Towards Automatic ICD Coding via Knowledge Enhanced Multi-Task Learning
PROCEEDINGS OF THE 32ND ACM INTERNATIONAL CONFERENCE ON INFORMATION AND KNOWLEDGE MANAGEMENT, CIKM 2023(2023)
摘要
The aim of ICD coding is to assign International Classification of Diseases (ICD) codes to unstructured clinical notes or discharge summaries. Numerous methods have been proposed for automatic ICD coding in an effort to reduce human labor and errors. However, existing works disregard the data imbalance problem of clinical notes. In addition, the noisy clinical note issue has not been thoroughly investigated. To address such issues, we propose a knowledge enhanced Graph Attention Network (GAT) under multi-task learning setting. Specifically, multi-level information transitions and interactions have been implemented. On the one hand, a large heterogeneous text graph is constructed to capture both intra- and inter-note correlations between various semantic concepts, thereby alleviating the data imbalance issue. On the other hand, two auxiliary healthcare tasks have been proposed to facilitate the sharing of information across tasks. Moreover, to tackle the issue of noisy clinical notes, we propose to utilize the rich structured knowledge facts and information provided by medical domain knowledge, thereby encouraging the model to focus on the clinical notes' noteworthy portion and valuable information. The experimental results on the widely-used medical dataset, MIMIC-III, demonstrate the advantages of our proposed framework.
更多查看译文
关键词
ICD coding,multi-task learning,knowledge graph
AI 理解论文
溯源树
样例
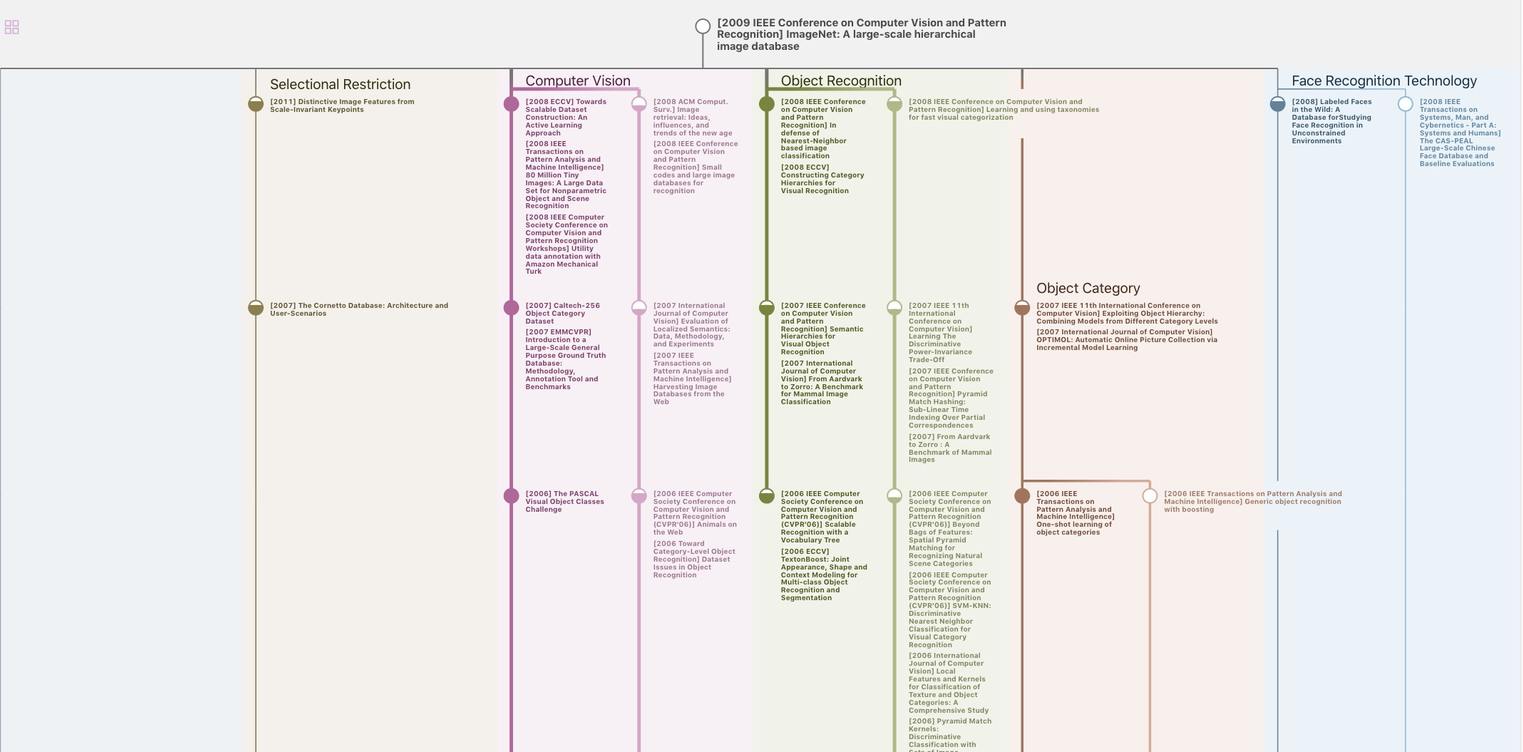
生成溯源树,研究论文发展脉络
Chat Paper
正在生成论文摘要