods have inadequate performance and efficiency, since they focus on matching user requirements with the model names, and thus cannot work well for high-dimensional data such as images. In this paper, we propose a user-task-centric multimodal model retrieval method named AutoMRM. AutoMRM can retrieve DNN models suitable for the user's task according to both the dataset and description of the task. Moreover, AutoMRM utilizes meta-learning to retrieve models for previously unseen task queries. Specifically, given a task, AutoMRM extracts the latent meta-features from the dataset and description for training meta-learners offline and obtaining the representation of user task queries online. Experimental results demonstrate that AutoMRM outperforms existing model retrieval methods including the state-of-the-art method in both effectiveness and efficiency.
更多查看译文
关键词
Model retrieval,Machine learning,Meta-learning,Information extraction
AI 理解论文
溯源树
样例
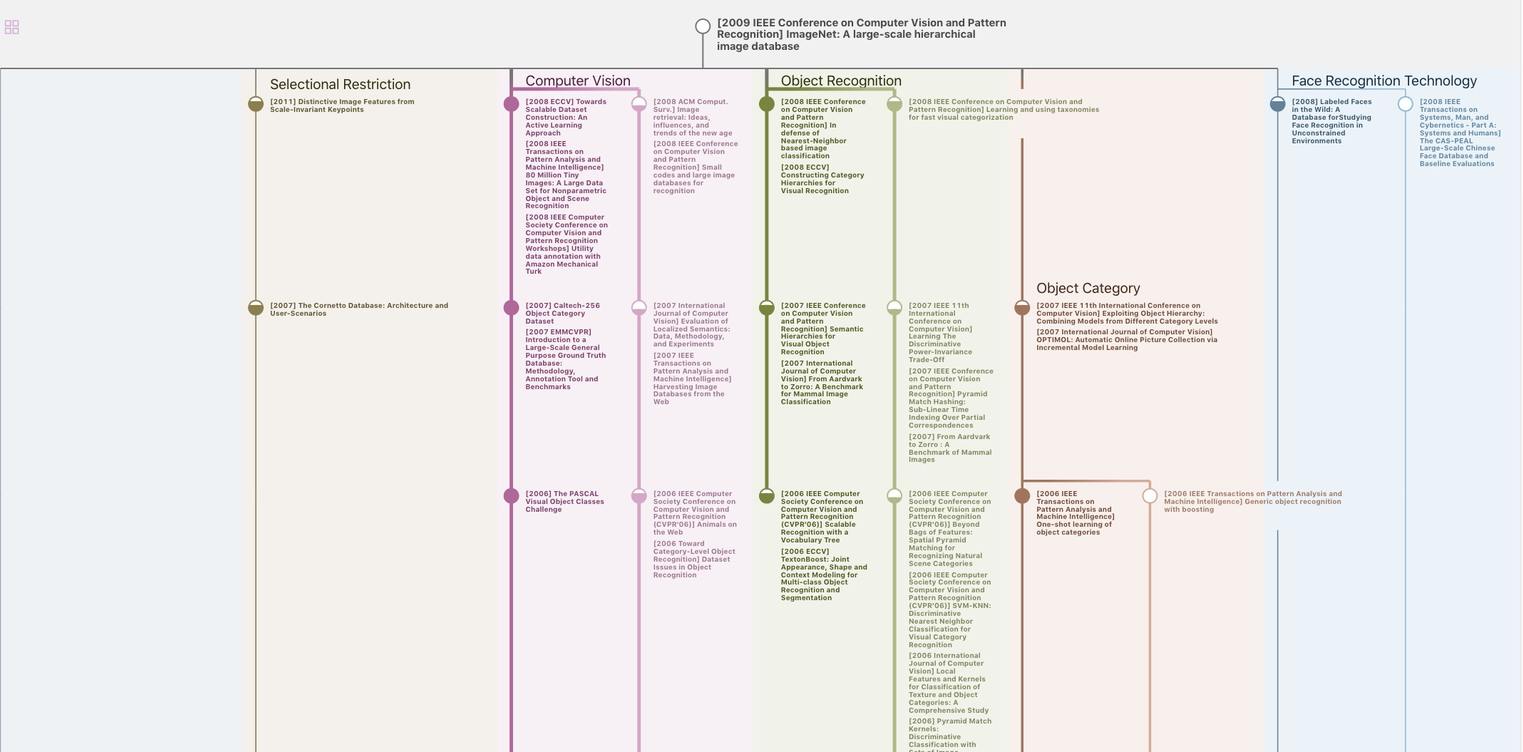
生成溯源树,研究论文发展脉络
Chat Paper
正在生成论文摘要